Gesture Recognition using FastDTW and Deep Learning Methods in the MSRC-12 and the NTU RGB+D Databases
Keywords:
Deep learning, convolutional neural networks, long short-term memory, gated recurrent unit, gesture recognition, FastDTWAbstract
This work explores the use of three deep learning methods for gesture recognition: Convolutional Neural Networks (CNN), Long Short-Term Memory (LSTM), and Gated Recurrent Unit (GRU) using Fast Dynamic Time Warping (FastDTW). The gestures were captured by Kinect sensors, two skeleton-based databases are used: Microsoft Research Cambridge-12 (MSRC-12) and NTU RGB+D. Also, the FastDTW technique was also employed to standardize the input size of the data. The MSRC-12 database achieved an accuracy rate of 82,36% in the test set with the CNN, the LSTM achieved an accuracy rate of 87,30% also in the test set, and in GRU the accuracy achieved in the test set was 89,34%. With the NTU RGB+D database, two evaluation methods were used: Cross-View and Cross-Subject. In the test set with Cross-View evaluation was obtained an accuracy rate of 63,53%, 55,14%, and 61,00%, with CNN, LSTM, and GRU respectively; and with the Cross-Subject evaluation method, it was achieved an accuracy rate of 66,19%, 64,43% and 60,17% in the test set on CNN, LSTM and GRU, respectively.
Downloads
References
S. Fothergill, H. M. Mentis, P. Kohli, and S. Nowozin, “Instructing
people for training gestural interactive systems,” Proceedings of the
SIGCHI Conference on Human Factors in Computing Systems, 2012.
A. Shahroudy, J. Liu, T.-T. Ng, and G. Wang, “Ntu rgb+d: A large
scale dataset for 3d human activity analysis,” in 2016 IEEE Conference
on Computer Vision and Pattern Recognition (CVPR), pp. 1010–1019,
S. Salvador and P. Chan, “Fastdtw: Toward accurate dynamic time
warping in linear time and space,” 2004.
K. Simonyan and A. Zisserman, “Very deep convolutional networks
for large-scale image recognition,” in 3rd International Conference on
Learning Representations, ICLR 2015, San Diego, CA, USA, May 7-9,
, Conference Track Proceedings (Y. Bengio and Y. LeCun, eds.),
C. Szegedy, Wei Liu, Yangqing Jia, P. Sermanet, S. Reed, D. Anguelov,
D. Erhan, V. Vanhoucke, and A. Rabinovich, “Going deeper with convolutions,”
in 2015 IEEE Conference on Computer Vision and Pattern
Recognition (CVPR), pp. 1–9, June 2015.
M. Pfitscher, D. Welfer, E. J. D. Nascimento, M. A. D. S. L. Cuadros,
and D. F. T. Gamarra, “Article users activity gesture recognition on
kinect sensor using convolutional neural networks and fastdtw for
controlling movements of a mobile robot,” Inteligencia Artif., vol. 22,
no. 63, pp. 121–134, 2019.
J. S. Peixoto, M. Pfitscher, M. A. de Souza Leite Cuadros, D. Welfer,
and D. F. T. Gamarra, “Comparison of different processing methods of
joint coordinates features for gesture recognition with a cnn in the msrc-
database,” in Intelligent Systems Design and Applications, (Cham),
pp. 590–599, Springer International Publishing, 2021.
F. Meng, H. Liu, Y. Liang, M. Liu, and W. Liu, “Hierarchical dropped
convolutional neural network for speed insensitive human action recognition,”
in 2018 IEEE International Conference on Multimedia and Expo
(ICME), pp. 1–6, 2018.
J. Tu, M. Liu, and H. Liu, “Skeleton-based human action recognition
using spatial temporal 3d convolutional neural networks,” in 2018 IEEE
International Conference on Multimedia and Expo (ICME), pp. 1–6,
K. Lai and S. N. Yanushkevich, “Cnn+rnn depth and skeleton based dynamic
hand gesture recognition,” in 2018 24th International Conference
on Pattern Recognition (ICPR), pp. 3451–3456, 2018.
D.-W. Lee, K. Jun, S. Lee, J.-K. Ko, and M. S. Kim, “Abnormal gait
recognition using 3d joint information of multiple kinects system and
rnn-lstm,” in 2019 41st Annual International Conference of the IEEE
Engineering in Medicine and Biology Society (EMBC), pp. 542–545,
P. Molchanov, X. Yang, S. Gupta, K. Kim, S. Tyree, and J. Kautz,
“Online detection and classification of dynamic hand gestures with
recurrent 3d convolutional neural networks,” in 2016 IEEE Conference
on Computer Vision and Pattern Recognition (CVPR), pp. 4207–4215,
Y.-F. Song, Z. Zhang, and L. Wang, “Richly activated graph convolutional
network for action recognition with incomplete skeletons,” in 2019
IEEE International Conference on Image Processing (ICIP), pp. 1–5,
M.-H. Ha and O. T.-C. Chen, “Deep neural networks using capsule
networks and skeleton-based attentions for action recognition,” IEEE
Access, vol. 9, pp. 6164–6178, 2021.
X. Hao, J. Li, Y. Guo, T. Jiang, and M. Yu, “Hypergraph neural network
for skeleton-based action recognition,” IEEE Transactions on Image
Processing, vol. 30, pp. 2263–2275, 2021.
