Spectral signature for quality assessment of anchovy fish meal (Engraulis Ringens) using Partial Least Squares
Keywords:
Fish meal, Spectral signature, Hyperspectral imageAbstract
Fishmeal is one of the most important foods for the aquaculture, livestock and poultry sectors in Latin America and the world. However, one of the constraints in the fishing industry is to measure online the physicochemical composition of this product and ensure its quality. In this study, the spectral signature of anchovy fish meal (Engraulis Ringens) has been obtained in wavelengths from 400 to 900 nm and has been related to its values of Protein, Ash, Moisture, Fat, Free Fatty Acids (FFA), total volatile basic nitrogen (TVB-N), sand, histamine and antioxidant remnant (REM.A/O). To analyze these relationships has been used the regression of Partial Least Squares (PLS) finding the best results to determine the Protein, Fat, Moisture and with some accuracy TBVN and Histamine. In addition, the most important spectral ranges have been identified for the calculation of the estimates. With these results we have a model to determine the physicochemical composition of anchovy fish meal based on the spectral signature that could be implemented in automatic systems for process control.
Downloads
References
R. L. Olsen and M. R. Hasan, “A limited supply of fishmeal: Impact on future increases in global aquaculture production,” Trends Food Sci. Technol., vol. 27, no. 2, pp. 120–128, 2012, doi:10.1016/j.tifs.2012.06.003.
A. G. J. Tacon and M. Metian, “Global overview on the use of fish meal and fish oil in industrially compounded aquafeeds: Trends and future prospects,” Aquaculture, vol. 285, no. 1–4, pp. 146–158, 2008, doi:10.1016/j.aquaculture.2008.08.015.
L. M. Potter, W. J. Pudelkiewics, L. Webster, and L. D. Matterson, “Metabolizable Energy and Digestibility Evaluation of Fish Meal for Chickens,” Poult. Sci., vol. 41, no. 6, pp. 1745–1752, 1961, doi:10.3382/ps.0411745.
S. W. Kim and R. A. Easter, “Nutritional value of fish meals in the diet for young pigs,” J. Anim. Sci., vol. 79, no. 7, pp. 1829–1839, 2001, doi:10.2527/2001.7971829x.
J. D. Berrocoso, M. P. Serrano, L. Cámara, P. G. Rebollar, and G. G. Mateos, “Influence of diet complexity on productive performance and nutrient digestibility of weanling pigs,” Anim. Feed Sci. Technol., vol. 171, no. 2–4, pp. 214–222, 2012, doi:10.1016/j.anifeedsci.2011.10.013.
S. M. Sinn, W. R. Gibbons, M. L. Brown, J. M. Derouchey, and C. L. Levesque, “Evaluation of microbially enhanced soybean meal as an alternative to fishmeal in weaned pig diets,” Animal, vol. 11, no. 5, pp. 784–793, 2017, doi:10.1017/S1751731116002020.
M. Hasan and M. Halwart, “Fish as feed inputs for aquaculture: Practices, sustainability and implications: a global synthesis,” in Fish as feed inputs for aquaculture: practices, sustainability and implications, 518th ed., no. 518, Rome: FAO Fisheries and Aquaculture Technical Paper, pp. 1–61, 2009.
R. W. Hardy, “Utilization of plant proteins in fish diets: Effects of global demand and supplies of fishmeal,” Aquac. Res., vol. 41, no. 5, pp. 770–776, 2010, doi:10.1111/j.1365-2109.2009.02349.x.
International Trade Center, “Trade Map - Estadísticas del comercio para el desarrolo internacional de las empresas.” [Online]. Available: https://www.trademap.org/Index.aspx, 2018.
Sociedad Nacional de Pesquería, “Harina de pescado / Fishmeal - Sociedad Nacional de Pesquería.” [Online]. Available: https://www.snp.org.pe/oferta_exportable/harina-de-pescado/, 2018.
J. M. Prats, Control Estad¡stico de Procesos mediante Análisis Multivariante de Imágenes. Valencia: Universidad Politécnica de Valencia, 2005.
