A Optimizing Solar Irradiance Prediction: Feature Selection for All-Sky Image Processing Using a Hybrid Prediction Method
Feature Selection for All-Sky Image Processing Using a Hybrid Prediction Method
Keywords:
all-sky image, solar irradiance prediction, artificial neural network, Hybrid Prediction Method, photovoltaic energy predictionAbstract
The forecasting of solar irradiance is crucial for photovoltaic solar energy generation, as production is subject to intermittency due to climatic conditions, such as cloud cover, wind and, temperature. Based on the Hybrid Prediction Method (HPM), this study investigated the influence of a set of all-sky image processing features on the HPM’s Artificial Neural Network prediction accuracy. Using correlation-based attribute selection, three predictive models with different input feature sets were evaluated. The results show that, when considering all horizons together and paired, the Medium set of 6 features achieves prediction accuracy statistically similar to the Complete set with 9 features, reducing the computational time (14.4%) and model input dimensionality (33.3%). However, when comparing individual horizons, the Complete set outperforms the Medium set at 5- and 15-minute horizon, while maintain similar accuracy at the 1-minute horizon. The Reduced set, with three features, consistently underperformed. This study provides news insights into the optimization of solar irradiance forecasting using HPM, contributing to advances in photovoltaic energy forecasting.
Downloads
References
ABSOLAR, “Energia Solar Fotovoltaica no Brasil - Infográfico ABSOLAR,” Associação Brasileira de Energia Solar Fotovoltaica. Accessed: Jul. 19, 2022. [Online]. Available: https://www.absolar.org.br/mercado/infografico
IEA, “Solar PV,” Paris, 2022. Accessed: Mar. 23, 2023. [Online]. Available: https://www.iea.org/reports/solar-pv
F. Antonanzas-Torres, R. Urraca, J. Polo, O. Perpiñán-Lamigueiro, and R. Escobar, “Clear sky solar irradiance models: A review of seventy models,” Renewable and Sustainable Energy Reviews, vol. 107, pp. 374–387, Jun. 2019, doi: 10.1016/j.rser.2019.02.032.
C. Ying, W. Wang, J. Yu, Q. Li, D. Yu, and J. Liu, “Deep learning for renewable energy forecasting: A taxonomy, and systematic literature review,” J Clean Prod, vol. 384, p. 135414, Jan. 2023, doi: 10.1016/j.jclepro.2022.135414.
J. N. Maciel, J. J. G. Ledesma, and O. H. Ando Junior, “Forecasting Solar Power Output Generation: A Systematic Review with the Proknow-C,” IEEE Latin America Transactions, vol. 19, no. 4, pp. 612–624, Apr. 2021, doi: 10.1109/TLA.2021.9448544.
D. S. Kumar, G. M. Yagli, M. Kashyap, and D. Srinivasan, “Solar irradiance resource and forecasting: a comprehensive review,” IET Renewable Power Generation, vol. 14, no. 10, pp. 1641–1656, 2020, doi: 10.1049/iet-rpg.2019.1227.
H. Wang, Z. Lei, X. Zhang, B. Zhou, and J. Peng, “A review of deep learning for renewable energy forecasting,” Energy Convers Manag, vol. 198, p. 111799, Oct. 2019, doi: 10.1016/j.enconman.2019.111799.
E. D. Obando, S. X. Carvajal, and J. Pineda Agudelo, “Solar Radiation Prediction Using Machine Learning Techniques: A Review,” IEEE Latin America Transactions, vol. 17, no. 04, pp. 684–697, Apr. 2019, doi: 10.1109/TLA.2019.8891934.
P. Kumari and D. Toshniwal, “Deep learning models for solar irradiance forecasting: A comprehensive review,” J Clean Prod, vol. 318, no. May, p. 128566, Oct. 2021, doi: 10.1016/j.jclepro.2021.128566.
F. Wang et al., “A minutely solar irradiance forecasting method based on real-time sky image-irradiance mapping model,” Energy Convers Manag, vol. 220, p. 113075, Sep. 2020, doi: 10.1016/j.enconman.2020.113075.
