Combining ArcFace and Visual Transformer Mechanisms for Biometric Periocular Recognition
Keywords:
biometrics, ocular recognition, periocular recognition, attention, visual transformers, arcfaceAbstract
In the last decades, advances in Biometrics have resulted in the popularization of biometric identification applications in different scenarios. However, biometric recognition techniques can exhibit sub-par performance in undesirable or restricted scenarios. Therefore, there is still a need to investigate better recognition techniques and more appropriate biometric traits. Studies have shown that attention is an important mechanism present in biological vision systems, including the human vision system, that can improve significantly the correct recognition rates in computer vision systems. Studies have also shown that periocular characteristics suffer less from environmental changes than faces in undesirable scenarios, achieving similar performance using only 25% of all the data in the face. Motivated by these findings, this paper proposes a new method for periocular recognition based on attention mechanisms that incorporates a recent ViT architecture together with the ArcFace loss function. Experimental results obtained on UBIPr and FRGC, two popular datasets, showed that the proposed method obtained lower error rates when compared to other state-of-the-art periocular recognition methods, in addition to being able to provide the visualization of attention weights for a better understanding of the most important periocular regions used by the neural network for biometric recognition.
Downloads
References
A. K. Jain, A. A. Ross, and K. Nandakumar, Introduction to biometrics. New York, NY: Springer Science & Business Media, Nov. 2011.
G. Guo and N. Zhang, “A survey on deep learning based face recognition,” Computer Vision and Image Understanding, vol. 189, p. 102805, 2019.
P. J. Phillips, P. J. Flynn, T. Scruggs, K. W. Bowyer, and W. Worek, “Preliminary face recognition grand challenge results,” in 7th International Conference on Automatic Face and Gesture Recognition (FGR06), (Southampton, UK), pp. 15–24, IEEE, 2006.
N. K. Benamara, E. Zigh, T. B. Stambouli, and M. Keche, “Towards a robust thermal-visible heterogeneous face recognition approach based on a cycle generative adversarial network,” Int. J. Interact. Multimed.Artif. Intell, vol. 7, no. 4, pp. 132–145, 2022.
P. Kumari and K. Seeja, “Periocular biometrics: A survey,” Journal of King Saud University - Computer and Information Sciences, vol. 34, no. 4, pp. 1086–1097, 2022.
L. A. Zanlorensi, R. Laroca, E. Luz, A. S. Britto Jr, L. S. Oliveira, and D. Menotti, “Ocular recognition databases and competitions: A survey,” Artificial Intelligence Review, vol. 55, no. 1, pp. 129–180, 2022.
J. Howard, A. Huang, Z. Li, Z. Tufekci, V. Zdimal, H.-M. Van Der Westhuizen, A. Von Delft, A. Price, L. Fridman, L.-H. Tang, et al., “An evidence review of face masks against covid-19,” Proceedings of the National Academy of Sciences, vol. 118, no. 4, p. e2014564118, 2021.
G. Santos and H. Proença, “Periocular biometrics: An emerging technology for unconstrained scenarios,” in 2013 IEEE Symposium on Computational Intelligence in Biometrics and Identity Management (CIBIM), pp. 14–21, 2013.
U. Park, R. R. Jillela, A. Ross, and A. K. Jain, “Periocular biometrics in the visible spectrum,” IEEE Transactions on Information Forensics and Security, vol. 6, no. 1, pp. 96–106, 2011.
J. Xiang and G. Zhu, “Joint face detection and facial expression recognition with mtcnn,” in 2017 4th International Conference on Information Science and Control Engineering (ICISCE), pp. 424–427, IEEE, 2017.
C. N. Padole and H. Proenca, “Periocular recognition: Analysis of performance degradation factors,” in 2012 5th IAPR international conference on biometrics (ICB), pp. 439–445, IEEE, 2012.
J. M. Smereka, B. V. Kumar, and A. Rodriguez, “Selecting discriminative regions for periocular verification,” in 2016 IEEE International Conference on Identity, Security and Behavior Analysis (ISBA), pp. 1–8, IEEE, 2016.
J. M. Smereka and B. V. Kumar, “What is a" good" periocular region for recognition?,” in 2013 IEEE Conference on Computer Vision and Pattern Recognition Workshops, pp. 117–124, IEEE, 2013.
