Hierarchical Short Term Load Forecasting Considering Weighting by Meteorological Region
Keywords:
Artificial Neural Network, Hierarchical Short Term Load Forecasting, Multi Region Forecasting, Meteorological variables weightingAbstract
Activities related to the planning and operation of power systems use as premise the load forecasting, which is responsible to provide a load estimative for a given horizon that assists mainly in the electroenergetic operation of a power system. The hierarchical short-term load forecasting becomes an approach used for this purpose, where the overall forecast is performed through system partition in smaller macro regions, and soon after, is aggregated to compose a global forecast. Then, this paper presents a hierarchical short-term forecasting approach for macro-regions, with the main contribution being the proposal of an indicator that represents the Average Consumption per Meteorological Region (CERM), to be used as weighting of each Meteorological Station (EM) as their importance for the total demand of the macro-region. This indicator is used to weight the temperature variable and then, is incorporated into a Multi-layer perceptron ANN model for the load forecasting on the horizon of 7 days ahead with hourly and daily discretization. The results showed higher average performance of the variable CERM in relation to the other combination performed, and the best results were used to compose the prediction of the Multi-Region (MTR). Finally, the proposed model presented a superior performance compared to an basis aggregate model for MTR, which shows the efficiency of the proposed methodology.
Downloads
References
A. M. Tudose, I. I. Picioroaga, D. O. Sidea, C. Bulac, and V. A. Boicea, “Short-Term Load Forecasting Using Convolutional Neural Networks in COVID-19 Context: The Romanian Case Study,” Energies, vol. 14, no. 13, p. 4046, Jul. 2021.
Y. Wi, S. Joo, and K. Song, “Holiday Load Forecasting Using Fuzzy Polynomial Regression With Weather Feature Selection and Adjustment,” IEEE Trans. Power Syst., vol. 27, no. 2, pp. 596–603, May 2012.
M. Q. Raza and A. Khosravi, “A review on artificial intelligence based load demand forecasting techniques for smart grid and buildings,” Renew. Sustain. Energy Rev., vol. 50, pp. 1352–1372, Oct. 2015.
V. Veeramsetty, D. R. Chandra, and S. R. Salkuti, “Short‐term electric power load forecasting using factor analysis and long short‐term memory for smart cities,” Int. J. Circuit Theory Appl., vol. 49, no. 6, pp. 1678–1703, Jun. 2021.
S. N. Fallah, M. Ganjkhani, S. Shamshirband, and K. wing Chau, “Computational intelligence on short-term load forecasting: A methodological overview,” Energies, vol. 12, no. 3, 2019.
X. Sun et al., “An Efficient Approach to Short-Term Load Forecasting at the Distribution Level,” pp. 1–12, 2015.
Y. Sakuma and H. Nishi, “Hierarchical Multiobjective Distributed Deep Learning for Residential Short-Term Electric Load Forecasting,” IEEE Access, vol. 10, no. June, pp. 69950–69962, 2022.
B. Auder, J. Cugliari, Y. Goude, and J.-M. Poggi, “Scalable Clustering of Individual Electrical Curves for Profiling and Bottom-Up Forecasting,” Energies, vol. 11, no. 7, p. 1893, Jul. 2018.
L. N. Silva, A. R. Abaide, V. G. Negri, and A. G. Dornelles, “Proposal for the Study of Short-Term Load Forecasting Multi-Regional Error Impact in the Operation Planning,” Proc. - 2018 53rd Int. Univ. Power Eng. Conf. UPEC 2018, pp. 1–6, 2018.
P. Lusis, K. R. Khalilpour, L. Andrew, and A. Liebman, “Short-term residential load forecasting: Impact of calendar effects and forecast granularity,” Appl. Energy, vol. 205, no. July, pp. 654–669, 2017.
J. Vaish, S. S. Datta, and K. Seethalekshmi, “Short Term Load Forecasting using ANN and Ensemble Models Considering Solar Irradiance,” in 2020 International Conference on Electrical and Electronics Engineering (ICE3), 2020, no. i, pp. 44–48.
K. Shahare, A. Mitra, D. Naware, R. Keshri, and H. M. Suryawanshi, “Performance analysis and comparison of various techniques for short-term load forecasting,” Energy Reports, vol. 9, pp. 799–808, Mar. 2023.