S. Dev, F. M. Savoy, Y. H. Lee, and S. Winkler, “Estimating Solar Irradiance Using Sky Imagers,” Oct. 2019, [Online]. Available: http://arxiv.org/abs/1910.04981
C. W. Chow, B. Urquhart, M. Lave, A. Dominguez, J. Kleissl, and ..., “Intra-hour forecasting with a total sky imager at the UC San Diego solar energy testbed,” Solar Energy, 2011, [Online]. Available: https://www.sciencedirect.com/science/article/pii/S0038092X11002982
Z. Zhen et al., “Ultra‐short‐term irradiance forecasting model based on ground‐based cloud image and deep learning algorithm,” IET Renewable Power Generation, vol. 16, no. 12, pp. 2604–2616, Sep. 2022, doi: 10.1049/rpg2.12280.
H. Yang, L. Wang, C. Huang, and X. Luo, “3d-cnn-based sky image feature extraction for short-term global horizontal irradiance forecasting,” Water (Switzerland), vol. 13, no. 13, Jul. 2021, doi: 10.3390/w13131773.
R. A. Rajagukguk, R. Kamil, and H.-J. Lee, “A Deep Learning Model to Forecast Solar Irradiance Using a Sky Camera,” Applied Sciences, vol. 11, no. 11, p. 5049, May 2021, doi: 10.3390/app11115049.
H. Victor et al., “Construção de um Banco de Dados para a Predição do Potencial de Geração de Energia Solar Fotovoltaica,” in IX Encontro Anual de Iniciação Científica - EICTI, Foz do Iguaçu-PR, 2020, p. 2020.
V. M. Serrano Ardila, J. N. Maciel, J. J. G. Ledesma, and O. H. Ando Junior, “Fuzzy Time Series Methods Applied to (In)Direct Short-Term Photovoltaic Power Forecasting,” Energies (Basel), vol. 15, no. 3, p. 845, Jan. 2022, doi: 10.3390/en15030845.
V. H. Wentz, J. N. Maciel, J. J. Gimenez Ledesma, and O. H. Ando Junior, “Solar Irradiance Forecasting to Short-Term PV Power: Accuracy Comparison of ANN and LSTM Models,” Energies (Basel), vol. 15, no. 7, p. 2457, Mar. 2022, doi: 10.3390/en15072457.
J. N. Maciel, “Método Híbrido de Predição da Irradiância Solar Com Processamento de Imagens e Inteligência Artificial Aplicável a Geração de Energia Solar Fotovoltaica,” Tese de Doutorado, Universidade Federal da Integração Latino-Americana (UNILA), Foz do Iguaçu - PR, 2022. Accessed: Feb. 08, 2023. [Online]. Available: https://dspace.unila.edu.br/handle/123456789/7135
J. N. Maciel, V. H. Wentz, J. J. G. Ledesma, and O. H. Ando Junior, “Analysis of Artificial Neural Networks for Forecasting Photovoltaic Energy Generation with Solar Irradiance,” Brazilian Archives of Biology and Technology, vol. 64, no. spe, 2021, doi: 10.1590/1678-4324-75years-2021210131.
J. N. Maciel, J. J. G. Ledesma, and O. H. Ando Junior, “Hybrid prediction method of solar irradiance applied to short-term photovoltaic energy generation,” Renewable and Sustainable Energy Reviews, vol. 192, p. 114185, Mar. 2024, doi: 10.1016/j.rser.2023.114185.
R. C. Gonzalez and R. E. Woods, Digital image processing, 2nd ed., vol. 1. 2002.
S. Russell and P. Norvig, Artificial Intelligence: A Modern Approach, 3rd edition. United Kingdom: Pearson Education, 2016.
H. T. C. Pedro, D. P. Larson, and C. F. M. Coimbra, “A comprehensive dataset for the accelerated development and benchmarking of solar forecasting methods,” Journal of Renewable and Sustainable Energy, vol. 11, no. 3, p. 036102, May 2019, doi: 10.1063/1.5094494.
