A Systematic Review of Radio Wave Techniques for Indoor Positioning Systems
Keywords:
Indoor positioning systems, Radio Waves, Radar Sensors, Antennas, Wireless.Abstract
Indoor human positioning has become crucial for applications such as health monitoring, security surveillance, human pose identification and rescue operations. However, achieving reliable indoor human positioning is challenging due to numerous constraints.This paper aims to compare and analyze radio waves techniques and approaches for indoor positioning,
providing a comprehensive review for human detection, positioning and activity recognition. A systematic review of the scientific literature and datasets was conducted. Four digital libraries, ACM Library Digital, IEEE Xplore, ScienceDirect and Spring Link were searched to identify results that met the selection criteria. A data extraction process was performed on the selected articles and datasets. The Parsifal platform was utilized to extract relevant information. After completing the systematic review, It was identified 26 eligible articles and extracted 11 methods for radio wave detection. The overview of indoor positioning system with radio waves was introduced. The most frequently mentioned tools in the articles for the capture stage were Radar Sensors, Wireless Sensor, and Antennas. For the processing stage, DNN Techniques, Processing Algorithms followed by Filtering, Fingerprint, Trilateration, and other machine learning algorithms formed the majority.
Downloads
References
D. A. Forsyth and J. Ponce, Computer vision: a modern approach. prentice hall professional technical reference, 2002. ISBN:
R. Szeliski, Computer vision: algorithms and applications. Springer
Nature, 2022. https://doi.org/10.1007/978-3-030-34372-9.
D. Gura, I. Markovskii, N. Khusht, I. Rak, and S. Pshidatok, “A complex for monitoring transport infrastructure facilities based on video
surveillance cameras and laser scanners,” Transportation Research
Procedia, vol. 54, pp. 775–782, 2021. https://doi.org/10.1016/j.trpro.
02.130.
E. Dilek and M. Dener, “Computer vision applications in intelligent
transportation systems: a survey,” Sensors, vol. 23, no. 6, p. 2938,
https://doi.org/10.3390/s23062938.
C.-Z. Dong and F. N. Catbas, “A review of computer vision–based
structural health monitoring at local and global levels,” Structural
Health Monitoring, vol. 20, no. 2, pp. 692–743, 2021. https://doi.
org/10.1177/1475921720935585.
C.-Z. Dong and F. N. Catbas, “A review of computer vision–based
structural health monitoring at local and global levels,” Structural
Health Monitoring, vol. 20, no. 2, pp. 692–743, 2021. https://doi.
org/10.1177/1475921720935585.
V. Jindal, S. Narayan Singh, and S. Suvra Khan, “Facial recognition
with computer vision,” in Machine Intelligence and Data Science
Applications: Proceedings of MIDAS 2021, pp. 313–330, Springer,
https://doi.org/10.1007/978-981-19-2347-0_24.
X. Song, “Emotional recognition and feedback of students in english
e-learning based on computer vision and face recognition algorithms,”
Entertainment Computing, p. 100847, 2024. https://doi.org/10.1016/j.
entcom.2024.100847.
X. Chen, X. Huang, Y. Wang, and X. Gao, “Combination of augmented
reality based brain-computer interface and computer vision for highlevel control of a robotic arm,” IEEE Transactions on Neural Systems
and Rehabilitation Engineering, vol. 28, no. 12, pp. 3140–3147, 2020.
https://doi.org/10.1109/tnsre.2020.3038209.
R. Anand, B. Madhusudan, and D. G. Bhalekar, “Computer vision and
agricultural robotics for disease control,” in Applications of Computer
Vision and Drone Technology in Agriculture 4.0, pp. 31–47, Springer,
https://doi.org/10.1007/978-981-99-8684-2_3.
H. Suri, H. Mahajan, K. K. Chauhan, A. Anand, and S. Sahana,
“Computer vision: a detailed review on augmented reality (ar), virtual
reality (vr), telehealth, and digital radiology,” Artificial Intelligence
in Medical Virology, pp. 99–115, 2023. https://doi.org/10.1007/
-981-99-0369-6_7.