A. Román-Portabales, M. López-Nores, and J. J. Pazos-Arias, “Systematic Review of Electricity Demand Forecast Using ANN-Based Machine Learning Algorithms,” Sensors, vol. 21, no. 13, p. 4544, Jul. 2021.
C. L. P. Pennings and J. van Dalen, “Integrated hierarchical forecasting,” Eur. J. Oper. Res., vol. 263, no. 2, pp. 412–418, Dec. 2017.
T. Hong, “Load Forecasting Case Study,” 2015.
Shu Fan, K. Methaprayoon, and Wei-Jen Lee, “Multiregion Load Forecasting for System With Large Geographical Area,” IEEE Trans. Ind. Appl., vol. 45, no. 4, pp. 1452–1459, Jul. 2009.
W.-C. Chu, Y.-P. Chen, Z.-W. Xu, and W.-J. Lee, “Multiregion Short-Term Load Forecasting in Consideration of HI and Load/Weather Diversity,” IEEE Trans. Ind. Appl., vol. 47, no. 1, pp. 232–237, Jan. 2011.
T. Hong, P. Wang, and L. White, “Weather station selection for electric load forecasting,” Int. J. Forecast., vol. 31, no. 2, pp. 286–295, Apr. 2015.
L. N. Silva, A. R. Abaide, I. C. Figueiro, J. O. Silva, J. Rigodanzo, and J. P. Sausen, “Development of Brazilian multi region short-term load forecasting model considering climate variables weighting in ANN model,” in 2017 52nd International Universities Power Engineering Conference (UPEC), 2017, pp. 1–6.
M. Brégère and M. Huard, “Online hierarchical forecasting for power consumption data,” Int. J. Forecast., vol. 38, no. 1, pp. 339–351, Jan. 2022.
S. Fan, K. Methaprayoon, and W. J. Lee, “Short-term Multi-Region Load Forecasting Based on Weather and Load Diversity Analysis,” in 2007 39th North American Power Symposium, 2007, pp. 562–567.
S. Fan, Y. Wu, W.-J. Lee, and C. Lee, “Comparative study on load forecasting technologies for different geographical distributed loads,” in 2011 IEEE Power and Energy Society General Meeting, 2011, pp. 1–8.
T. Hong and S. Lai, “When One Size No Longer Fits All – Electric Load Forecasting with a Geographic Hierarchy,” 2013.
T. Hong, P. Wang, and L. White, “Weather station selection for electric load forecasting,” Int. J. Forecast., vol. 31, no. 2, pp. 286–295, 2015.
L. N. Silva, A. R. Abaide, I. C. Figueiro, J. O. Silva, J. Rigodanzo, and J. P. Sausen, “Development of Brazilian multi region short-term load forecasting model considering climate variables weighting in ANN model,” in 2017 52nd International Universities Power Engineering Conference (UPEC), 2017, vol. 2017-Janua, pp. 1–6.
Ministério de Minas e Energia, “Anuário Estatístico de Energia Elétrica 2016.” 2016.
S. Fallah, M. Ganjkhani, S. Shamshirband, and K. Chau, “Computational Intelligence on Short-Term Load Forecasting: A Methodological Overview,” Energies, vol. 12, no. 3, p. 393, Jan. 2019.
R. Mamlook, O. Badran, and E. Abdulhadi, “A fuzzy inference model for short-term load forecasting,” Energy Policy, vol. 37, no. 4, pp. 1239–1248, Apr. 2009.
L. Suganthi and A. a. Samuel, “Energy models for demand forecasting—A review,” Renew. Sustain. Energy Rev., vol. 16, no. 2, pp. 1223–1240, Feb. 2012.
K. Zor, O. Timur, and A. Teke, “A state-of-the-art review of artificial intelligence techniques for short-term electric load forecasting,” in 2017 6th International Youth Conference on Energy (IYCE), 2017, pp. 1–7.
T. Ahmad and H. Chen, “Deep learning for multi-scale smart energy forecasting,” Energy, 2019.
S. Haykin, Redes neurais artificiais: princípios e prática, 2a Edição. Porto Alegre: Tradução Paulo Martins Engel., 2001.