K. Lappalainen and S. Valkealahti, “Photovoltaic mismatch losses caused by moving clouds,” Solar Energy, vol. 158, no. October, pp. 455–461, 2017, doi: 10.1016/j.solener.2017.10.001.
R. Blaga, A. Sabadus, N. Stefu, C. Dughir, M. Paulescu, and V. Badescu, “A current perspective on the accuracy of incoming solar energy forecasting,” Prog Energy Combust Sci, vol. 70, pp. 119–144, 2019, doi: 10.1016/j.pecs.2018.10.003.
J. Antonanzas, N. Osorio, R. Escobar, R. Urraca, F. J. Martinez-de-Pison, and F. Antonanzas-Torres, “Review of photovoltaic power forecasting,” Solar Energy, vol. 136, pp. 78–111, 2016, doi: 10.1016/j.solener.2016.06.069.
R. Asghar, F. R. Fulginei, M. Quercio, and A. Mahrouch, “Artificial Neural Networks for Photovoltaic Power Forecasting: A Review of Five Promising Models,” IEEE Access, pp. 1–1, 2024, doi: 10.1109/ACCESS.2024.3420693.
F. Lin, Y. Zhang, and J. Wang, “Recent advances in intra-hour solar forecasting: A review of ground-based sky image methods,” Int J Forecast, Jan. 2022, doi: 10.1016/j.ijforecast.2021.11.002.
G. M. Tina, C. Ventura, S. Ferlito, and S. De Vito, “A State-of-Art-Review on Machine-Learning Based Methods for PV,” Applied Sciences, vol. 11, no. 16, p. 7550, Aug. 2021, doi: 10.3390/app11167550.
R. A. Rajagukguk, R. A. A. Ramadhan, and H.-J. Lee, “A Review on Deep Learning Models for Forecasting Time Series Data of Solar Irradiance and Photovoltaic Power,” Energies (Basel), vol. 13, no. 24, p. 6623, Dec. 2020, doi: 10.3390/en13246623.
A. L. Martinez and M. C. R. Dumer, “Adoption of IFRS and the Properties of Analysts’ Forecasts: The Brazilian Case,” SSRN Electronic Journal, Oct. 2012, doi: 10.2139/ssrn.2153173.
C. A. Gueymard, “A review of validation methodologies and statistical performance indicators for modeled solar radiation data: Towards a better bankability of solar projects,” Renewable and Sustainable Energy Reviews, vol. 39, pp. 1024–1034, 2014, doi: 10.1016/j.rser.2014.07.117.
B. Juncklaus Martins et al., “Systematic review of nowcasting approaches for solar energy production based upon ground-based cloud imaging,” Solar Energy Advances, vol. 2, p. 100019, 2022, doi: 10.1016/j.seja.2022.100019.
U. K. Das et al., “Forecasting of photovoltaic power generation and model optimization: A review,” Renewable and Sustainable Energy Reviews, vol. 81, no. August 2017, pp. 912–928, 2018, doi: 10.1016/j.rser.2017.08.017.
A. C. Gil, Como elaborar projetos de pesquisa, 6a Edição. São Paulo.: Atlas, 2017.
S. Visalakshi and V. Radha, “A literature review of feature selection techniques and applications: Review of feature selection in data mining,” in 2014 IEEE International Conference on Computational Intelligence and Computing Research, IEEE, Dec. 2014, pp. 1–6. doi: 10.1109/ICCIC.2014.7238499.
P. A. Morettin and W. de O. Bussab, Estatística básica, 9a edição. São Paulo: Saraivauni, 2017.
F. Pedregosa et al., “Scikit-learn: Machine Learning in Python,” Journal of Machine Learning Research, vol. 12, pp. 2825–2830, 2011.
E. Bisong, “Google Colaboratory,” in Building Machine Learning and Deep Learning Models on Google Cloud Platform, Berkeley, CA: Apress, 2019, pp. 59–64. doi: 10.1007/978-1-4842-4470-8_7.
A. C. Müller, M. A. C, and S. Guido, Introduction to Machine Learning with Python: A Guide for Data Scientists. O’Reilly Media, 2016.