M. V. Vizoni and A. N. Marana, “Ocular recognition using deep features for identity authentication,” in 2020 International Conference on Systems, Signals and Image Processing (IWSSIP), pp. 155–160, 2020.
K. Hernandez-Diaz, F. Alonso-Fernandez, and J. Bigun, “Periocular recognition using cnn features off-the-shelf,” in 2018 International conference of the biometrics special interest group (BIOSIG), pp. 1–5, IEEE, 2018.
R. C. Dalapicola, R. T. V. Queiroga, C. T. Ferraz, T. T. N. Borges, J. H. Saito, and A. Gonzaga, “Impact of facial expressions on the accuracy of a cnn performing periocular recognition,” in 2019 8th Brazilian Conference on Intelligent Systems (BRACIS), pp. 401–406, IEEE, 2019.
Z. Zhao and A. Kumar, “Accurate periocular recognition under less constrained environment using semantics-assisted convolutional neural network,” IEEE Transactions on Information Forensics and Security, vol. 12, no. 5, pp. 1017–1030, 2016.
Z. Zhao and A. Kumar, “Improving periocular recognition by explicit attention to critical regions in deep neural network,” IEEE Transactions on Information Forensics and Security, vol. 13, no. 12, pp. 2937–2952, 2018.
K. K. Kamarajugadda and P. Movva, “Periocular region based biometric identification using sift and surf key point descriptors,” in 2019 IEEE 10th Annual Information Technology, Electronics and Mobile Communication Conference (IEMCON), pp. 0968–0972, IEEE, 2019.
S. Umer, A. Sardar, B. C. Dhara, R. K. Rout, and H. M. Pandey, “Person identification using fusion of iris and periocular deep features,” Neural Networks, vol. 122, pp. 407–419, 2020.
G. Santos, E. Grancho, M. V. Bernardo, and P. T. Fiadeiro, “Fusing iris and periocular information for cross-sensor recognition,” Pattern Recognition Letters, vol. 57, pp. 52–59, 2015.
F. Boutros, N. Damer, K. Raja, R. Ramachandra, F. Kirchbuchner, and A. Kuijper, “Iris and periocular biometrics for head mounted displays: Segmentation, recognition, and synthetic data generation,” Image and Vision Computing, vol. 104, p. 104007, 2020.
D. K. Jain, X. Lan, and R. Manikandan, “Fusion of iris and sclera using phase intensive rubbersheet mutual exclusion for periocular recognition,” Image and Vision Computing, vol. 103, p. 104024, 2020.
H. Proença and J. C. Neves, “Deep-prwis: Periocular recognition without the iris and sclera using deep learning frameworks,” IEEE Transactions on Information Forensics and Security, vol. 13, no. 4, pp. 888–896, 2017.
J. N. Kolf, F. Boutros, F. Kirchbuchner, and N. Damer, “Lightweight periocular recognition through low-bit quantization,” in 2022 IEEE International Joint Conference on Biometrics (IJCB), pp. 1–12, IEEE, 2022.
L. Nie, A. Kumar, and S. Zhan, “Periocular recognition using unsupervised convolutional rbm feature learning,” in 2014 22nd International Conference on Pattern Recognition, pp. 399–404, IEEE, 2014.
F. Boutros, N. Damer, K. Raja, F. Kirchbuchner, and A. Kuijper, “Template-driven knowledge distillation for compact and accurate periocular biometrics deep-learning models,” Sensors, vol. 22, no. 5, p. 1921, 2022.
S. S. Behera and N. B. Puhan, “High boost 3-d attention network for cross-spectral periocular recognition,” IEEE Sensors Letters, vol. 6, no. 9, pp. 1–4, 2022.
J. Brito and H. Proença, “A deep adversarial framework for visually explainable periocular recognition,” in Proceedings of the IEEE/CVF Conference on Computer Vision and Pattern Recognition, pp. 1453–1461, 2021.
K. Zhang, Z. Zhang, Z. Li, and Y. Qiao, “Joint face detection and alignment using multitask cascaded convolutional networks,” IEEE Signal Processing Letters, vol. 23, pp. 1499–1503, Oct 2016.
A. Dosovitskiy, L. Beyer, A. Kolesnikov, D. Weissenborn, X. Zhai, T. Unterthiner, M. Dehghani, M. Minderer, G. Heigold, S. Gelly, et al., “An image is worth 16x16 words: Transformers for image recognition at scale,” arXiv preprint arXiv:2010.11929, 2020.