Y. R. Ye, H. G. Ceng, Y. Qiang, G. S. Qiang, and L. Qiang,
“Advancing production operation safety with virtual reality solutions
and ai-driven computer vision,” 2024. https://doi.org/10.14733/cadaps.
s17.132-143.
A. Morar, A. Moldoveanu, I. Mocanu, F. Moldoveanu, I. E. Radoi,
V. Asavei, A. Gradinaru, and A. Butean, “A comprehensive survey
of indoor localization methods based on computer vision,” Sensors,
vol. 20, no. 9, p. 2641, 2020. https://doi.org/10.3390/s20092641.
O. Elharrouss, N. Almaadeed, and S. Al-Maadeed, “A review of video
surveillance systems,” Journal of Visual Communication and Image
Representation, vol. 77, p. 103116, 2021. https://doi.org/10.1016/j.jvcir.
103116.
S. Rishabh, P. Mayank, G. Swapnil, et al., “Smart home automation
using computer vision and segmented image processing,” in 2019
International Conference on Communication and Signal Processing
(ICCSP), pp. 0429–0433, IEEE, 2019. https://doi.org/10.1109/iccsp.
8697997.
B. Yang, S. Yang, X. Zhu, M. Qi, H. Li, Z. Lv, X. Cheng, and F. Wang,
“Computer vision technology for monitoring of indoor and outdoor
environments and hvac equipment: a review,” Sensors, vol. 23, no. 13,
p. 6186, 2023. https://doi.org/10.3390/s23136186.
J. Pincott, P. W. Tien, S. Wei, and J. K. Calautit, “Indoor fire detection
utilizing computer vision-based strategies,” Journal of Building Engineering, vol. 61, p. 105154, 2022. https://doi.org/10.1016/j.jobe.2022.
X. Liu, L. Xie, Y. Wang, J. Zou, J. Xiong, Z. Ying, and A. V.
Vasilakos, “Privacy and security issues in deep learning: A survey,”
IEEE Access, vol. 9, pp. 4566–4593, 2020. https://doi.org/10.1109/
access.2020.3045078.
S. Sahoo and B. Choudhury, “Exploring the use of computer vision
in assistive technologies for individuals with disabilities: A review,”
Journal of Future Sustainability, vol. 4, no. 3, pp. 133–148, 2024.
https://doi.org/10.5267/j.jfs.2024.7.002.
R. A. Waelen, “The ethics of computer vision: an overview in terms
of power,” AI and Ethics, vol. 4, no. 2, pp. 353–362, 2024. https:
//doi.org/10.1007/s43681-023-00272-x.
A. K. Mishra, S. Tiwari, A. K. Tyagi, and M. O. Arowolo, “Security,
privacy, trust, and provenance issues in internet of things–based edge
environment,” in IoT Edge Intelligence, pp. 233–263, Springer, 2024.
X. Zhou, T. Jin, Y. Dai, Y. Song, and Z. Qiu, “Md-pose: Human
pose estimation for single-channel uwb radar,” IEEE Transactions on
Biometrics, Behavior, and Identity Science, vol. 5, no. 4, pp. 449–463,
https://doi.org/10.1109/tbiom.2023.3265206.
Y. Song, T. Jin, Y. Dai, and X. Zhou, “Efficient through-wall human
pose reconstruction using uwb mimo radar,” IEEE Antennas and
Wireless Propagation Letters, vol. 21, no. 3, pp. 571–575, 2021.
https://doi.org/10.1109/lawp.2021.3138512.
Y. Song, T. Jin, Y. Dai, Y. Song, and X. Zhou, “Through-wall human
pose reconstruction via uwb mimo radar and 3d cnn,” Remote Sensing,
vol. 13, no. 2, p. 241, 2021. https://doi.org/10.3390/rs13020241.
F. Alkhawaja, M. Jaradat, and L. Romdhane, “Techniques of indoor
positioning systems (ips): A survey,” in 2019 Advances in Science and
Engineering Technology International Conferences (ASET), pp. 1–8,
IEEE, 2019. https://doi.org/10.1109/icaset.2019.8714291.
