Optimization of the Containment Levels for the Reopening of Mexico City due to COVID-19
Keywords:
COVID-19, rein, deep q-learning, Genetic Algorithms, model simulationAbstract
One of the main problems that governments face in a pandemic is preserving the public health of the country whilst reducing the negative effects on the economy. In tackling the COVID-19 pandemic, there is an implicit trade-off between the economy and the reduction in the number of cases and deaths by the virus. If governmental restrictions to combat the pandemic are very strong, the economy could be seriously damaged. Conversely, if restrictions are very mild to minimize economic losses, it would be very difficult to stop the spread of the virus. It is necessary to find an optimization model to support government decisions balancing the impacts of COVID-19 in health and economic aspects. In this paper, we propose a methodology to find out the optimal number of days per contingency phase, in such a way that public health is prioritized and the damage to the economic impact is reduced. Then, our methodology is applied to one of the most densely populated areas in the world, Mexico City. Our methodology uses an SEIR (Susceptible-Exposed-Infected-Removed) model to simulate the evolution of the pandemic, and it can be implemented utilizing either a genetic algorithm or a Deep Q-Learning algorithm. For the experiments, we propose two scenarios in which the number of days for each phase is predicted within a 120-day period. The first experiment guarantees that the number of beds is not exceeded, considering the economic impact less relevant. By contrast, the second experiment reduces the number of days in which beds are exceeded as long as the economic losses are not higher than 20%, prioritizing the economy. According to the experiments, the implementation based on genetic algorithms has a higher performance.
Downloads
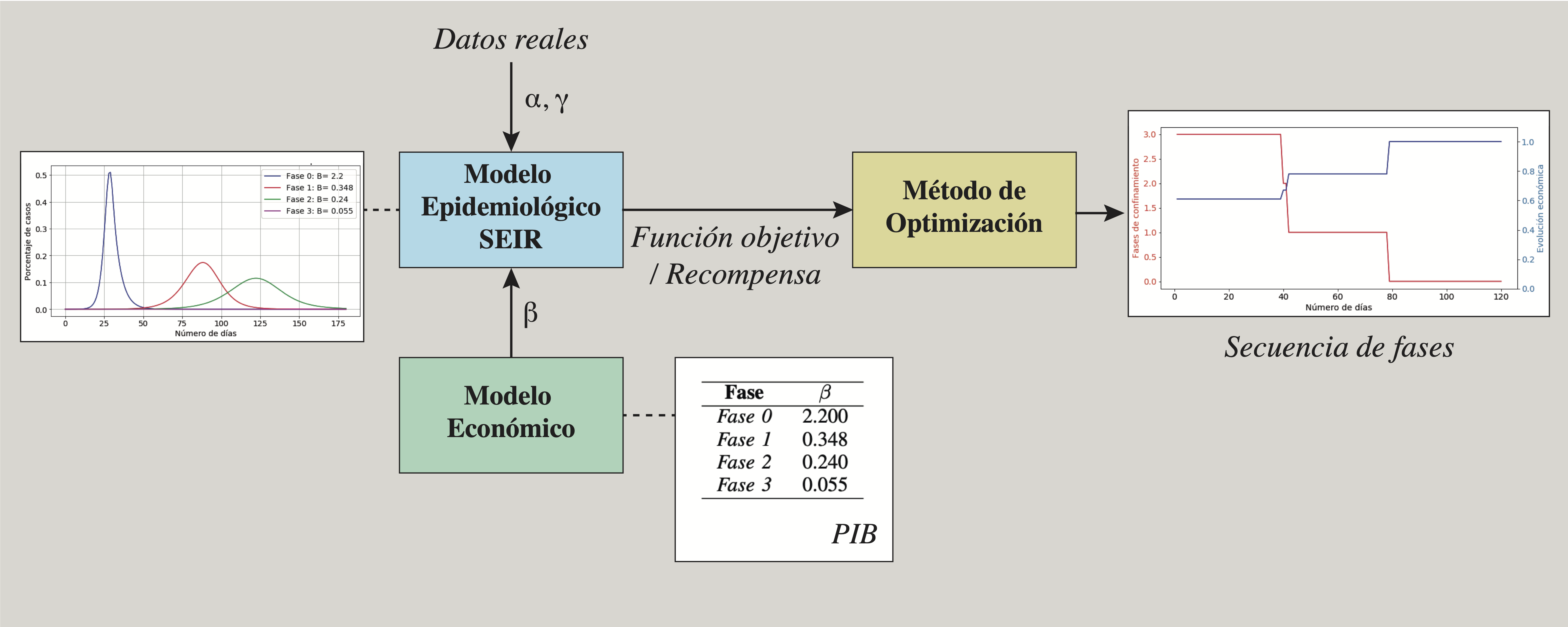