A. Steiner, A. Kolesnikov, , X. Zhai, R. Wightman, J. Uszkoreit, and L. Beyer, “How to train your vit? data, augmentation, and regularization in vision transformers,” arXiv preprint arXiv:2106.10270, 2021.
A. Vaswani, N. Shazeer, N. Parmar, J. Uszkoreit, L. Jones, A. N. Gomez, L. Kaiser, and I. Polosukhin, “Attention is all you need,” in Proceedings of the 31st International Conference on Neural Information Processing Systems, NIPS’17, (Red Hook, NY, USA), p. 6000–6010, Curran Associates Inc., 2017.
T. Lin, Y. Wang, X. Liu, and X. Qiu, “A survey of transformers,” AI Open, vol. 3, pp. 111–132, 2022.
S. Khan, M. Naseer, M. Hayat, S. W. Zamir, F. S. Khan, and M. Shah, “Transformers in vision: A survey,” ACM computing surveys (CSUR), vol. 54, no. 10s, pp. 1–41, 2022.
J. Deng, J. Guo, N. Xue, and S. Zafeiriou, “Arcface: Additive angular margin loss for deep face recognition,” in Proceedings of the IEEE/CVF Conference on Computer Vision and Pattern Recognition, pp. 4690–4699, 2019.
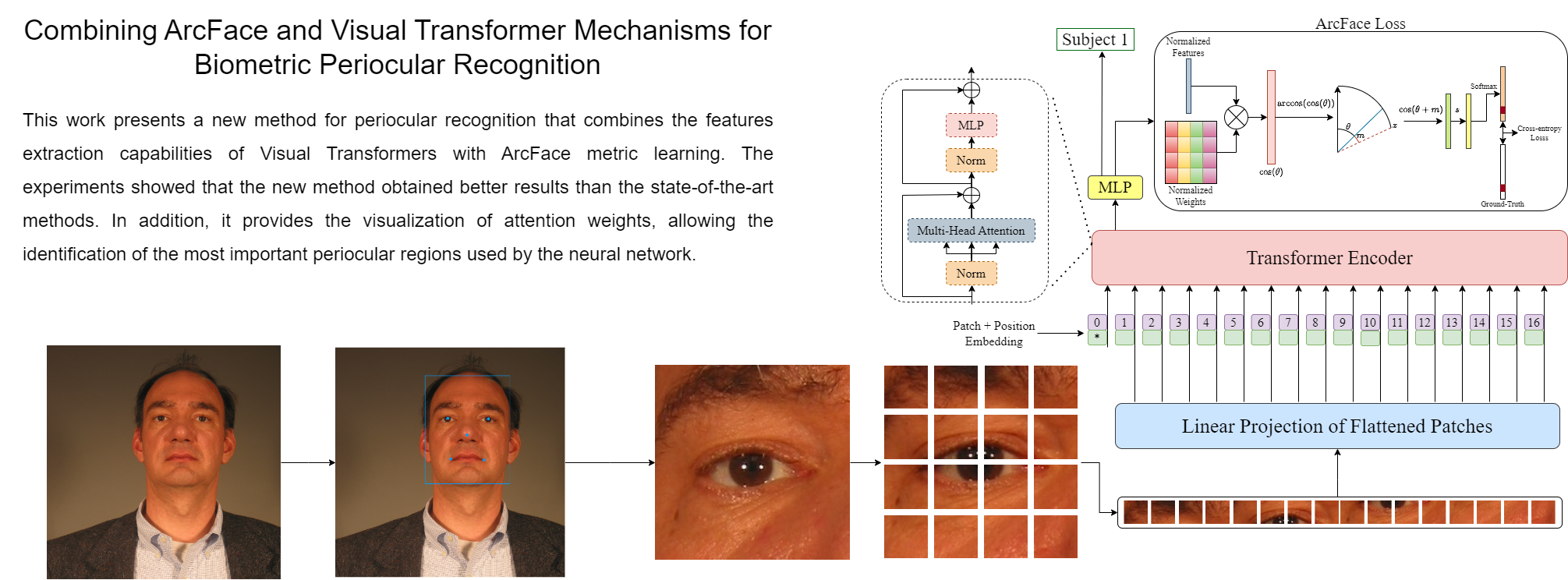