Computer Vision in Automatic Visceral Leishmaniasis Diagnosis: a Survey
Keywords:
Visceral Leishmaniasis, Computer Vision, Automatic DetectionAbstract
Visceral Leishmaniasis (VL) is a neglected disease that affects 1 billion people in tropical and subtropical countries. In Brazil, VL causes about 3,500 cases/year. Although this disease is lethal when left untreated, the number of cases is increasing. Thus, it is necessary to study current and safety technologies for VL diagnosis, treatment, and control. Specialized laboratories carry out the LV diagnosis, and this step has great automation power through automatic methods based on computer vision to aid in diagnosis. This work aims to present state-of-the-art research on computer vision techniques to detect VL in humans and provide a theoretical basis for developing computational systems to aid in diagnosing VL. This work's contributions are finding the methodologies and algorithms used in VL automatic detection and listing the gaps in developing those systems. As a result, we find out the lack of image databases and the use of deep learning techniques is still scarce. We conclude that methodologies that use the segmentation procedure perform better in terms of accuracy and that it is possible to develop a CAD system to help diagnose VL in humans.
Downloads
References
World Health Organization, “Global leishmaniasis surveillance, 2017–2018, and first report on 5 additional indicators,” Weekly Epi- demiological Record, vol. 95, no. 25, pp. 265 – 279, 2020.
M. O. Harhay, P. L. Olliaro, D. L. Costa, and C. H. N. Costa, “Urban parasitology: visceral leishmaniasis in brazil,” Trends in Parasitology, vol. 27, no. 9, pp. 403–409, 2011. [Online]. Available: https: //www.sciencedirect.com/science/article/pii/S147149221100064X
World of
able:
Health Organization, “Number of cases cutaneous leishmaniasis reported.” [Online]. Avail- https://www.who.int/data/gho/data/indicators/indicator- details/
GHO/number- of- cases- of- cutaneous- leishmaniasis- reported [4] ——, “Number of cases of visceral
maniasis reported.” [Online]. Available: //www.who.int/data/gho/data/indicators/indicator- details/GHO/ number- of- cases- of- visceral- leishmaniasis- reported
leish- https:
Pan American Health Organization, “Visceral leishmaniasis.” [Online]. Available: https://www.paho.org/en/topics/leishmaniasis/ visceral- leishmaniasis
G. Herrera, N. Barragán, N. Luna, D. Martínez, F. De Martino, J. Medina, S. Niño, L. Páez, A. Ramírez, L. Vega, et al., “An interactive database of leishmania species distribution in the americas,” Scientific data, vol. 7, no. 1, pp. 1–7, 2020.
A. Kumar, S. C. Pandey, and M. Samant, “Dna-based microarray stud- ies in visceral leishmaniasis: identification of biomarkers for diagnostic, prognostic and drug target for treatment,” Acta Tropica, vol. 208, p. 105512, 8 2020.
R. Cunha, R. Andreotti, M. C. R. B. de ..., and U. 2014, “Detection of leishmania infantum in lutzomyia longipalpis captured in campo grande, ms,” SciELO Brasil, 2014. [Online]. Available: https://www.scielo.br/scielo.php?pid= S1984- 29612014000200269&script=sci_arttext
R. Kumar and S. Nylén, “Immunobiology of visceral leishmaniasis,” Frontiers in immunology, vol. 3, p. 251, 2012.
L. A. Silva, H. D. Romero, G. A. N. Nascentes, R. T. Costa, V. Rodrigues, and A. Prata, “Antileishmania immunological tests for asymptomatic subjects living in a visceral leishmaniasis-endemic area in brazil,” The American journal of tropical medicine and hygiene, vol. 84, no. 2, p. 261, 2011.
J. M. Silva, D. A. Zacarias, L. C. D. Figueirêdo, M. R. A. Soares, E. A. Ishikawa, D. L. Costa, and C. H. Costa, “Bone marrow parasite burden among patients with new world kala-azar is associated with disease severity,” American Journal of Tropical Medicine and Hygiene, vol. 90, pp. 621–626, 2014.