A. Krizhevsky, I. Sutskever, and G. E. Hinton, “Imagenet classification
with deep convolutional neural networks,” in Advances in Neural Information
Processing Systems (F. Pereira, C. J. C. Burges, L. Bottou, and
K. Q. Weinberger, eds.), vol. 25, Curran Associates, Inc., 2012.
D. Reis, D. Welfer, M. Cuadros, and D. F. Gamarra, “Mobile robot
navigation using an object recognition software with rgbd images and
the yolo algorithm,” Applied Artificial Intelligence, vol. 33, pp. 1–16,
2019.
J. C. Jesus, J. A. Bottega, M. A. S. L. Cuadros, and D. F. T. Gamarra,
“Deep deterministic policy gradient for navigation of mobile robots
in simulated environments,” in 2019 19th International Conference on
Advanced Robotics (ICAR), pp. 362–367, 2019.
V. Phung and E. Rhee, “A deep learning approach for classification of
cloud image patches on small datasets,” Journal of Information and
Communication Convergence Engineering, vol. 16, pp. 173–178, 01
S. Hochreiter and J. Schmidhuber, “Long short-term memory,” Neural
Computation, vol. 9, no. 8, pp. 1735–1780, 1997.
X. Yuan, L. Li, and Y. Wang, “Nonlinear dynamic soft sensor modeling
with supervised long short-term memory network,” IEEE Transactions
on Industrial Informatics, vol. PP, pp. 1–1, 02 2019.
K. Cho, B. van Merriënboer, C. Gulcehre, F. Bougares, H. Schwenk, and
Y. Bengio, “Learning phrase representations using rnn encoder-decoder
for statistical machine translation,” 06 2014.
H. Zhao, Z. Chen, H. Jiang,W. Jing, L. Sun, and M. Feng, “Evaluation of
three deep learning models for early crop classification using sentinel-1a
imagery time series-a case study in zhanjiang, china,” Remote Sensing,
vol. 11, p. 2673, 11 2019.
S. Fothergill, H. Mentis, P. Kohli, and S. Nowozin, “Instructing people
for training gestural interactive systems,” Conference on Human Factors
in Computing Systems - Proceedings, 05 2012
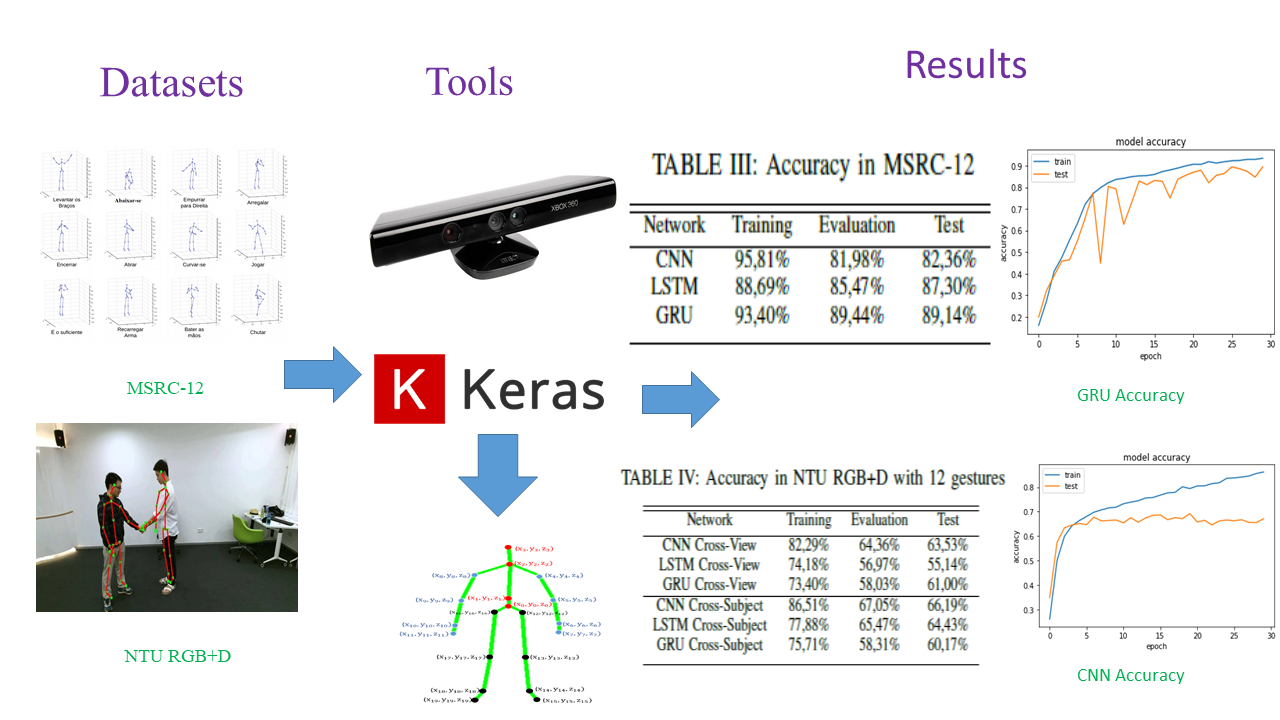