Exploring COVID-19 Trends in Mexico during the Winter Season with Explainable Artificial Intelligence (XAI)
Keywords:
Explainable Artificial Intelligence, XAI, Interpretable Random Forest, COVID-19, winter season, MexicoAbstract
COVID-19 has become the most significant pandemic in recent years. Today, Mexico has recorded millions of infections and deaths since the pandemic started. Around the world, machine learning methods have been used to understand, predict or develop strategies to manage the virus and the pandemic. Although algorithms provide good results, it is necessary to understand why a model makes specific predictions with a particular data set. To explain this question, we apply Explainable Artificial Intelligence (XAI) in this paper. With this, it is possible to understand the characteristics that influence the model decisions when denoting between deaths and survivors. As a case of study, the positive cases detected during the winter season of 2020-2021 and 2021-2022 were considered. In this season, respiratory diseases increased considerably, and in the study period, they influenced the increase in positive cases and the spread of COVID-19. Preliminary results suggest that age is essential when using a Random Forest model. Preliminary results suggest that age is essential when determining the prognosis of a patient infected by COVID-19 in winter seasons.
Downloads
References
R. F. Albuquerque Paiva de Oliveira, C. J. A. Bastos Filho, A. C. A. M. V. F. de Medeiros, P. J. Buarque Lins dos Santos, and D. Lopes Freire, “Machine learning applied in sars-cov-2 covid 19 screening using clinical analysis parameters,” IEEE Lat. Am. Trans., vol. 19, pp. 978-985, Jun. 2021.
C. Noak and S. Wang, “Analysis of covid-19 data using advanced machine learning models,” in 2022 3rd PRML, (Chengdu, China), pp. 430–437, 2022.
S. Tiwari, P. Chanak, and S. K. Singh, “A Review of the Machine Learning algorithms for Covid-19 Case Analysis,” IEEE Trans. Artif. Intell., vol. 4, no. 1, pp. 44–59, 2023.
N. Alballa and I. Al-Turaiki, “Machine learning approaches in COVID-19 diagnosis, mortality, and severity risk prediction: A review,” Informatics in Medicine Unlocked, vol. 24, p. 100564, 2021.
Y. V. Kistenev, D. A. Vrazhnov, E. E. Shnaider, and H. Zuhayri, “Predictive models for COVID-19 detection using routine blood tests and machine learning,” Heliyon, vol. 8, no. 10, p. e11185, 2022.
V. Paramarta, A. Mailangkay, H. Amalia, and D. Chrismas, “Public sentiment analysis of indonesian tweets about covid-19 vaccination using different machine learning approaches,” in 2022 Seventh ICIC, (Bali, Indonesia), pp. 1–5, 2022.
D. García-Gil, F. Luque-Sánchez, J. Luengo, S. García, and F. Herrera, “From Big to Smart Data: Iterative ensemble filter for noise filtering in
big data classification,” Int. J. Intell. Syst., vol. 34, no. 12, pp. 3260–3274, 2019.
I. Rodrigues, G. L. Santos, D. F. Sadok, and P. T. Endo, “Classifying COVID-19 positive X-ray using deep learning models,” IEEE Lat. Am. Trans., vol. 19, pp. 884—-892, Jun. 2021.
E. Cortés and S. Sánchez, “Deep Learning Transfer with AlexNet for chest X-ray COVID-19 recognition,” IEEE Lat. Am. Trans., vol. 19,
pp. 944-951, Jun. 2021.
A. D. Orjuela-Cañon and O. Perdomo, “Clustering Proposal Support for the COVID-19 Making Decision Process in a Data Demanding Scenario,” IEEE Lat. Am. Trans., vol. 19, pp. 1041—-1049, Jun. 2021.
D. C. d. S. Gomes and G. L. d. O. Serra, “Machine Learning Model
for Computational Tracking and Forecasting the COVID-19 Dynamic
Propagation,” IEEE J. Biomed. Health Inform., vol. 25, no. 3, pp. 615–
, 2021.
S. Ghafouri-Fard, H. Mohammad-Rahimi, P. Motie, M. A. Minabi, M. Taheri, and S. Nateghinia, “Application of machine learning in the
prediction of COVID-19 daily new cases: A scoping review,” Heliyon, vol. 7, no. 10, p. e08143, 2021.
F. Giuste, W. Shi, Y. Zhu, T. Naren, M. Isgut, Y. Sha, L. Tong, M. Gupte, and M. D. Wang, “Explainable Artificial Intelligence Methods in Combating Pandemics: A Systematic Review,” IEEE Rev. Biomed. Eng., vol. 16, pp. 5–21, 2023.
I. Ahmed, G. Jeon, and F. Piccialli, “From artificial intelligence to explainable artificial intelligence in industry 4.0: A survey on what, how,
and where,” IEEE Trans. Ind. Inform., vol. 18, no. 8, pp. 5031–5042, 2022.