R. R. Silva, F. H. Araujo, D. M. Ushizima, A. G. Bianchi, C. M. Carneiro, and F. N. Medeiros, “Radial feature descriptors for cell classification and recommendation,” Journal of Visual Communication and Image Representation, vol. 62, pp. 105–116, 2019.
F. H. Araújo, R. R. Silva, F. N. Medeiros, J. F. R. Neto, P. H. C. Oliveira, A. G. C. Bianchi, and D. Ushizima, “Active contours for overlapping cervical cell segmentation,” International Journal of Biomedical Engineering and Technology, vol. 35, no. 1, pp. 70–92, 2021. [Online]. Available: https://www.inderscienceonline.com/doi/ abs/10.1504/IJBET.2021.112834
E. D. Carvalho, A. O. Filho, R. R. Silva, F. H. Araújo, J. O. Diniz, A. C. Silva, A. C. Paiva, and M. Gattass, “Breast cancer diagnosis from histopathological images using textural features and CBIR,” Artificial Intelligence in Medicine, vol. 105, p. 101845, 2020.
R.R.V.eSilva,F.H.D.deAraujo,L.M.R.dosSantos,R.M.S. Veras, and F. N. S. de Medeiros, “Optic disc detection in retinal images using algorithms committee with weighted voting,” IEEE Latin America Transactions, vol. 14, no. 5, pp. 2446–2454, 2016.
M. Górriz, A. Aparicio, B. Raventós, V. Vilaplana, E. Sayrol, and D. López-Codina, “Leishmaniasis parasite segmentation and classifi- cation using deep learning,” in Articulated Motion and Deformable Objects, F. J. Perales and J. Kittler, Eds. Cham: Springer International Publishing, 2018, pp. 53–62.
S. Sundar and M. Rai, “Laboratory diagnosis of visceral leishmaniasis,” Clinical and Vaccine Immunology, vol. 9, no. 5, pp. 951–958, 2002. [Online]. Available: https://journals.asm.org/doi/abs/10.1128/CDLI.9. 5.951- 958.2002
G. Lebedev, H. Klimenko, S. Kachkovskiy, V. Konushin, I. Ryabkov, and A. Gromov, “Application of artificial intelligence methods to recognize pathologies on medical images,” vol. 126. Elsevier B.V., 2018, pp. 1171–1177.
G. Terán-Ángel, V. Rodríguez, R. Silva, O. Zerpa, H. Schallig, M. Ul- rich, and M. Cabrera, “Non invasive diagnostic tools for visceral leishmaniasis: a comparison of the immunoserological tests dat, rk26 and rk39,” Biomedica, vol. 30, no. 1, pp. 39–45, 2010.
M. de Paiva-Cavalcanti, R. C. S. de Morais, R. Pessoa-e Silva, L. A. M. Trajano-Silva, S. d. C. Goncalves-de Albuquerque, D. d. H. C. Tavares, M. C. A. Brelaz-de Castro, V. R. A. Pereira, et al., “Leishmaniases diagnosis: an update on the use of immunological and molecular tools,” Cell & bioscience, vol. 5, no. 1, pp. 1–10, 2015.
J. Cunningham, E. Hasker, P. Das, S. El Safi, H. Goto, D. Mondal, M. Mbuchi, M. Mukhtar, A. Rabello, S. Rijal, et al., “A global comparative evaluation of commercial immunochromatographic rapid diagnostic tests for visceral leishmaniasis,” Clinical infectious diseases, vol. 55, no. 10, pp. 1312–1319, 2012.
L. d. A. Silva, H. D. Romero, A. Prata, R. T. Costa, E. Nascimento, S. F. G. Carvalho, and V. Rodrigues, “Immunologic tests in patients after clinical cure of visceral leishmaniasis,” The American journal of tropical medicine and hygiene, vol. 75, no. 4, pp. 739–743, 2006.
O. P. Singh and S. Sundar, “Developments in diagnosis of visceral leishmaniasis in the elimination era,” Journal of parasitology research, vol. 2015, 2015.