M. Lin, M. Mousavi, M. Al-Holy, A. G. Cavinato, and B. A. Rasco, “Rapid near infrared spectroscopic method for the detection of spoilage in rainbow trout (Oncorhynchus mykiss) fillet,” J. Food Sci., vol. 71, no. 1,2006), doi:10.1111/j.1365-2621.2006.tb12400.x.
B. Gümüş and M. O. Balaban, “Prediction of the weight of aquacultured rainbow trout (Oncorhynchus mykiss) by image analysis,” J. Aquat. Food Prod. Technol., vol. 19, no. 3–4, pp. 227–237, 2010, doi:10.1080/10498850.2010.508869.
A. Folkestad et al., “Rapid and non-invasive measurements of fat and pigment concentrations in live and slaughtered Atlantic salmon (Salmo salar L.),” Aquaculture, vol. 280, no. 1–4, pp. 129–135, 2008, doi:10.1016/j.aquaculture.2008.04.037.
P. Katikou, S. I. Hughes, and D. H. F. Robb, “Lipid distribution within atlantic salmon (Salmo salar) fillets,” Aquaculture, vol. 202, no. 1–2, pp. 89–99, 2001, doi:10.1016/S0044-8486(01)00562-2.
J. P. Wold and T. Isaksson, “Non-destructive determination of fat and moisture in whole Atlantic salmon by near-infrared diffuse spectroscopy,” J. Food Sci., vol. 62, no. 4, pp. 734–736, 1997, doi:10.1111/j.1365-2621.1997.tb15446.x.
J. F. Wold et al., “Non-contact transflectance near infrared imaging for representative on-line sampling of dried salted coalfish (bacalao),” J. Near Infrared Spectrosc., vol. 14, no. 1, pp. 59–66, 2006, doi:10.1255/jnirs.587.
H.-J. He, D. Wu, and D.-W. Sun, “Nondestructive Spectroscopic and Imaging Techniques for Quality Evaluation and Assessment of Fish and Fish Products,” Crit. Rev. Food Sci. Nutr., vol. 55, no. 6, pp. 864–886, 2015, doi:10.1080/10408398.2012.746638.
Y. Zhang, L.-H. Tan, F. Cao, and Y. He, “Study on brand discrimination of fish oil based on multiple spectroscopy techniques,” Mod. Food Sci. Technol., vol. 30, no. 10, pp. 263–267, 2014, doi:10.13982/j.mfst.1673-9078.2014.10.044.
J. H. Cheng and D. W. Sun, “Partial Least Squares Regression (PLSR) Applied to NIR and HSI Spectral Data Modeling to Predict Chemical Properties of Fish Muscle,” Food Eng. Rev., vol. 9, no. 1, pp. 36–49, 2017, doi:10.1007/s12393-016-9147-1.
T. Isaksson, G. Tøgersen, A. Iversen, and K. I. Hildrum, “Non‐destructive determination of fat, moisture and protein in salmon fillets by use of near‐infrared diffuse spectroscopy,” J. Sci. Food Agric., vol. 69, no. 1, pp. 95–100, 1995, doi:10.1002/jsfa.2740690115.
D. Wu and D. W. Sun, “Colour measurements by computer vision for food quality control - A review,” Trends Food Sci. Technol., vol. 29, no. 1, pp. 5–20, 2013, doi:10.1016/j.tifs.2012.08.004.
Y. Huang and A. Cavinato, “Nondestructive Determination of Moisture and Sodium Chloride in Cured Atlantic Salmon (Salmo salar)(Teijin) Using Short-wavelength Near-infrared Spectroscopy (SW-NIR),” J. Food Sci., vol. 68, no. 2, 2003, doi:10.1111/j.1365-2621.2003.tb05698.x.
G. Viera-maza, “Aplicación de procesamiento de imágenes para clasificación de granos de cacao según su color interno,” Universidad de Piura, Piura, 2018.
A. Höskuldsson, “PLS regression methods” Journal of Chemometrics, vol. 2, no. 3, pp. 211–228, 1988, doi:10.1002/cem.1180020306.
R. Kohavi, “A Study of Cross-Validation and Bootstrap for Accuracy Estimation and Model Selection A Study of Cross-Validation and Bootstrap for Accuracy Estimation and Model Selection,” Int. Jt. Conf. Artif. Intell., no. March 2001, pp. 1137–1143, 2016.