J. Singh, N. Tyagi, S. Singh, F. Ali, and D. Kwak, “A systematic review
of contemporary indoor positioning systems: Taxonomy, techniques,
and algorithms,” IEEE Internet of Things Journal, 2024. https://doi.
org/10.1109/jiot.2024.3416255.
O. Casha, “A comparative analysis and review of indoor positioning
systems and technologies,” 2024. https://doi.org/10.5772/intechopen.
T. Kim Geok, K. Zar Aung, M. Sandar Aung, M. Thu Soe, A. Abdaziz,
C. Pao Liew, F. Hossain, C. P. Tso, and W. H. Yong, “Review of indoor
positioning: Radio wave technology,” Applied Sciences, vol. 11, no. 1,
p. 279, 2020. https://doi.org/10.3390/app11010279.
M. Li, X. Qiu, S. Zhu, Z. Sheng, Y. Liu, Y. Zhao, X. Zhao,
R. You, S. Wang, and D. Bi, “Intelligent robotic arm for human pose
recognition based on teleoperation system,” in Journal of Physics:
Conference Series, vol. 2741, p. 012015, IOP Publishing, 2024. https:
//doi.org/10.1088/1742-6596/2741/1/012015
H. Hertz, Electric waves: being researches on the propagation of
electric action with finite velocity through space. Dover Publications,
https://doi.org/10.4324/9780429198960-14.
W. Gosling, Radio Antennas and Propagation: Radio Engineering Fundamentals. Newnes, 1998. https://doi.org/10.1016/b978-075063741-1/
-0.
C. Bai, H.-P. Ren, W.-Y. Zheng, and C. Grebogi, “Radio-wave communication with chaos,” IEEE Access, vol. 8, pp. 167019–167026, 2020.
https://doi.org/10.1109/access.2020.3022632.
A. V. Gurevich and E. E. Tsedilina, Long distance propagation
of HF radio waves. Springer, 1985. https://doi.org/10.1007/
-3-642-70249-5.
T. S. Rappaport and S. Sandhu, “Radio wave propagation for emerging
wireless personal communication systems,” Wireless Personal Communications: Research Developments, pp. 1–27, 1995. https://doi.org/10.
/978-1-4757-2368-7_1.
T. Kim Geok, K. Zar Aung, M. Sandar Aung, M. Thu Soe, A. Abdaziz,
C. Pao Liew, F. Hossain, C. P. Tso, and W. H. Yong, “Review of indoorpositioning: Radio wave technology,” Applied Sciences, vol. 11, no. 1,
p. 279, 2020. https://doi.org/10.3390/app11010279.
N. Soltanieh, Y. Norouzi, Y. Yang, and N. C. Karmakar, “A review
of radio frequency fingerprinting techniques,” IEEE Journal of Radio
Frequency Identification, vol. 4, no. 3, pp. 222–233, 2020. https://doi.
org/10.1109/jrfid.2020.2968369.
S. Subedi and J.-Y. Pyun, “A survey of smartphone-based indoor
positioning system using rf-based wireless technologies,” Sensors,
vol. 20, no. 24, p. 7230, 2020. https://doi.org/10.3390/s20247230.
K.-L. Du and M. N. Swamy, Wireless communication systems: from RF
subsystems to 4G enabling technologies. Cambridge University Press,
https://doi.org/10.1017/cbo9780511841453.
L. Cui, Z. Zhang, N. Gao, Z. Meng, and Z. Li, “Radio frequency
identification and sensing techniques and their applications—a review
of the state-of-the-art,” Sensors, vol. 19, no. 18, p. 4012, 2019. https:
//doi.org/10.3390/s19184012.
A. Jagannath, J. Jagannath, and P. S. P. V. Kumar, “A comprehensive
survey on radio frequency (rf) fingerprinting: Traditional approaches,
deep learning, and open challenges,” Computer Networks, vol. 219,
p. 109455, 2022. https://doi.org/10.1016/j.comnet.2022.109455.
S. A. Shah and F. Fioranelli, “Rf sensing technologies for assisted
daily living in healthcare: A comprehensive review,” IEEE Aerospace
and Electronic Systems Magazine, vol. 34, no. 11, pp. 26–44, 2019.
https://doi.org/10.1109/maes.2019.2933971.