J. M. Burns, W. G. Shreffler, D. R. Benson, H. W. Ghalib, R. Badaro, and S. G. Reed, “Molecular characterization of a kinesin-related antigen of leishmania chagasi that detects specific antibody in african and american visceral leishmaniasis.” Proceedings of the National Academy of Sciences, vol. 90, no. 2, pp. 775–779, 1993.
S. F. G. Carvalho, E. M. Lemos, R. Corey, and R. Dietze, “Performance of recombinant k39 antigen in the diagnosis of brazilian visceral leishmaniasis,” The American journal of tropical medicine and hygiene, vol. 68, no. 3, pp. 321–324, 2003.
S. Sundar, R. Singh, K. Bharti, R. Maurya, I. Delafosse, and P. Jacquier, “Evaluation of a new rapid immunochromatographicdiagnostic test (diamed-it leish) for indian visceral leishmaniasis and pkdl,” in The American Society of Tropical Medicine and Hygiene: ASTMH 52 nd Annual Meeting, 2003, pp. 3–7.
M. Boelaert, K. Verdonck, J. Menten, T. Sunyoto, J. van Griensven, F. Chappuis, and S. Rijal, “Rapid tests for the diagnosis of visceral leishmaniasis in patients with suspected disease,” Cochrane Database of Systematic Reviews, no. 6, 2014. [Online]. Available: https://doi.org//10.1002/14651858.CD009135.pub2
V. Peruhype-Magalhães, T. S. Machado-de Assis, and A. Rabello, “Use of the kala-azar detect® and it-leish® rapid tests for the diagnosis of visceral leishmaniasis in brazil,” Memorias do Instituto Oswaldo Cruz, vol. 107, pp. 951–952, 2012.
S. C. Mendonça, W. J. Souza, M. P. Nunes, M. C. Marzochi, and S. G. Coutinho, “Indirect immunofluorescence test in new world leishmaniasis: serological and clinical relation-ship,” Memórias do
Instituto Oswaldo Cruz, vol. 83, no. 3, pp. 347–355, 1988.
A. Harith, A. Kolk, P. Kager, J. Leeuwenburg, F. Faber, R. Muigai, S. Kiugu, and J. Laarman, “Evaluation of a newly developed direct agglutination test (dat) for serodiagnosis and sero-epidemiological studies of visceral leishmaniasis: comparison with ifat and elisa,” Transactions of the Royal Society of Tropical Medicine and Hygiene, vol. 81, no. 4, pp. 603–606, 1987. [Online]. Available:
https://www.sciencedirect.com/science/article/pii/0035920387904238 [31] E. A. E. R. Mohammed, E. Wright, A. Rahman, A. Kolk, J. Laarman, and K. Pondman, “Serodiagnosis of sudanese visceral and mucosal leishmaniasis: comparison of elisa-immunofluorescence and indirect haemagglutination,” Transactions of the Royal Society of Tropical Medicine and Hygiene, vol. 80, no. 2, pp. 271–274, 1986. [Online]. Available: https://www.sciencedirect.com/science/article/pii/
J. C. Neves, H. Castro, A. Tomás, M. Coimbra, and H. Proença,
“Detection and separation of overlapping cells based on contour concavity for leishmania images,” Cytometry Part A, vol. 85, no. 6, pp. 491–500, 2014. [Online]. Available: https://onlinelibrary.wiley. com/doi/abs/10.1002/cyto.a.22465
P. Srivastava, A. Dayama, S. Mehrotra, and S. Sundar, “Diagnosis of visceral leishmaniasis,” Transactions of The Royal Society of Tropical Medicine and Hygiene, vol. 105, no. 1, pp. 1–6, 01 2011. [Online]. Available: https://doi.org/10.1016/j.trstmh.2010.09.006
G. Srividya, A. Kulshrestha, R. Singh, and P. Salotra, “Diagnosis of visceral leishmaniasis: developments over the last decade,” Parasitol- ogy research, vol. 110, no. 3, pp. 1065–1078, 2012.
