Model for Automatic Generation of Difficulty Curves in Digital Games
Keywords:
Digital Games, Difficulty Curves, User Experience, Apollo & RosettaAbstract
This paper presents a model for the generation of difficulty curves adaptive to the user's context. From studies on Flow theory combined with difficulty curves, element of digital game development process, mathematical models and previous data were used to create an adaptive difficulty curve based on user performance. It was used a research developed digital game as a case study, ‘The Incredible Adventures of Apollo & Rosetta in the Space’, which provided previous user data to perform the statistical analysis. Through the applied study, it is proposed the Difficulty Curves Generator Model (DCGM) for the generation of adaptive difficulty curves in digital games. Different approaches were used, and the final results demonstrated via simulation of the DCGM the ability of the generator to predict and adjust the game level for the player to experience, by calculating the relation between difficulty and player performance, aimed and focused in the Flow state.
Downloads
References
E. Murphy-Hill et al., “Cowboys, ankle sprains, and keepers of quality: How is video game development different from software development” in Proc. 36th Int. Conf. Softw. Eng., 2014, pp. 1-11.
S. Natkin, Video games and interactive media: A glimpse at new digital entertainment. Florida, EUA: CRC Press, 2006.
E. Byrne, Game Level Design (Vol. 6). Rockland, MA: Charles River Media. Inc., 2004.
O. Missura, T. Gärtner, “Player modeling for intelligent difficulty adjustment,” in Int. Conf. Discov. Sci., Springer., pp. 197-211, 2009.
M. Csikszentmihalyi, I. Csikszentmihalyi, Flow: The Psychology of Optimal Experience, NY: Harper Row, 1990.
J. Mossmann et al., “The Planning of Difficulty Curves in an Exergame for Inhibitory Control stimulation in a school intervention program: a pilot study,” Front. Psy., vol. 10, pp. 2271, 2019.
A. Wagner, “Um Modelo para Gerenciamento de Perfis de Entidades Através de Inferência em Trilhas,” M.S thesis, Dept. App. Comp., Unisinos, São Leopoldo, Brazil, 2013
G. Kendall and G. Whitwell, “An evolutionary approach for the tuning of a chess evaluation function using population dynamics” in Proc. 2001 Con. Evol. Comp., 2001, pp. 995–1002.
T. Tong, M. Chignell, “Developing a serious game for cognitive assessment: choosing settings and measuring performance,” in Proc. 2nd I. Symp. Chi. CHI. ACM, pp. 70-79, 2014.
R. Hunicke, “The case for dynamic difficulty adjustment in games,” in ACM SIGCHI Inter. Conf. Adv. Comp. Entert. Tech., 2005.
R. Koster, Theory of fun for game design, Sebastopol, CA, EUA: O'Reilly Media, Inc, 2013.
B. Cowley et al., “Toward an understanding of flow in video games,” Comp. Ent. (CIE), vol. 6, no. 2, pp. 20, 2008.
L. McMillan, “The Rational Design Handbook: An Intro to RLD,” Gamasutra, 2013. [Online]. Disponível em: http://www.gamasutra.com/blogs/LukeMcMillan/20130806/197147/The_Rational_Design_Handbook_An_Intro_to_RLD.php, Acesso: Ago., 2021.
O. Missura, “Dynamic Difficulty Adjustment,” Ph.D. dissertation, Inf. Inst., Universität Bonn, Germany, 2015.
A. Diamond, “Executive Functions,” A. Rev. Psy., vol. 64, pp. 135, 2013.
J. Mossmann et al.. “Evaluation of the usability and playability of an exergame for executive functions stimulation and its development process,”in Int. Conf. Eng. Psy. Cog. Erg, Vancouver, 2017, pp. 164-179.
G. M. Fitzmaurice et al., Applied Longitudinal Analysis, NJ, EUA: John Wiley & Sons, 2011.
H. Akaike, “Information theory and an extension of the maximum likelihood principle,” in Sel. Papers of H. A. NY, EUA, 1998, pp. 199-213.
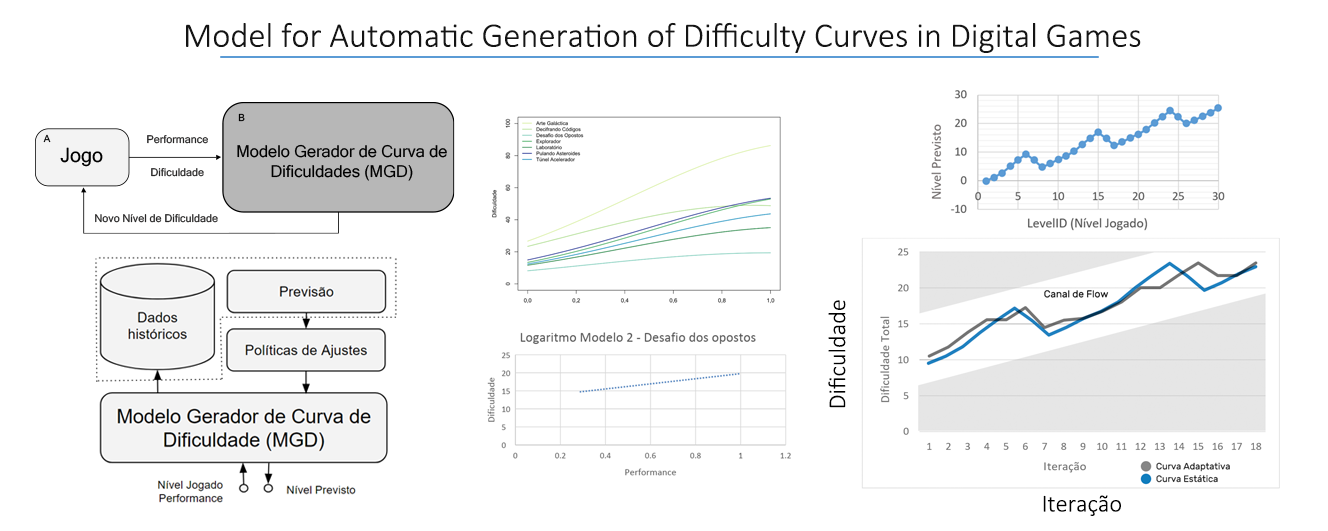