A graph-based superpixel segmentation method for measuring pressure ulcers
Keywords:
Graph-based superpixel segmentation, Pressure ulcers, Medical imagesAbstract
Monitoring wound healing is a necessary procedure to help health services control pressure ulcers. The correct diagnosis depends on clinical observations by doctors and nurses during patient visits. The evaluation of the wound area represents one of the most important data. Usually, health professionals assess ulcers through visual inspection, using rulers and decals. These ones, in direct contact with these lesions, may cause discomfort and inducing other infections, and consequently, worsen the patient’s clinical condition. Understanding and knowing these injuries allows for better preventive and therapeutic actions. In this paper, we aim to present an automatic and effective method for ulcer delineation according to the following pipeline: (i) graph-based superpixel segmentation; (ii) superpixel feature extraction; (iii) superpixel classification; (iv) ulcer segmentation; and (v) feature description. The main idea is to automatically compute pressure ulcer measurements for identifying the lesion area, allowing the follow-up of the scar evolution. Our graph-based superpixel segmentation method outperformed five other state-of-the-art approaches, as well as deep learning models, reaching 92.6% sensitivity, 98.6% specificity, 97.6% precision, 96.6% accuracy, and 90.4% intersection over the union.
Downloads
References
J. Tang, B. Li, J. Gong, W. Li, and J. Yang, “Challenges in the management of critical ill covid-19 patients with pressure ulcer,” International
Wound Journal, vol. 17, no. 5, pp. 1523–1524, 2020.
L. Peko, M. Barakat-Johnson, and A. Gefen, “Protecting prone positioned patients from facial pressure ulcers using prophylactic dressings: A timely biomechanical analysis in the context of the covid-19 pandemic,” International wound journal, vol. 17, no. 6, pp. 1595–1606, 2020.
S. Young, J. Narang, S. Kumar, E. Kwizera, P. Malik, S. D. Billings, J. S. Ko, and A. P. Fernandez, “Large sacral/buttocks ulcerations in the setting of coagulopathy: A case series establishing the skin as a target organ of significant damage and potential morbidity in patients with severe covid-19,” International wound journal, vol. 17, no. 6, pp. 2033–2037, 2020.
T. Wild, M. Prinz, N. Fortner, W. Krois, K. Sahora, S. Stremitzer, and T. Hölzenbein, “Digital measurement and analysis of wounds based on color segmentation,” European Surgery, vol. 40, pp. 5–10, 05 2014.
L. Wang, P. C. Pedersen, E. Agu, D. M. Strong, and B. Tulu, “Area determination of diabetic foot ulcer images using a cascaded two-stage
svm-based classification,” IEEE Transactions on Biomedical Engineering, vol. 64, no. 9, pp. 2098–2109, 2017.
R. H. L. e Silva and A. M. C. Machado, “A computational method for semi-automatic measurement of pressure ulcers,” Wound Repair Regen, vol. 26, no. 4, pp. 332–339, 2018.
R. H. L. e Silva and A. M. C. Machado, “Automatic measurement of pressure ulcers using support vector machines and grabcut,” Computer Methods and Programs in Biomedicine, vol. 200, p. 105867, 2021.
E. LE, B. JM, oldberg M, M. L, M. L, and S. M, “Revised national pressure ulcer advisory panel pressure injury staging system: Revised
pressure injury staging system.,” J Wound Ostomy Continence Nurs, vol. 43, pp. 585–597, Nov/Dec 2016.
R. Achanta et al., “Slic superpixels compared to state-of-the-art superpixel methods,” IEEE T. Pattern Anal., vol. 34, no. 11, pp. 2274–2282, 2012.
Z. Li and J. Chen, “Superpixel segmentation using linear spectral clustering,” in 2015 IEEE Conference on Computer Vision and Pattern
Recognition (CVPR), pp. 1356–1363, 2015.