H. Sakkas, C. Gartzonika, and S. Levidiotou, “Laboratory diagnosis of human visceral leishmaniasis,” Journal of vector borne diseases, vol. 53, no. 1, p. 8, 2016.
S. Sundar and O. P. Singh, “Molecular diagnosis of visceral leishma- niasis,” Molecular diagnosis & therapy, vol. 22, no. 4, pp. 443–457, 2018.
E. K. Elmahallawy, A. S. Martínez, J. Rodriguez-Granger, Y. Hoyos- Mallecot, A. Agil, J. M. N. Mari, and J. G. Fernández, “Diagnosis of leishmaniasis,” The Journal of Infection in Developing Countries, vol. 8, no. 08, pp. 961–972, 2014.
A. Bermejo Rodriguez, J. M. Ruiz Giardin, J. Garcia Martinez, J. V. San Martin Lopez, A. Castaneda de la Mata, D. Lopez Lacomba, J. Jaqueti Aroca, and S. Walter, “Diagnostic model of visceral leishmaniasis based on bone marrow findings. study of patients with clinical suspicion in which the parasite is not observed,” European Journal of Internal Medicine, vol. 69, pp. 42–49, 2019. [Online]. Available: https://www.sciencedirect.com/science/article/pii/ S095362051930305X
C. H. Chen, Handbook of pattern recognition and computer vision. World Scientific, 2015.
J. R. Parker, Algorithms for image processing and computer vision. John Wiley & Sons, 2010.
B. Zhang, “Computer vision vs. human vision,” in 9th IEEE Interna- tional Conference on Cognitive Informatics (ICCI’10), 2010, pp. 3–3. [42] J. Salazar, M. Vera, Y. Huérfano, M. I. Vera, E. Gelvez-Almeida, and O. Valbuena, “Semi-automatic detection of the evolutionary forms of visceral leishmaniasis in microscopic blood smears,” Journal of Physics: Conference Series, vol. 1386, p. 012135, nov 2019. [Online].
Available: https://doi.org/10.1088/1742- 6596/1386/1/012135
E. H. Shortliffe, E. H. Shortliffe, J. J. Cimino, and J. J. Cimino, Biomedical informatics: computer applications in health care and
biomedicine. Springer, 2014.
M. Nixon and A. Aguado, Feature extraction and image processing for
computer vision. Academic press, 2019.
R. Scherer, Computer vision methods for fast image classification and
retrieval. Springer, 2020.
B. Kitchenham, “Procedures for performing systematic reviews,” Keele,
UK, Keele University, vol. 33, no. 2004, pp. 1–26, 2004.
F. Ouertani, H. Amiri, J. Bettaib, R. Yazidi, and A. Ben Salah, “Adaptive automatic segmentation of leishmaniasis parasite in indi- rect immunofluorescence images,” in 2014 36th Annual International Conference of the IEEE Engineering in Medicine and Biology Society,
, pp. 4731–4734.
B. R. Jipkate and V. Gohokar, “A comparative analysis of fuzzy c-
means clustering and k means clustering algorithms,” International Journal Of Computational Engineering Research, vol. 2, no. 3, pp. 737–739, 2012.
J. C. Neves, H. Castro, H. Proença, and M. Coimbra, “Automatic an- notation of leishmania infections in fluorescence microscopy images,” in Image Analysis and Recognition, M. Kamel and A. Campilho, Eds.
Berlin, Heidelberg: Springer Berlin Heidelberg, 2013, pp. 613–620.
T. Lindeberg, “Scale-space theory in computer vision,” Kluwer Aca-
demic Publishers, 1994.
P. Leal, L. Ferro, M. Marques, S. Romão, T. Cruz, A. M. Tomá,
H. Castro, and P. Quelhas, “Automatic assessment of leishmania infection indexes on in vitro macrophage cell cultures,” in Image Analysis and Recognition, A. Campilho and M. Kamel, Eds. Berlin, Heidelberg: Springer Berlin Heidelberg, 2012, pp. 432–439.