M. Salgado, “Sistema de Suporte à Decisão para Análise e Previsão de Carga por Barramento,” Universidade Estadual de Campinas, 2009.
I. Koprinska, M. Rana, and V. G. Agelidis, “Correlation and instance based feature selection for electricity load forecasting,” Knowledge-Based Syst., vol. 82, pp. 29–40, Jul. 2015.
INMET, “Instituto Nacional de Meteorologia,” 2017. [Online]. Available: http://www.inmet.gov.br/portal/index.php?r=estacoes/estacoesautomaticas.
L. N. Silva, A. R. Abaide, V. G. Negri, M. Capeletti, and L. F. Lopes, “Impact Evaluation of Feature Selection to Short-Term Load Forecasting Models considering Weather Inputs and Load History,” in 2019 54th International Universities Power Engineering Conference (UPEC), 2019, pp. 1–6
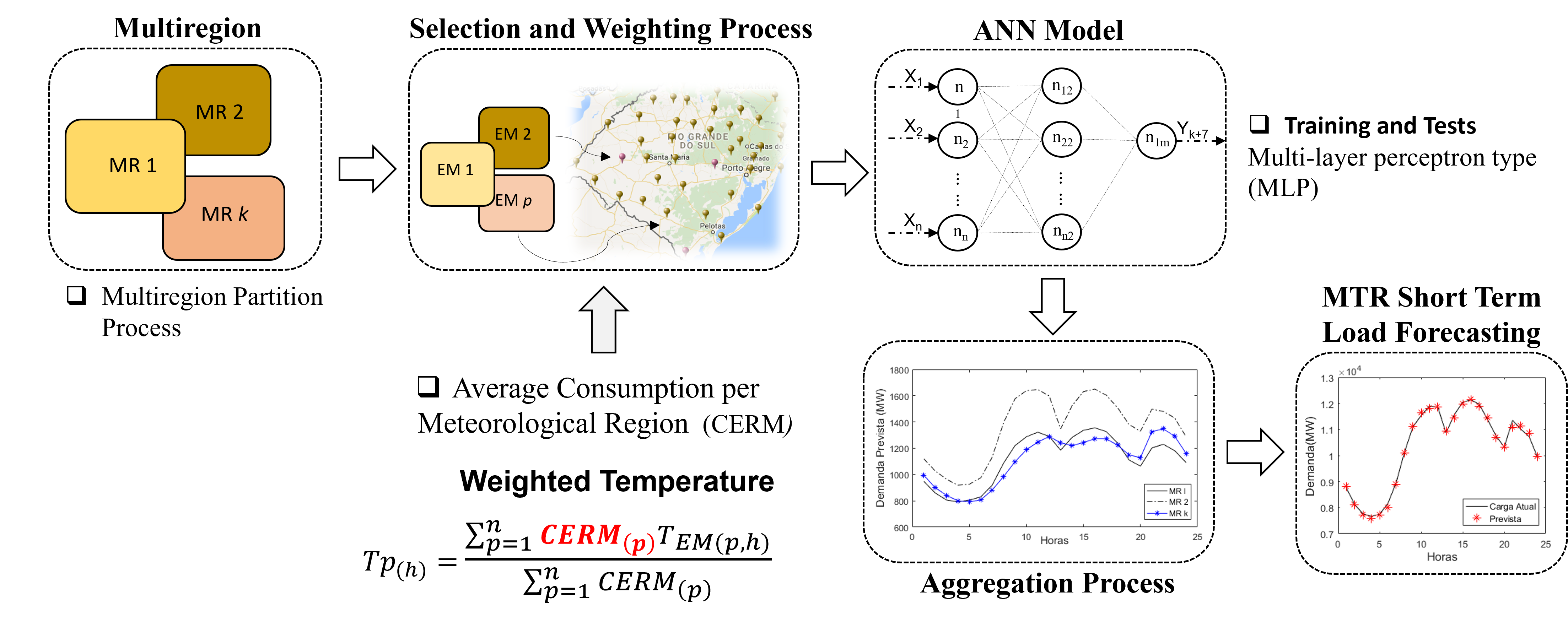