L. Ståhle and S. Wold, “Partial Least Squares Analysis with Cross-Validation for the Two-Class Problem: A Monte Carlo Study,” J. Chemom., vol. 1, no. 3, pp. 185–196, 1987, doi:10.1002/cem.1180010306.
T. Mehmood, K. H. Liland, L. Snipen, and S. Sæbø, “A review of variable selection methods in Partial Least Squares Regression,” Chemom. Intell. Lab. Syst., vol. 118, pp. 62–69, 2012, doi:10.1016/j.chemolab.2012.07.010.
Ruiz, J., Soto, J., Ipanaque, W. Evaluation of spectral relation indexes of the Peruvian's cocoa beans during fermentation process. IEEE Latin America Transactions 14(6), 2862 – 2867, 2016, doi:10.1109 / TLA.2016.7555266.
Soto, J., Ruiz, J., Ipanaque, W., Chinguel, C. Nuevo índice hiperespectral para determinar el estado de fermentación en el análisis no destructivo del cacao violeta orgánico. Transacciones IEEE América Latina, 16 (9), 2435-2440, 2018, doi:10.1109 / TLA.2018.8789565.
Soto, J., Prieto, F., Ipanaque, W., Machacuay, J. Evaluación de la calidad del grano de cacao mediante el uso de imágenes hiperespectrales y técnicas de lógica difusa. Proc. SPIE 9534, Duodécima Conferencia Internacional sobre Control de Calidad por Visión Artificial 2015, 95340M, 2015, doi:10.1117/12.2182598.
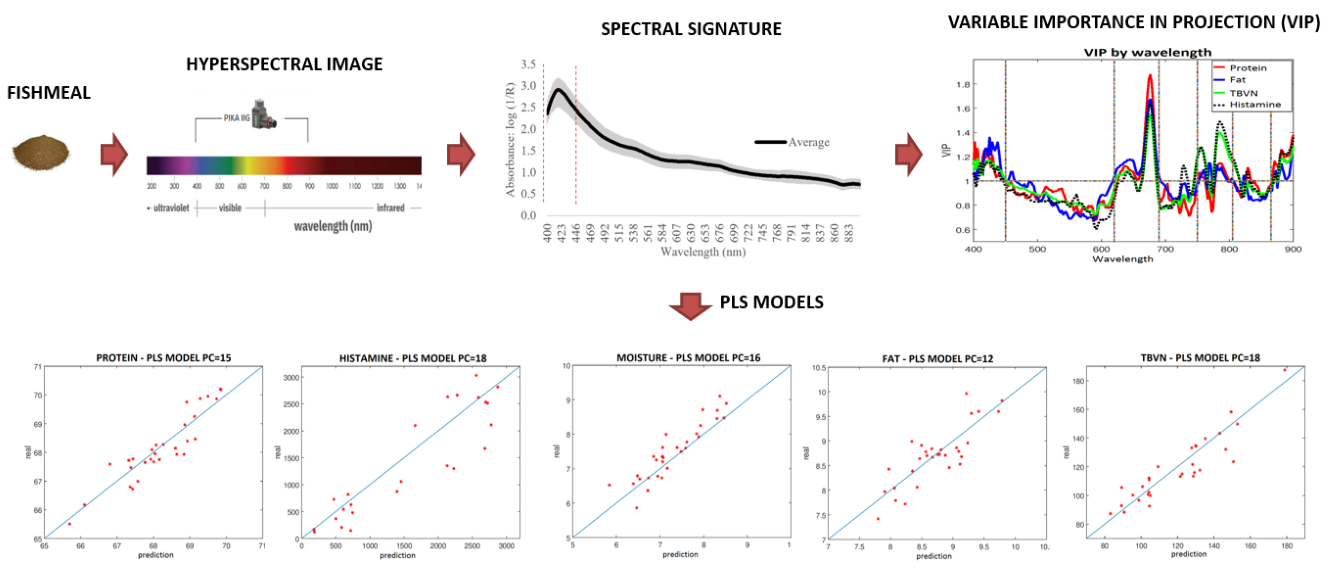