L. Ferro, M. Marques, P. Leal, S. Romão, T. Cruz, A. M. Tomás, H. Castro, and P. Quelhas, “Automatic spectral unmixing of leishmania infection macrophage cell cultures image,” in Image Analysis and Recognition, M. Kamel and A. Campilho, Eds. Berlin, Heidelberg: Springer Berlin Heidelberg, 2013, pp. 621–629.
P. A. Nogueira, “Determining leishmania infection levels by automatic analysis of microscopy images,” Master’s thesis, Department of Com- puter Science, University of Porto, 2011.
F. Ouertani, H. Amiri, J. Bettaib, R. Yazidi, and A. Ben Salah, “Hybrid segmentation of fluorescent leschmania-infected images using a watersched and combined region merging based method,” in 2016 38th Annual International Conference of the IEEE Engineering in Medicine and Biology Society (EMBC), 2016, pp. 3910–3913.
K. Haris, S. Efstratiadis, N. Maglaveras, and A. Katsaggelos, “Hybrid image segmentation using watersheds and fast region merging,” IEEE Transactions on Image Processing, vol. 7, no. 12, pp. 1684–1699, 1998.
C. B. Moraes and L. M. Alcântara, “Quantification of parasite loads by automated microscopic image analysis,” in Leishmania. Humana Press, New York, NY, 2019, pp. 279–288.
P. A. Nogueira and L. F. Teófilo, “Automatic analysis of leishmania infected microscopy images via gaussian mixture models,” in Advances in Artificial Intelligence - SBIA 2012, L. N. Barros, M. Finger, A. T. Pozo, G. A. Gimenénez-Lugo, and M. Castilho, Eds. Berlin, Heidelberg: Springer Berlin Heidelberg, 2012, pp. 82–91.
——, “A probabilistic approach to organic component detection in leishmania infected microscopy images,” in Artificial Intelligence Ap- plications and Innovations, L. Iliadis, I. Maglogiannis, and H. Pa- padopoulos, Eds. Berlin, Heidelberg: Springer Berlin Heidelberg, 2012, pp. 1–10.
J. L. Siqueira-Neto, S. Moon, J. Jang, G. Yang, C. Lee, H. K. Moon, E. Chatelain, A. Genovesio, J. Cechetto, and L. H. Freitas-Junior, “An image-based high-content screening assay for compounds targeting intracellular leishmania donovani amastigotes in human macrophages,” PLOS Neglected Tropical Diseases, vol. 6, no. 6, pp. 1–9, 06 2012. [Online]. Available: https://doi.org/10.1371/journal.pntd.0001671
N. Otsu, “A threshold selection method from gray-level histograms,” IEEE transactions on systems, man, and cybernetics, vol. 9, no. 1, pp. 62–66, 1979.
M. Hearst, S. Dumais, E. Osuna, J. Platt, and B. Scholkopf, “Support vector machines,” IEEE Intelligent Systems and their Applications, vol. 13, no. 4, pp. 18–28, 1998.
D. Tien and P. Nickolls, “A combined statistical and rule-based classifier,” in Images of the Twenty-First Century. Proceedings of the Annual International Engineering in Medicine and Biology Society,, 1989, pp. 1829 vol.6–.
D. A. Reynolds, “Gaussian mixture models.” Encyclopedia of biomet- rics, vol. 741, pp. 659–663, 2009.
A. Buades, B. Coll, and J. M. Morel, “On image denoising methods,” CMLA Preprint, vol. 5, 2004.
A. Kuijper, “On detecting all saddle points in 2d images,” Pattern Recognition Letters, vol. 25, no. 15, pp. 1665–1672, 2004.
M.I.Karavelas,“Arobustandefficientimplementationforthesegment voronoi diagram,” in International symposium on Voronoi diagrams in science and engineering, vol. 2004. Citeseer, 2004, pp. 51–62.
K.Suzuki,I.Horiba,andN.Sugie,“Linear-timeconnected-component labeling based on sequential local operations,” Computer Vision and Image Understanding, vol. 89, no. 1, pp. 1–23, 2003.