L. Cui, Z. Zhang, N. Gao, Z. Meng, and Z. Li, “Radio frequency
identification and sensing techniques and their applications—a review
of the state-of-the-art,” Sensors, vol. 19, no. 18, p. 4012, 2019. https:
//doi.org/10.3390/s19184012.
M. H. Alsharif, S. Kim, and N. Kuruoglu, “Energy harvesting tech- ˘
niques for wireless sensor networks/radio-frequency identification: A
review,” Symmetry, vol. 11, no. 7, p. 865, 2019. https://doi.org/10.3390/
sym11070865.
T. Pfister, J. Charles, and A. Zisserman, “Flowing convnets for human
pose estimation in videos,” in Proceedings of the IEEE international
conference on computer vision, pp. 1913–1921, 2015. https://doi.org/
1109/iccv.2015.222.
M. Zhao, T. Li, M. Abu Alsheikh, Y. Tian, H. Zhao, A. Torralba, and
D. Katabi, “Through-wall human pose estimation using radio signals,”
in Proceedings of the IEEE conference on computer vision and pattern
recognition, pp. 7356–7365, 2018. https://doi.org/10.1109/cvpr.2018.
F. Adib, C.-Y. Hsu, H. Mao, D. Katabi, and F. Durand, “Capturing
the human figure through a wall,” ACM Transactions on Graphics
(TOG), vol. 34, no. 6, pp. 1–13, 2015. https://doi.org/10.1145/2816795.
S. S. Rahman, M. A. Kamaruzaman, R. A. Rashid, J. E. Calveen,
E. T. Tashbib, M. Imran, and M. A. Sarijari, “A survey of internet of
things based indoor positioning systems based on bluetooth low energy
beacon,” ELEKTRIKA-Journal of Electrical Engineering, vol. 23, no. 1,
pp. 43–50, 2024. https://doi.org/10.11113/elektrika.v23n1.449.
M. S. Hossain and R. J. Wandell, “Indoor positioning system for
smart spaces,” in 2024 6th International Conference on Electrical Engineering and Information & Communication Technology (ICEEICT),
pp. 1112–1117, IEEE, 2024. https://doi.org/10.1109/iceeict62016.2024.
M. Niang, M. Ndong, P. Canalda, F. Spies, I. Dioum, I. Diop,
and M. Abdel El Ghany, “A comparative study of machine-learning
algorithms for indoor localization based on the wi-fi fingerprint according to user postures,” in International Congress on Information
and Communication Technology, pp. 227–237, Springer, 2024. https:
//doi.org/10.1007/978-981-97-3305-7_18.
F. Firmansyah, F. Rahma, K. D. Irianto, and A. M. Shiddiqi, “Indoor
positioning system: A brief review of its technologies and signalfiltering techniques,” in 2024 International Conference on Smart Computing, IoT and Machine Learning (SIML), pp. 1–7, IEEE, 2024.
https://doi.org/10.1109/siml61815.2024.10578093.
V.-C. Ta, T.-K. Dao, D. Vaufreydaz, and E. Castelli, “Smartphone-based
user positioning in a multiple-user context with wi-fi and bluetooth,”
in 2018 International Conference on Indoor Positioning and Indoor
Navigation (IPIN), pp. 206–212, 2018. https://doi.org/10.1109/ipin.
8533809.
V. Vladislav and B. Marina, “Implementation of indoor positioning
methods: virtual hospital case,” Procedia Computer Science, vol. 193,
pp. 183–189, 2021. https://doi.org/10.1016/j.procs.2021.10.018.
T.-M. T. Dinh, N.-S. Duong, and Q.-T. Nguyen, “Developing a novel
real-time indoor positioning system based on ble beacons and smart-phone sensors,” IEEE Sensors Journal, vol. 21, no. 20, pp. 23055–
, 2021. https://doi.org/10.1109/jsen.2021.3106019.
L. Bai, F. Ciravegna, R. Bond, and M. Mulvenna, “A low cost
indoor positioning system using bluetooth low energy,” Ieee Access,
vol. 8, pp. 136858–136871, 2020. https://doi.org/10.1109/access.2020.
