CPP-UNet: Combined Pyramid Pooling Modules in the U-Net Network for Kidney, Tumor and Cyst Segmentation
Keywords:
Pyramid Pooling Module, Segmentation Renal Diseases, Kidney Cancer, U-NetAbstract
Renal carcinoma stands prominently as a significant contributor to global cancer-related mortality rates, highlighting the critical importance of early detection and diagnosis in the management of this ailment. Moreover, the rising incidence of kidney tumors poses a challenge in differentiating between malignant and benign lesions using radiographic methods. Therefore, we present CPP-UNet, an innovative convolutional neural network-based architecture designed for the segmentation of renal structures, including the kidneys themselves and renal masses (cysts and tumors), in a computed tomography (CT) scan. Particularly, we investigate the fusion of the Pyramid Pooling Module (PPM) and Atrous Spatial Pyramid Pooling (ASPP) for improving the UNet network by integrating contextual information across multiple scales. Our proposed method yielded promising outcomes in the Kidney and Kidney Tumor Segmentation challenge (KiTS21 and KiTS23) datasets, exhibiting Dice indices of 93.51% and 92.84% for Kidneys and Masses, 90.33% and 92.08% for Renal Masses, and 85.69% and 88.17% for Tumors, respectively.
Downloads
References
A. L. Ammirati, “Chronic kidney disease,” Revista da Associação
Médica Brasileira, vol. 66, pp. s03–s09, 2020. DOI:https://doi.org/10.
/1806-9282.66.S1.3.
A. Chang, A. Finelli, J. S. Berns, and M. Rosner, “Chronic kidney
disease in patients with renal cell carcinoma,” Advances in chronic
kidney disease, vol. 21, no. 1, pp. 91–95, 2014. DOI:https://doi.org/
1053/j.ackd.2013.09.003.
T. Gansler, S. Fedewa, M. B. Amin, C. C. Lin, and A. Jemal, “Trends
in reporting histological subtyping of renal cell carcinoma: association
with cancer center type,” Human pathology, vol. 74, pp. 99–108, 2018.
DOI:https://doi.org/10.1016/j.humpath.2018.01.010.
R. Siegel, J. Ma, Z. Zou, and A. Jemal, “Cancer statistics, 2014.,” CA: a
cancer journal for clinicians, vol. 64, no. 1, pp. 9–29, 2014. DOI:https:
//doi.org/10.3322/caac.21208.
Q. Cai, Y. Chen, X. Qi, D. Zhang, J. Pan, Z. Xie, C. Xu, S. Li, X. Zhang,
Y. Gao, et al., “Temporal trends of kidney cancer incidence and mortality
from 1990 to 2016 and projections to 2030,” Translational Andrology
and Urology, vol. 9, no. 2, p. 166, 2020. DOI:https://doi.org/10.21037/
tau.2020.02.23.
X. Bai, M. Yi, B. Dong, X. Zheng, and K. Wu, “The global, regional,
and national burden of kidney cancer and attributable risk factor analysis
from 1990 to 2017,” Experimental Hematology & Oncology, vol. 9,
pp. 1–15, 2020. DOI:https://doi.org/10.1186/s40164-020-00181-3.
R. L. Siegel, A. N. Giaquinto, and A. Jemal, “Cancer statistics, 2024,”
CA: A Cancer Journal for Clinicians, 2024. DOI:https://doi.org/10.3322/
caac.21820.
American Cancer Society, “Kidney cancer early detection, diagnosis,
and staging.” Available online: https://www.cancer.org/content/dam/
CRC/PDF/Public/8661.00.pdf (accessed on 06 February 2024), February
E. Bercovich and M. C. Javitt, “Medical imaging: from roentgen to the
digital revolution, and beyond,” Rambam Maimonides medical journal,
vol. 9, no. 4, 2018. DOI:https://doi.org/10.5041/RMMJ.10355.
Z. Zhao, H. Chen, and L. Wang, “A coarse-to-fine framework for the
kidney and kidney tumor segmentation challenge,” in Kidney and
Kidney Tumor Segmentation: MICCAI 2021 Challenge, KiTS 2021, Held
in Conjunction with MICCAI 2021, Strasbourg, France, September 27,
, Proceedings, pp. 53–58, Springer, 2022. DOI:https://doi.org/10.
/978-3-030-98385-7_8.