A. G. Gomes-Alves, A. F. Maia, T. Cruz, H. Castro, and A. M. Tomás, “Development of an automated image analysis protocol for quantification of intracellular forms of leishmania spp.” PLOS ONE, vol. 13, no. 8, pp. 1–15, 08 2018. [Online]. Available: https://doi.org/10.1371/journal.pone.0201747
E. Yazdanparast, A. Dos Anjos, D. Garcia, C. Loeuillet, H. R. Shahbazkia, and B. Vergnes, “Inspect, an open-source and versatile software for automated quantification of (leishmania) intracellular parasites,” PLOS Neglected Tropical Diseases, vol. 8, no. 5, pp. 1–10, 05 2014. [Online]. Available: https://doi.org/10.1371/journal. pntd.0002850
S. Beucher, “The watershed transformation applied to image segmen- tation,” Scanning Microscopy, vol. 1992, no. 6, p. 28, 1992.
R. C. Gonzalez and R. E. Woods, “Digital image processing addison- wesley,” Reading, Ma, 1992.
R. Maini and H. Aggarwal, “Study and comparison of various image edge detection techniques,” International journal of image processing (IJIP), vol. 3, no. 1, pp. 1–11, 2009.
World Health Organization, “Leishmaniasis.” [Online]. Available: https://www.who.int/news- room/fact- sheets/detail/leishmaniasis
D. Li and Y. Dong, “Deep learning,” Foundations and trends in signal processing, vol. 7, pp. 197–387, 2014.
O. Ronneberger, P. Fischer, and T. Brox, “U-net: Convolutional net- works for biomedical image segmentation,” in Medical Image Com- puting and Computer-Assisted Intervention – MICCAI 2015, N. Navab, J. Hornegger, W. M. Wells, and A. F. Frangi, Eds. Cham: Springer International Publishing, 2015, pp. 234–241.
N. Suman Gupta, “Visceral leishmaniasis: experimental models for drug discovery,” The Indian journal of medical research, vol. 133, no. 1, p. 27, 2011.
A. M. M. Farahi, H. Rabbani, M. Farahi, H. Rabbani, and A. Talebi, “Automatic boundary extraction of leishman bodies in bone marrow samples from patients with visceral leishmaniasis,” Journal of Isfahan Medical School, vol. 32, no. 286, pp. 726–739, 2014. [Online]. Avail- able: https://sites.google.com/site/hosseinrabbanikhorasgani/datasets-1/ dataset- of- leishmania- parasite- in- microscopic- images
A. Isaza-Jaimes, V. Bermúdez, A. Bravo, J. S. Castrillo, J. D. H. Lalinde, C. A. Fossi, A. Flórez, and J. E. Rodríguez, “A computational approach for leishmania genus protozoa detection in bone marrow sam- ples from patients with visceral leishmaniasis,” Archivos Venezolanos de Farmacología y Terapéutica, vol. 39, no. 7, pp. 908–920, 2020.
G. Coelho, A. R. Galvão Filho, R. Viana-de Carvalho, G. Teodoro- Laureano, S. Almeida-da Silveira, C. Eleutério-da Silva, R. M. P. Pereira, A. d. S. Soares, T. W. d. L. Soares, A. Gomes-da Silva, H. B. Napolitano, and C. J. Coelho, “Microscopic image segmentation to quantification of leishmania infection in macrophages,” Fronteiras: Journal of Social, Technological and Environmental Science, vol. 9, no. 1, pp. 488–498, mar. 2020.
J. Serra, “Image analysis and mathematical morphol-ogy,” 1982.
M.Farahi,H.Rabbani,A.Talebi,O.Sarrafzadeh,andS.Ensafi,“Auto- matic segmentation of leishmania parasite in microscopic images using a modified cv level set method,” in Seventh International Conference on Graphic and Image Processing (ICGIP 2015), vol. 9817. International
Society for Optics and Photonics, 2015, p. 98170K.
H. Tan, H. Jiang, A. Dong, B. Yang, and L. Zhang, “C-v level set based cell image segmentation using color filter and morphology,” in 2014 International Conference on Information Science, Electronics and
Electrical Engineering, vol. 2, 2014, pp. 1073–1077.