B. P. Guamán and J. Cordero, “Indoor positioning system using beacon
technology,” in 2020 15th Iberian Conference on Information Systems
and Technologies (CISTI), pp. 1–4, IEEE, 2020. https://doi.org/10.
/cisti49556.2020.9141009.
L. Bouse, S. A. King, and T. Chu, “Simplified indoor localization
using bluetooth beacons and received signal strength fingerprinting
with smartwatch,” Sensors, vol. 24, no. 7, p. 2088, 2024. https:
//doi.org/10.3390/s24072088.
J. Cecílio, K. Duarte, P. Martins, and P. Furtado, “Robustpathfinder:
handling uncertainty in indoor positioning techniques,” Procedia computer science, vol. 130, pp. 408–415, 2018. https://doi.org/10.1016/j.
procs.2018.04.061.
B. Andò, S. Baglio, R. Crispino, and V. Marletta, “An introduction
to indoor localization techniques. case of study: A multi-trilaterationbased localization system with user–environment interaction feature,”
Applied Sciences, vol. 11, no. 16, p. 7392, 2021. https://doi.org/10.
/app11167392.
T. W. Moleski and J. P. Wilhelm, “Trilateration positioning using hybrid
camera–lidar system with spherical landmark surface fitting,” Journal
of Guidance, Control, and Dynamics, vol. 45, no. 7, pp. 1213–1228,
https://doi.org/10.2514/1.g006248.
A. El-Naggar, A. Wassal, and K. Sharaf, “Indoor positioning using
wifi rssi trilateration and ins sensor fusion system simulation,” in
Proceedings of the 2019 2nd International Conference on Sensors,
Signal and Image Processing, pp. 21–26, 2019. https://doi.org/10.1145/
3365261.
Z. Xiong, Z. Y. Song, A. Scalera, F. Sottile, R. Tomasi, and M. A.
Spirito, “Enhancing wsn-based indoor positioning and tracking through
rfid technology,” in 2012 Fourth International EURASIP Workshop
on RFID Technology, pp. 107–114, 2012. https://doi.org/10.1109/rfid.
26.
M. Merenda, L. Catarinucci, R. Colella, D. Iero, F. G. Della Corte,
and R. Carotenuto, “Rfid-based indoor positioning using edge machine
learning,” IEEE Journal of Radio Frequency Identification, vol. 6,
pp. 573–582, 2022. https://doi.org/10.1109/jrfid.2022.3182819.
A. Vena, I. Illanes, L. Alidieres, B. Sorli, and F. Perea, “Rfid based
indoor localization system to analyze visitor behavior in a museum,”
in 2021 IEEE International Conference on RFID Technology and
Applications (RFID-TA), pp. 183–186, IEEE, 2021. https://doi.org/10.
/rfid-ta53372.2021.9617265.
A. A. N. Shirehjini and S. Shirmohammadi, “Improving accuracy and
robustness in hf-rfid-based indoor positioning with kalman filtering
and tukey smoothing,” IEEE Transactions on Instrumentation and
Measurement, vol. 69, no. 11, pp. 9190–9202, 2020. https://doi.org/
1109/tim.2020.2995281.
Z. Wei, J. Chen, H. Tang, and H. Zhang, “Rssi-based location fingerprint method for rfid indoor positioning: a review,” Nondestructive
Testing and Evaluation, pp. 1–29, 2023.
E. Matrosova and A. Tikhomirova, “Intelligent data processing received
from radio frequency identification system,” Procedia computer science, vol. 145, pp. 332–336, 2018. https://doi.org/10.1016/j.procs.2018.
080.
G. Xu, P. Sharma, X. Hui, and E. C. Kan, “3-d indoor device-free object
detection by passive radio frequency identification,” IEEE Transactions
on Instrumentation and Measurement, vol. 70, pp. 1–13, 2021. https:
//doi.org/10.1109/tim.2021.3059309.
Y. Ma, W. Ning, B. Wang, and X. Liang, “A data augmentation-based
method for robust device-free localization in changing environments
of passive radio frequency identification system,” IEEE Transactions
on Instrumentation and Measurement, vol. 70, pp. 1–13, 2021. https:
//doi.org/10.1109/tim.2021.3065426.