M. V. den Bergh, X. Boix, G. Roig, and L. V. Gool, “Seeds: Superpixels extracted via energy-driven sampling,” 2013.
M.-Y. Liu, O. Tuzel, S. Ramalingam, and R. Chellappa, “Entropy rate superpixel segmentation,” in CVPR 2011, pp. 2097–2104, 2011.
R. Achanta and S. Susstrunk, “Superpixels and polygons using simple non-iterative clustering,” in IEEE Conference on Computer Vision and Pattern Recognition (CVPR), 2017.
F. J. Veredas, R. M. Luque-Baena, F. J. Martín-Santos, J. C. Morilla- Herrera, and L. Morente, “Wound image evaluation with machine
learning,” Neurocomput., vol. 164, p. 112–122, Sept. 2015.
L. Deng, H. Huang, J. Yuan, and X. Tang, “Superpixel based automatic segmentation of corneal ulcers from ocular staining images,” pp. 1–5, 2018.
H. Lu et al., “Wound intensity correction and segmentation with convolutional neural networks,” Concurr. Comp-Pract. E., vol. 29, no. 6,
pp. e3927–n/a, 2017.
X. Liu et al., “A framework of wound segmentation based on deep convolutional networks,” pp. 1–7, 2017.
S. Zahia et al., “Tissue classification and segmentation of pressure injuries using convolutional neural networks,” Comput. Meth. Prog. Bio., vol. 159, pp. 51–58, 2018.
F. Li et al., “A composite model of wound segmentation based on traditional methods and deep neural networks,” Comput. Intel. Neurosc., vol. 2018, 2018.
G. Blanco, A. J. Traina, C. Traina Jr., P. M. Azevedo-Marques, A. E. Jorge, D. de Oliveira, and M. V. Bedo, “A superpixel-driven deep
learning approach for the analysis of dermatological wounds,” Computer Methods and Programs in Biomedicine, vol. 183, p. 105079, Jan 2020.
M. Goyal, N. D. Reeves, S. Rajbhandari, N. Ahmad, C. Wang, and M. H. Yap, “Recognition of ischaemia and infection in diabetic foot
ulcers: Dataset and techniques,” Computers in Biology and Medicine, vol. 117, p. 103616, Feb 2020.
J. E. Vargas-Muñoz, A. S. Chowdhury, E. B. Alexandre, F. L. Galvão, P. A. Vechiatto Miranda, and A. X. Falcão, “An iterative spanning forest
framework for superpixel segmentation,” IEEE Transactions on Image Processing, vol. 28, no. 7, pp. 3477–3489, 2019.
F. C. Belem, S. J. F. Guimaraes, and A. X. Falcao, “Superpixel segmentation using dynamic and iterative spanning forest,” IEEE Signal
Processing Letters, vol. 27, p. 1440–1444, 2020.
MEDETEC, “Wound database: stock pictures of wounds,” 2007.
V. Kudva, K. Prasad, and S. Guruvare, “Detection of specular reflection and segmentation of cervix region in uterine cervix images for cervical cancer screening,” RBM - Revue Europeenne de Technologie Biomedicale, vol. 38, pp. 281–291, Oct. 2017.
A. Telea, “An image inpainting technique based on the fast marching method,” J. Graph. Tools, vol. 9, no. 1, pp. 23–34, 2004.
X. Ren and J. Malik, “Learning a classification model for segmentation,” in Proceedings of the Ninth IEEE International Conference on Computer Vision - Volume 2, ICCV ’03, (USA), p. 10, IEEE Computer Society, 2003.
R. Achanta, A. Shaji, K. Smith, A. Lucchi, P. Fua, and S. Süsstrunk, “Slic superpixels compared to state-of-the-art superpixel methods,” IEEE Transactions on Pattern Analysis and Machine Intelligence, vol. 34, no. 11, pp. 2274–2282, 2012.
V. Arvis, C. Debain, M. Berducat, and A. Benassi, “Generalization of the cooccurrence matrix for colour images: Application to colour texture classification,” Image Analysis & Stereology, vol. 23, pp. 63–72, 2011.