E. Vocaturo, E. Zumpano, and P. Veltri, “Image pre-processing in computer vision systems for melanoma detection,” in 2018 IEEE International Conference on Bioinformatics and Biomedicine (BIBM),
, pp. 2117–2124.
——,“Imagepre-processingincomputervisionsystemsformelanoma
detection,” in 2018 IEEE International Conference on Bioinformatics
and Biomedicine (BIBM), 2018, pp. 2117–2124.
Y. Chen, T. Ogata, T. Ueyama, T. Takada, and J. Ota, “Automated
design of the field-of-view, illumination, and image pre-processing parameters of an image recognition system,” in 2017 13th IEEE Conference on Automation Science and Engineering (CASE), 2017, pp. 1079–1084.
R. B. Jeyavathana, R. Balasubramanian, and A. A. Pandian, “A survey: analysis on pre-processing and segmentation techniques for medical images,” International Journal of Research and Scientific Innovation (IJRSI), vol. 3, 2016.
M. Zare, H. Akbarialiabad, H. Parsaei, Q. Asgari, A. Alinejad, M. S. Bahreini, S. H. Hosseini, M. Ghofrani-Jahromi, R. Shahriarirad, Y. Amirmoezzi, et al., “A machine learning-based system for detecting leishmaniasis in microscopic images,” BMC infectious diseases, vol. 22, no. 1, pp. 1–6, 2022.
J. N. Itri, R. R. Tappouni, R. O. McEachern, A. J. Pesch, and S. H. Patel, “Fundamentals of diagnostic error in imaging,” Radiographics, vol. 38, no. 6, pp. 1845–1865, 2018.
F. H. Araújo, R. R. Silva, F. N. Medeiros, J. F. R. Neto, P. H. C. Oliveira, A. G. C. Bianchi, and D. Ushizima, “Active contours for overlapping cervical cell segmentation,” International Journal of Biomedical Engineering and Technology, vol. 35, no. 1, pp. 70–92, 2021.
M. Lai, “Deep learning for medical image segmentation,” arXiv preprint arXiv:1505.02000, 2015.
M. H. Hesamian, W. Jia, X. He, and P. Kennedy, “Deep learning tech- niques for medical image segmentation: achievements and challenges,” Journal of digital imaging, vol. 32, no. 4, pp. 582–596, 2019.
D. Liu and J. Yu, “Otsu method and K-means,” in Proceedings - 2009 9th International Conference on Hybrid Intelligent Systems, HIS 2009, vol. 1, 2009, pp. 344–349.
L. Cai, J. Gao, and D. Zhao, “A review of the application of deep learning in medical image classification and segmentation,” Annals of translational medicine, vol. 8, no. 11, 2020.
G. Litjens, T. Kooi, B. E. Bejnordi, A. A. A. Setio, F. Ciompi, M. Ghafoorian, J. A. Van Der Laak, B. Van Ginneken, and C. I. Sánchez, “A survey on deep learning in medical image analysis,” Medical image analysis, vol. 42, pp. 60–88, 2017.
D. Shen, G. Wu, and H.-I. Suk, “Deep learning in medical image analysis,” Annual review of biomedical engineering, vol. 19, pp. 221– 248, 2017.
F. Xing, Y. Xie, H. Su, F. Liu, and L. Yang, “Deep learning in microscopy image analysis: A survey,” IEEE Transactions on Neural Networks and Learning Systems, vol. 29, no. 10, pp. 4550–4568, 2018.
J.Rama,C.Nalini,andA.Kumaravel,“Imagepre-processing:enhance the performance of medical image classification using various data augmentation technique,” ACCENTS Transactions on Image Processing and Computer Vision, vol. 5, no. 14, p. 7, 2019.
TensorFlow, “Data augmentation.” [Online]. Available: https://www. tensorflow.org/tutorials/images/data_augmentation
P. Vieira, O. Sousa, D. Magalhães, R. Rabêlo, and R. Silva, “Detecting pulmonary diseases using deep features in x-ray images,” Pattern Recognition, vol. 119, p. 108081, 2021.