N. H. A. Wahab, N. Sunar, S. H. Ariffin, K. Y. Wong, Y. Aun,
et al., “Indoor positioning system: A review,” International Journal
of Advanced Computer Science and Applications, vol. 13, no. 6, 2022.
https://doi.org/10.14569/ijacsa.2022.0130659.
G. M. Mendoza-Silva, J. Torres-Sospedra, and J. Huerta, “A metareview of indoor positioning systems,” Sensors, vol. 19, no. 20, p. 4507,
https://doi.org/10.3390/s19204507.
T. Kim Geok, K. Zar Aung, M. Sandar Aung, M. Thu Soe, A. Abdaziz,
C. Pao Liew, F. Hossain, C. P. Tso, and W. H. Yong, “Review of indoor positioning: Radio wave technology,” Applied Sciences, vol. 11, no. 1,
p. 279, 2020. https://doi.org/10.3390/app11010279.
B. A. Kitchenham, P. Brereton, M. Turner, M. K. Niazi, S. Linkman,
R. Pretorius, and D. Budgen, “Refining the systematic literature review
process—two participant-observer case studies,” Empirical Software
Engineering, vol. 15, pp. 618–653, 2010. https://doi.org/10.1007/
s10664-010-9134-8.
“Parsif.al.”
I. Cushman, D. B. Rawat, A. Bhimraj, and M. Fraser, “Experimental
approach for seeing through walls using wi-fi enabled software defined
radio technology,” Digital Communications and Networks, vol. 2, no. 4,
pp. 245–255, 2016. https://doi.org/10.1016/j.dcan.2016.09.001.
U. M. Khan, R. H. Venkatnarayan, and M. Shahzad, “Using rf signals to
generate indoor maps,” ACM Transactions on Sensor Networks, vol. 19,
no. 1, pp. 1–30, 2023. https://doi.org/10.1145/3534121.
K. Oguchi, S. Maruta, and D. Hanawa, “Human positioning estimation
method using received signal strength indicator (rssi) in a wireless
sensor network,” Procedia Computer Science, vol. 34, pp. 126–132,
https://doi.org/10.1016/j.procs.2014.07.066.
A. Al-Habashna and G. Wainer, “Rssi-based indoor localization with
lte-a ultra-dense networks,” in 2020 International Symposium on Performance Evaluation of Computer and Telecommunication Systems
(SPECTS), pp. 1–7, IEEE, 2020.
J. Lu, “A new indoor location algorithm based on radio frequency
fingerprint matching,” IEEE Access, vol. 8, pp. 83290–83297, 2020.
https://doi.org/10.1109/access.2020.2989137.
M. Liu, J. Wang, N. Zhao, Y. Chen, H. Song, and F. R. Yu, “Radio frequency fingerprint collaborative intelligent identification using
incremental learning,” IEEE Transactions on Network Science and
Engineering, vol. 9, no. 5, pp. 3222–3233, 2021. https://doi.org/10.
/tnse.2021.3103805.
A. Buonanno, M. D’Urso, G. Prisco, M. Felaco, L. Angrisani, M. Ascione, R. S. L. Moriello, and N. Pasquino, “A new measurement
method for through-the-wall detection and tracking of moving targets,”
Measurement, vol. 46, no. 6, pp. 1834–1848, 2013. https://doi.org/10.
/j.measurement.2012.12.021.
S. Yue, H. He, P. Cao, K. Zha, M. Koizumi, and D. Katabi, “Cornerradar: Rf-based indoor localization around corners,” Proceedings of
the ACM on Interactive, Mobile, Wearable and Ubiquitous Technologies, vol. 6, no. 1, pp. 1–24, 2022. https://doi.org/10.1145/3517226.
W. Jiang, H. Xue, C. Miao, S. Wang, S. Lin, C. Tian, S. Murali, H. Hu,
Z. Sun, and L. Su, “Towards 3d human pose construction using wifi,”
in Proceedings of the 26th Annual International Conference on Mobile
Computing and Networking, pp. 1–14, 2020. https://doi.org/10.1145/
3380900.