S. M. Lundberg, G. Erion, H. Chen, A. DeGrave, J. M. Prutkin, B. Nair, R. Katz, J. Himmelfarb, N. Bansal, and S.-I. Lee, “From local
explanations to global understanding with explainable ai for trees,” Nat. Mach. Intell., vol. 2, no. 1, pp. 56–67, 2020.
C. E. Kistler, R. L. Jump, P. D. Sloane, and S. Zimmerman, “The Winter Respiratory Viral Season During the COVID-19 Pandemic,” J. Am. Med. Dir. Assoc., vol. 21, no. 12, pp. 1741–1745, 2020.
K. K. Kırboga, E. U. Kü.üksille, M. E. Naldan, M. I.sık, O. Gülcü, and E. Aksakal, “CVD22: Explainable artificial intelligence determination of the relationship of troponin to D-Dimer, mortality, and CK-MB in COVID-19 patients,” Comput. Methods Programs Biomed., vol. 233, p. 107492, 2023.
Y. Chen, R. Calabrese, and B. Martin-Barragan, “Interpretable machine learning for imbalanced credit scoring datasets,” Eur. J. Oper. Res., 2023.
J. H. Ong, K. M. Goh, and L. L. Lim, “Comparative analysis of explainable artificial intelligence for covid-19 diagnosis on cxr image,”
in 2021 IEEE ICSIPA, (Kuala Terengganu, Malaysia), pp. 185–190, 2021.
T. Chauhan and S. Sonawane, “Explicable ai for surveillance and interpretation of coronavirus using x-ray imaging,” in 2023 ESCI, (Pune, India), pp. 1–6, 2023.
M. N. S. Choudary, V. B. Bommineni, G. Tarun, G. P. Reddy, and G. Gopakumar, “Predicting Covid-19 Positive Cases and Analysis on the Relevance of Features using SHAP (SHapley Additive exPlanation),” in 2021 Second ICESC, (Coimbatore, India), pp. 1892–1896, 2021.
H. Wu, W. Ruan, J. Wang, D. Zheng, B. Liu, Y. Geng, X. Chai, J. Chen, K. Li, S. Li, and S. Helal, “Interpretable Machine Learning for COVID-19: An Empirical Study on Severity Prediction Task,” IEEE Trans. Artif. Intell., vol. 4, no. 4, pp. 764–777, 2023.
S. Dahal, R. Luo, M. H. Swahn, and G. Chowell, “Geospatial Variability in excess death rates during the COVID-19 pandemic in Mexico:
Examining Socio Demographic, Climate and Population Health Characteristics,” Int. Jour. Infec. Diseases, vol. 113, pp. 347–354, 2021.
P. Liu, A. Blet, D. Smyth, and H. Li, “The Science Underlying COVID-19 Implications for the Cardiovascular System,” Circulation, vol. 142, no. 1, pp. 68–78, 2020.
J. Tian, X. Yuan, J. Xiao, Q. Zhong, C. Yang, B. Liu, Y. Cai, Z. Lu, J. Wang, Y. Wang, S. Liu, B. Cheng, J. Wang, M. Zhang, L. Wang,
S. Niu, Z. Yao, X. Deng, F. Zhou, W. Wei, Q. Li, X. Chen, W. Chen, Q. Yang, S. Wu, J. Fan, B. Shu, Z. Hu, S. Wang, X. Yang, W. Liu,
X. Miao, and Z. Wang, “Clinical characteristics and risk factors associated with COVID-19 disease severity in patients with cancer in Wuhan,
China: a multicentre, retrospective, cohort study,” Lancet Oncol., vol. 21, no. 7, pp. 893–903, 2020.
A. Luque, A. Carrasco, A. Martín, and A. de las Heras, “The impact of class imbalance in classification performance metrics based on the
binary confusion matrix,” Pattern Recognition, vol. 91, pp. 216–231,2019.
L. Buitinck, G. Louppe, M. Blondel, F. Pedregosa, A. Mueller, O. Grisel, V. Niculae, P. Prettenhofer, A. Gramfort, J. Grobler, R. Layton, J. VanderPlas, A. Joly, B. Holt, and G. Varoquaux, “API design for machine learning software: experiences from the scikit-learn project,” in ECML
PKDD Workshop: Languages for Data Mining and Machine Learning, (Praga,Czech Republic), pp. 108–122, 2013.
C. Sammut and G. I. Webb, eds., Random Forests, pp. 1054–1054. Boston, MA: Springer US, 2017.
Q. Kang, X. Chen, S. Li, and M. Zhou, “A noise-filtered under-sampling scheme for imbalanced classification,” IEEE Trans. on Cybernetics, vol. 47, no. 12, pp. 4263–4274, 2017.
J. Deng, J. Sun, W. Peng, D. Zhang, and V. Vyatkin, “Imbalanced multiclass classification with active learning in strip rolling process,”
Knowl.-Based Syst., vol. 255, p. 109754, 2022.