Q. Li, W. Cai, X. Wang, Y. Zhou, D. D. Feng, and M. Chen, “Medical image classification with convolutional neural network,” in 2014 13th international conference on control automation robotics & vision (ICARCV). IEEE, 2014, pp. 844–848.
S. M. Anwar, M. Majid, A. Qayyum, M. Awais, M. Alnowami, and M. K. Khan, “Medical image analysis using convolutional neural networks: a review,” Journal of medical systems, vol. 42, no. 11, pp. 1–13, 2018.
T. Kaur and T. K. Gandhi, “Deep convolutional neural networks with transfer learning for automated brain image classification,” Machine Vision and Applications, vol. 31, no. 3, pp. 1–16, 2020.
S. Liu and W. Deng, “Very deep convolutional neural network based image classification using small training sample size,” in 2015 3rd IAPR Asian conference on pattern recognition (ACPR). IEEE, 2015, pp. 730–734.
I. Goodfellow, Y. Bengio, and A. Courville, Deep Learning. MIT Press, 2016.
C. Shorten and T. M. Khoshgoftaar, “A survey on image data augmen- tation for deep learning,” Journal of big data, vol. 6, no. 1, pp. 1–48, 2019.
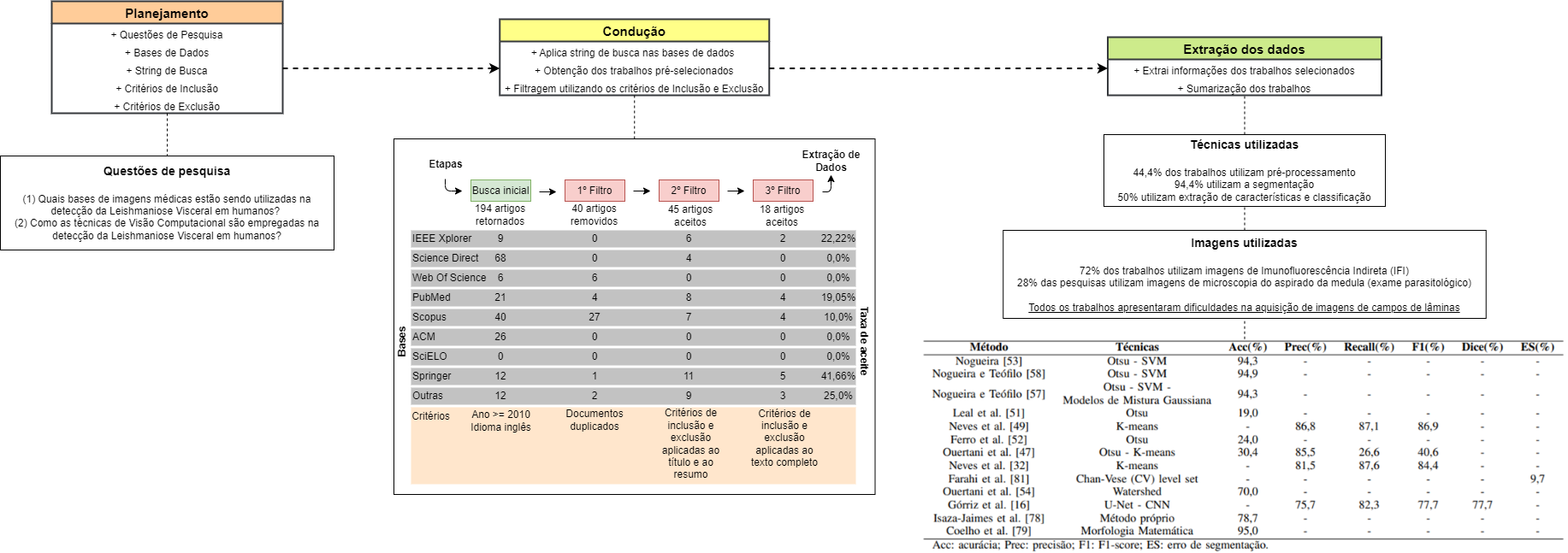