T. Ojala, M. Pietikainen, and T. Maenpaa, “Multiresolution gray-scale and rotation invariant texture classification with local binary patterns,” IEEE Transactions on Pattern Analysis and Machine Intelligence, vol. 24, no. 7, pp. 971–987, 2002.
I. Daubechies, “Orthonormal bases of compactly supported wavelets,” Communications on Pure and Applied Mathematics, vol. 41, no. 7, pp. 909–996, 1988.
S. Khalid, T. Khalil, and S. Nasreen, “A survey of feature selection and feature extraction techniques in machine learning,” 2014 Science and Information Conference, pp. 372–378, 2014.
J. Quinlan, “Decision trees and decision-making,” IEEE Transactions on Systems, Man, and Cybernetics, vol. 20, no. 2, pp. 339–346, 1990.
N. J. Nilsson, Artificial Intelligence: A New Synthesis. San Francisco, CA, USA: Morgan Kaufmann Publishers Inc., 1998.
R. Kohavi, “A study of cross-validation and bootstrap for accuracy estimation and model selection,” pp. 1137–1143, Morgan Kaufmann, 1995.
R. C. Gonzalez and R. E. Woods, Digital Image Processing (3rd Edition). USA: Prentice-Hall, Inc., 2006.
T. Y. Zhang and C. Y. Suen, “A fast parallel algorithm for thinning digital patterns,” Commun. ACM, vol. 27, p. 236–239, Mar. 1984.
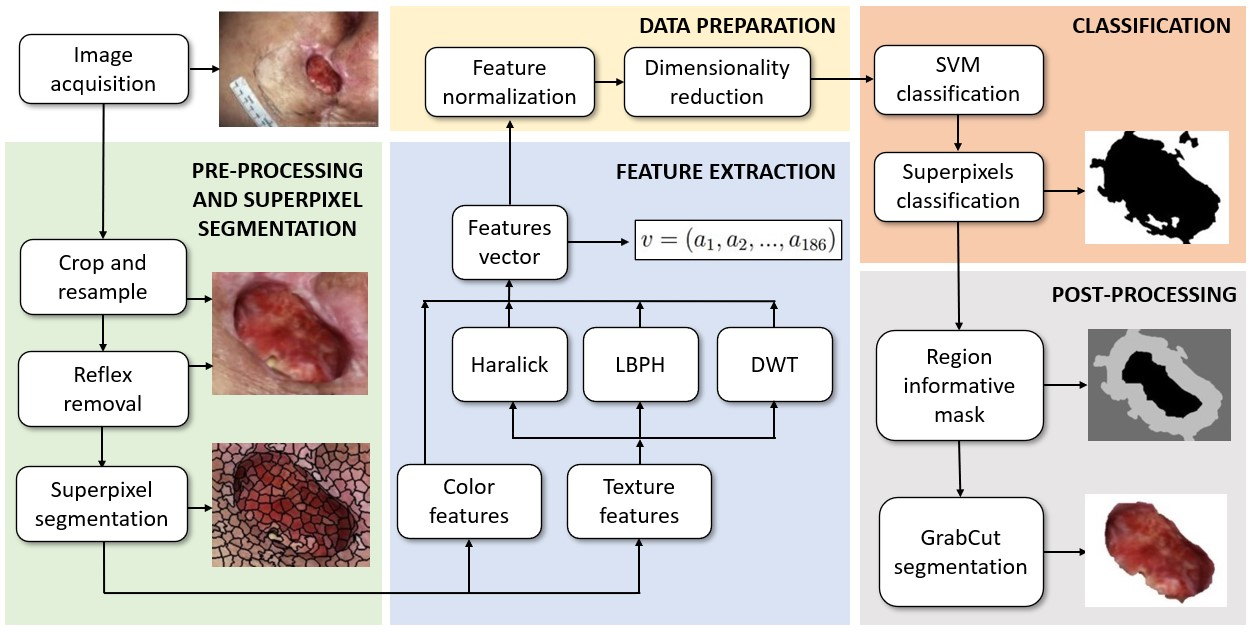