M. E. Camacho Moll, A. M. Salinas Martínez, B. Tovar Cisneros, J. I. García Onofre, G. Navarrete Floriano, and M. Bermúdez de León,
“Extension and Severity of Self-Reported Side Effects of Seven COVID-19 Vaccines in Mexican Population,” Front. Public Health, vol. 10, 2022.
O. Y. Bello-Chavolla, N. Antonio-Villa, S. Valdés-Ferrer, C. Fermín- Martínez, L. Fernández-Chirino, D. Ramírez-García, J. Mancilla- Galindo, A. Kammar-García, J. Ávila Funes, C. H. Zuñiga-Gil, M. García-Grimshaw, S. E. Ceballos-Liceaga, G. Carbajal-Sandoval,
J. A. Montes-González, C. Zaragoza-Jiménez, G. Garcia-Rodriguez, R. Cortes-Alcala, G. Reyes-Terón, H. Lopez-Gatell, and L. Gutiérrez- Robledo, “Effectiveness of a nationwide covid-19 vaccination program in mexico against symptomatic covid-19, hospitalizations, and death:
a retrospective analysis of national surveillance data,” International Journal of Infectious Diseases, vol. 129, pp. 188–196, 2022.
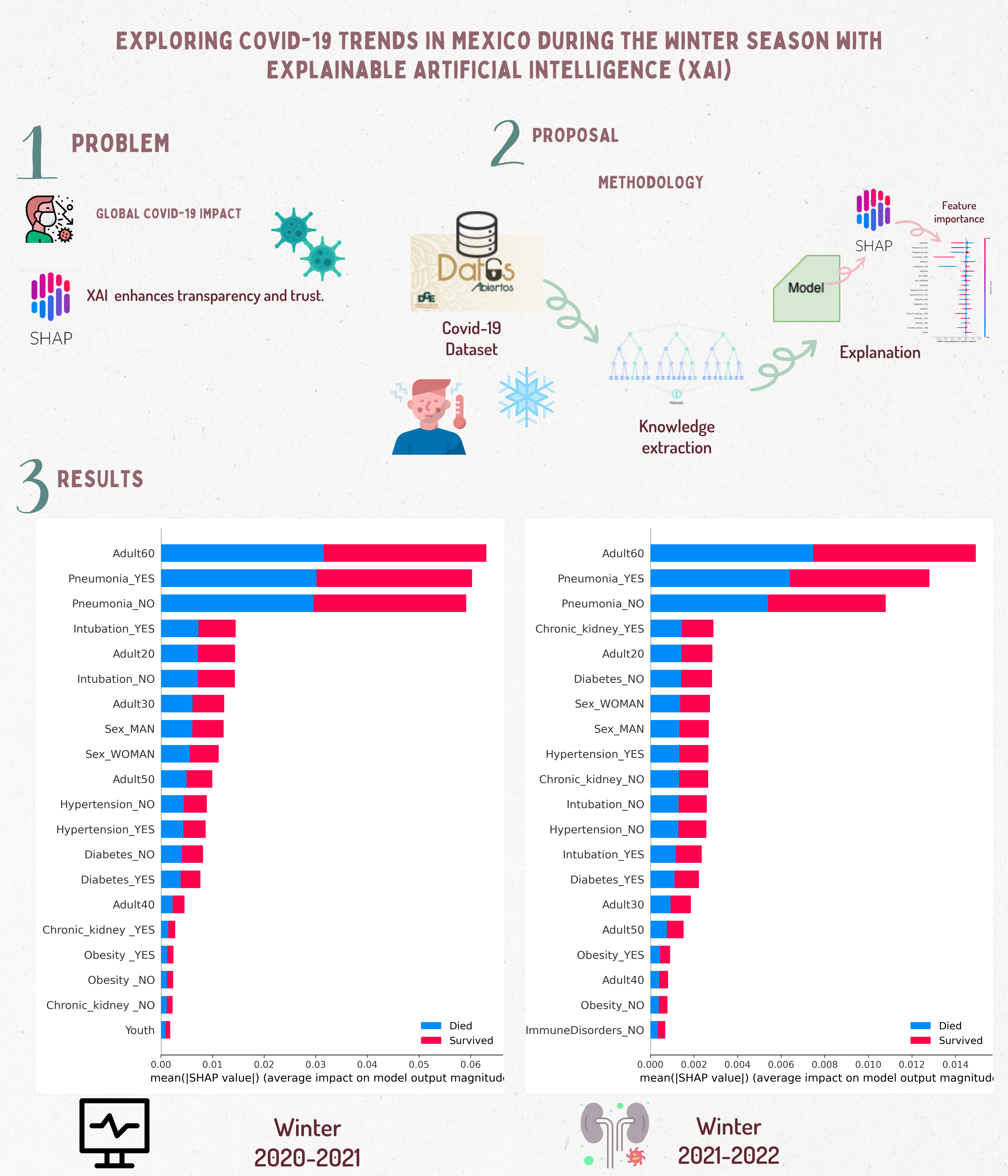