E. Mining, Python Machine Learning: Understand Python Libraries (Keras, NumPy, Scikit-Lear, TensorFlow) for Implementing Machine Learning Models in Order to Build Intelligent Systems. Amazon Digital Services LLC - KDP Print US, 2019. [Online]. Available: https://books.google.com.br/books?id=qqQdzAEACAAJ
C. Nadeau and Y. Bengio, “Inference for the Generalization Error,” in Machine Learning, Lisa Hellerstein, Ed., Netherlands: The MIT Press, 2003, pp. 239–281. doi: https://doi.org/10.1023/A:1024068626366.
I. Guyon and A. Elisseeff, “An Introduction to Variable and Feature Selection,” in Journal of Machine Learning Research, Leslie Pack Kaelblin, Ed., Germany: MIT Press, 2003, pp. 1157–1182.
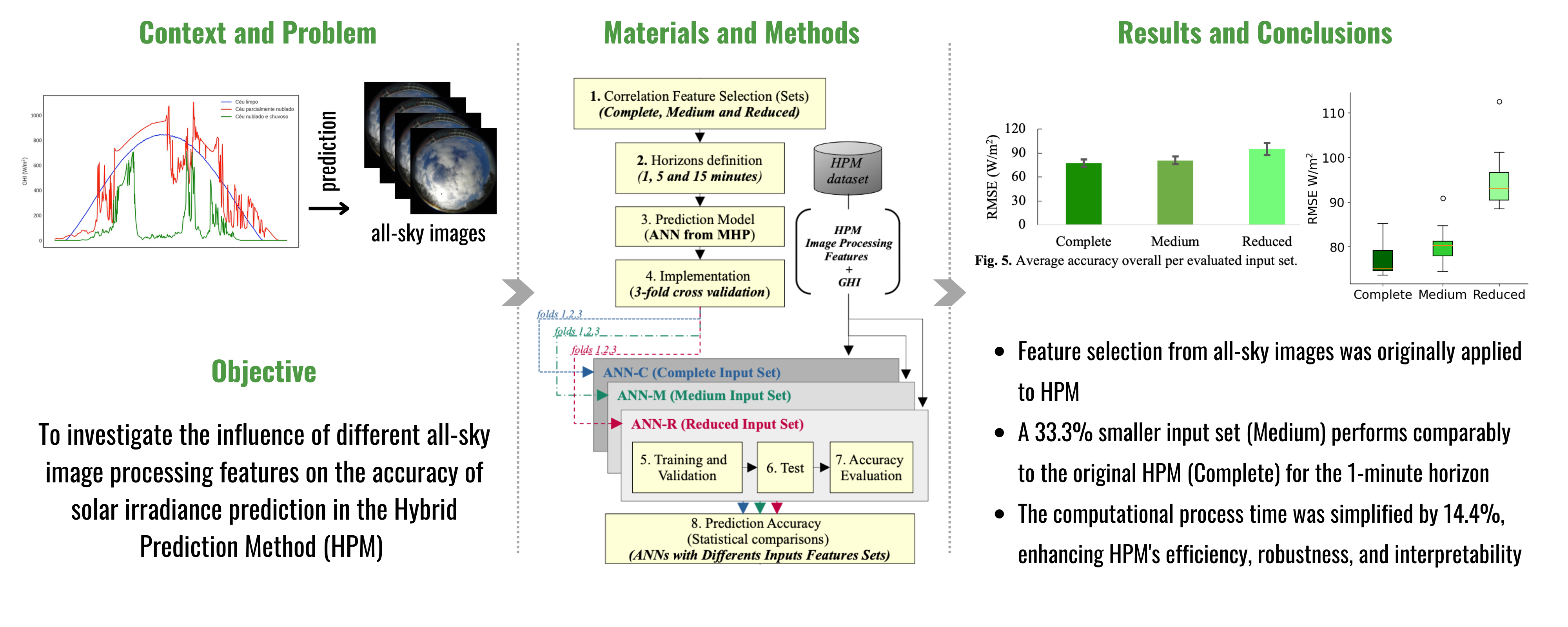