S. Ding, Z. Chen, T. Zheng, and J. Luo, “Rf-net: A unified metalearning framework for rf-enabled one-shot human activity recognition,” in Proceedings of the 18th Conference on Embedded Networked
Sensor Systems, pp. 517–530, 2020. https://doi.org/10.1145/3384419.
S. Fang, R. Alterovitz, and S. Nirjon, “Non-line-of-sight around the
corner human presence detection using commodity wifi devices,” in
Proceedings of the 1st ACM International Workshop on Device-Free
Human Sensing, pp. 22–26, 2019. https://doi.org/10.1145/3360773.
[85] P.-F. Gimenez, J. Roux, E. Alata, G. Auriol, M. Kaâniche, and
V. Nicomette, “Rids: Radio intrusion detection and diagnosis system
for wireless communications in smart environment,” ACM Transactions
on Cyber-Physical Systems, vol. 5, no. 3, pp. 1–1, 2021. https:
//doi.org/10.1145/3441458.
S. Harada, M. Mochizuki, K. Murao, and N. Nishio, “A wi-fi positioning method considering radio attenuation of human body,” in
Proceedings of the 2018 ACM International Joint Conference and 2018
International Symposium on Pervasive and Ubiquitous Computing and
Wearable Computers, pp. 1472–1478, 2018. https://doi.org/10.1145/
3267513.
F. Abuhoureyah, Y. C. Wong, A. S. B. M. Isira, and M. N. Al-Andoli,
“Csi-based location independent human activity recognition using deep
learning,” Human-Centric Intelligent Systems, vol. 3, no. 4, pp. 537–
, 2023. https://doi.org/10.1007/s44230-023-00047-x.
G. A. S. Surek, L. O. Seman, S. F. Stefenon, V. C. Mariani, and L. d. S.
Coelho, “Video-based human activity recognition using deep learning
approaches,” Sensors, vol. 23, no. 14, p. 6384, 2023. https://doi.org/
3390/s23146384.
V. Pasku, M. L. Fravolini, and A. Moschitta, “Effects of antenna
directivity on rf ranging when using space diversity techniques,
Measurement, vol. 98, pp. 429–438, 2017. https://doi.org/10.1016/j.
measurement.2015.11.030.
T. Ni, Y. Chen, K. Song, and W. Xu, “A simple and fast human
activity recognition system using radio frequency energy harvesting,” in
Adjunct Proceedings of the 2021 ACM International Joint Conference
on Pervasive and Ubiquitous Computing and Proceedings of the 2021
ACM International Symposium on Wearable Computers, pp. 666–671,
https://doi.org/10.1145/3460418.3480399.
F. Adib, C.-Y. Hsu, H. Mao, D. Katabi, and F. Durand, “Capturing
the human figure through a wall,” ACM Transactions on Graphics
(TOG), vol. 34, no. 6, pp. 1–13, 2015. https://doi.org/10.1145/2816795.
H. Bedri, O. Gupta, A. Temme, M. Feigin, G. Charvat, and R. Raskar,
“Rflow: User interaction beyond walls,” in Adjunct Proceedings of the
th Annual ACM Symposium on User Interface Software & Technology, pp. 45–46, 2015. https://doi.org/10.1145/2815585.2817801.
C. Xu, B. Firner, Y. Zhang, R. Howard, J. Li, and X. Lin, “Improving rfbased device-free passive localization in cluttered indoor environments
through probabilistic classification methods,” in Proceedings of the
th international conference on Information Processing in Sensor Networks, pp. 209–220, 2012. https://doi.org/10.1145/2185677.2185734.
J. Cecílio, K. Duarte, P. Martins, and P. Furtado, “Robustpathfinder:
handling uncertainty in indoor positioning techniques,” Procedia computer science, vol. 130, pp. 408–415, 2018. https://doi.org/10.1016/j.
procs.2018.04.061.
J. Wagner, P. Mazurek, A. Mi˛ekina, and R. Z. Morawski, “Regularised
differentiation of measurement data in systems for monitoring of human
movements,” Biomedical Signal Processing and Control, vol. 43,
pp. 265–277, 2018. https://doi.org/10.1016/j.bspc.2018.02.010.