A. Golts, D. Khapun, D. Shats, Y. Shoshan, and F. Gilboa-Solomon,
“An ensemble of 3d u-net based models for segmentation of kidney
and masses in ct scans,” in Kidney and Kidney Tumor Segmentation:
MICCAI 2021 Challenge, KiTS 2021, Held in Conjunction with MICCAI
, Strasbourg, France, September 27, 2021, Proceedings, pp. 103–
, Springer, 2022. DOI:https://doi.org/10.1007/978-3-030-98385-7_
Y. George, “A coarse-to-fine 3d u-net network for semantic segmentation
of kidney ct scans,” in Kidney and Kidney Tumor Segmentation: MICCAI
Challenge, KiTS 2021, Held in Conjunction with MICCAI 2021,
Strasbourg, France, September 27, 2021, Proceedings, pp. 137–142,
Springer, 2022. DOI:https://doi.org/10.1007/978-3-030-98385-7_18.
X. Yang, J. Zhang, J. Zhang, and Y. Xia, “Transfer learning for
kits21 challenge,” in Kidney and Kidney Tumor Segmentation: MICCAI
Challenge, KiTS 2021, Held in Conjunction with MICCAI 2021,
Strasbourg, France, September 27, 2021, Proceedings, pp. 158–163,
Springer, 2022. DOI:https://doi.org/10.1007/978-3-030-98385-7_21.
P. Sun, Z. Mo, F. Hu, X. Song, T. Mo, B. Yu, Y. Zhang, and
Z. Chen, “Segmentation of kidney mass using agdenseu-net 2.5 d
model,” Computers in Biology and Medicine, vol. 150, p. 106223, 2022.
DOI:https://doi.org/10.1016/j.compbiomed.2022.106223.
M. Wu and Z. Liu, “Less is more: Contrast attention assisted unet
for kidney, tumor and cyst segmentations,” in Kidney and Kidney
Tumor Segmentation: MICCAI 2021 Challenge, KiTS 2021, Held in
Conjunction with MICCAI 2021, Strasbourg, France, September 27,
, Proceedings, pp. 46–52, Springer, 2022. DOI:https://doi.org/10.
/978-3-030-98385-7_7.
K.-H. Uhm, H. Cho, Z. Xu, S. Lim, S.-W. Jung, S.-H. Hong, and S.-
J. Ko, “Exploring 3d u-net training configurations and post-processing
strategies for the miccai 2023 kidney and tumor segmentation challenge,”
arXiv preprint arXiv:2312.05528, 2023. DOI:https://doi.org/10.48550/
arXiv.2312.05528.
A. Myronenko, D. Yang, Y. He, and D. Xu, “Automated 3d segmentation
of kidneys and tumors in miccai kits 2023 challenge,” arXiv
preprint arXiv:2310.04110, 2023. DOI:https://doi.org/10.48550/arXiv.
04110.
G. Stoica, M. Breaban, and V. Barbu, “Analyzing domain shift
when using additional data for the miccai kits23 challenge,” arXiv
preprint arXiv:2309.02001, 2023. DOI:https://doi.org/10.48550/arXiv.
02001.
S. Zheng, Q. Sun, X. Ye, W. Li, L. Yu, and C. Yang, “Multi-scale
adversarial learning with difficult region supervision learning models for
primary tumor segmentation,” Physics in Medicine & Biology, vol. 69,
no. 8, p. 085009, 2024. DOI:https://doi.org/10.1088/1361-6560/ad3321.
N. Heller, N. Sathianathen, A. Kalapara, E. Walczak, K. Moore,
H. Kaluzniak, J. Rosenberg, P. Blake, Z. Rengel, M. Oestreich, et al.,
“The 2021 kidney and kidney tumor segmentation challenge.” Available
online: https://kits21.kits-challenge.org/ (accessed on 06 January 2024),
S. Samuel, M. Moore, B. Patterson, H. Sheridan, and C. Sorensen,
“Neuroimaging data primer: A resource for curating digital imaging
and communications in medicine (dicom) and neuroimaging informatics
technology initiative (nifti) files,” 2020. DOI:https://doi.org/10.7302/
S. M. Pizer, E. P. Amburn, J. D. Austin, R. Cromartie, A. Geselowitz,
T. Greer, B. ter Haar Romeny, J. B. Zimmerman, and K. Zuiderveld,
“Adaptive histogram equalization and its variations,” Computer vision,
graphics, and image processing, vol. 39, no. 3, pp. 355–368, 1987.
DOI:https://doi.org/10.1016/S0734-189X(87)80186-X.
L.-C. Chen, Y. Zhu, G. Papandreou, F. Schroff, and H. Adam, “Encoderdecoder
with atrous separable convolution for semantic image segmentation,”
in Proceedings of the European conference on computer vision
(ECCV), pp. 801–818, 2018. DOI:https://doi.org/10.48550/arXiv.1802.
M. Bland, An Introduction to Medical Statistics. OUP Oxford, 2015.
ISBN: 9780191002991.
A. A. Taha and A. Hanbury, “Metrics for evaluating 3d medical
image segmentation: analysis, selection, and tool,” BMC medical imaging,
vol. 15, no. 1, pp. 1–28, 2015. DOI:https://doi.org/10.1186/
s12880-015-0068-x.
T. Eelbode, J. Bertels, M. Berman, D. Vandermeulen, F. Maes, R. Bisschops,
and M. B. Blaschko, “Optimization for medical image segmentation:
theory and practice when evaluating with dice score or
jaccard index,” IEEE Transactions on Medical Imaging, vol. 39, no. 11,
pp. 3679–3690, 2020. DOI:https://doi.org/10.1109/TMI.2020.3002417.
C. E. F. Matos, M. V. S. L. Oliveira, J. O. B. Diniz, A. G. S. Fernandes,
G. B. Junior, and A. C. de Paiva, “Ppm-deeplab: Módulo de pirâmide
de pooling como codificador da rede deeplabv3+ para segmentaçao de
rins, cistos e tumores renais,” in Anais do XXIII Simpósio Brasileiro de
Computação Aplicada à Saúde, pp. 210–221, SBC, 2023. DOI:https:
//doi.org/10.5753/sbcas.2023.229611.
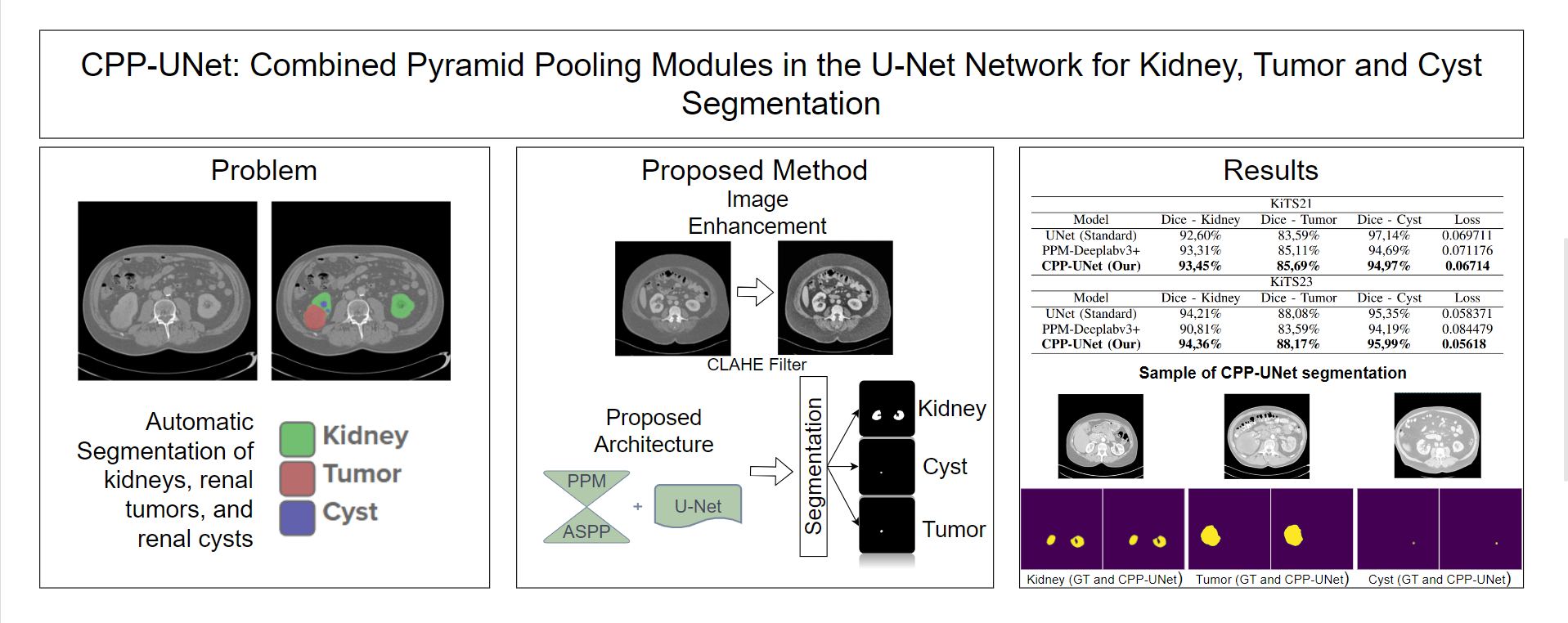