N. Suzuki and H. Matsuno, “Radio wave environment analysis at different locations based on frequent pattern mining,” Procedia computer
science, vol. 112, pp. 1396–1403, 2017. https://doi.org/10.1016/j.procs.
08.061.
A. Hugeat, J. Bernard, G. Goavec-Mérou, P.-Y. Bourgeois, and J.-M.
Friedt, “Filter optimization for real-time digital processing of radio
frequency signals: Application to oscillator metrology,” IEEE Transactions on Ultrasonics, Ferroelectrics, and Frequency Control, vol. 67,
no. 2, pp. 440–449, 2019. https://doi.org/10.1109/tuffc.2019.2945013.
E. Balestrieri, L. De Vito, F. Picariello, and I. Tudosa, “A method
exploiting compressive sampling for localization of radio frequency
emitters,” IEEE Transactions on Instrumentation and Measurement,
vol. 69, no. 5, pp. 2325–2334, 2019. https://doi.org/10.1109/tim.2019.
T. Ma, Z. Chen, J. Wu, W. Zheng, S. Wang, N. Qi, M. Lin, and B. Chi,
“A 77 ghz fmcw mimo radar system based on 65nm cmos cascadable
t3r transceiver,” Science China. Information Sciences, vol. 64, no. 1,
p. 114301, 2021. https://doi.org/10.1007/s11432-019-1511-5.
X. Wang, Y. Zhang, H. Zhang, Y. Li, and X. Wei, “Radio frequency
signal identification using transfer learning based on lstm,” Circuits,
Systems, and Signal Processing, vol. 39, pp. 5514–5528, 2020. https:
//doi.org/10.1007/s00034-020-01417-7.
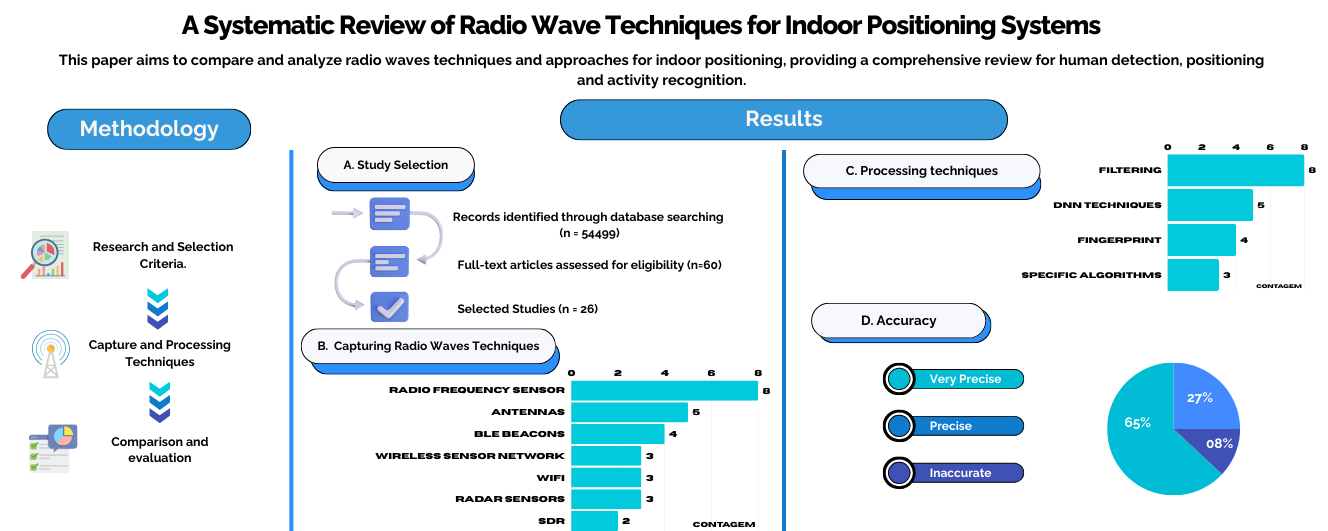