Using of Transformers Models for Text Classification to Mobile Educational Applications
Keywords:
Natural Language Processing, Multiclass Text Classification, Transformers, Bidirectional Encoder Representations from Transformers.Abstract
In Q2 2022, educational apps were the second most popular category on the Google Play Store, accounting for 10.47% of the apps available worldwide. This work explores the application of five BERT-based pre-trained models with the Transformers architecture to classify mobile educational applications. These five models are according to the knowledge field: bert-base-cased, bert-base-uncased, roberta-base, albert-base-v2 and distilbert-base-uncased. This study uses a dataset with educational apps of Google Play, this dataset was enriched with description and category because it lacked this information. In all models, a tokenizer and fine-tuning works were applied for training in the classification task. After training the data, the testing phase was performed in which the models had to go through four training epochs to obtain better results: roberta-base with 81% accuracy, bert-base-uncased with 79% accuracy, bert-base-cased obtained 80% accuracy, albert-base-v2 obtained 78% accuracy and distilbert-base-uncased obtained 76% accuracy.
Downloads
References
Natural Language Processing, Multiclass Text
Classification, Transformers, Bidirectional Encoder
Representations from Transformers.
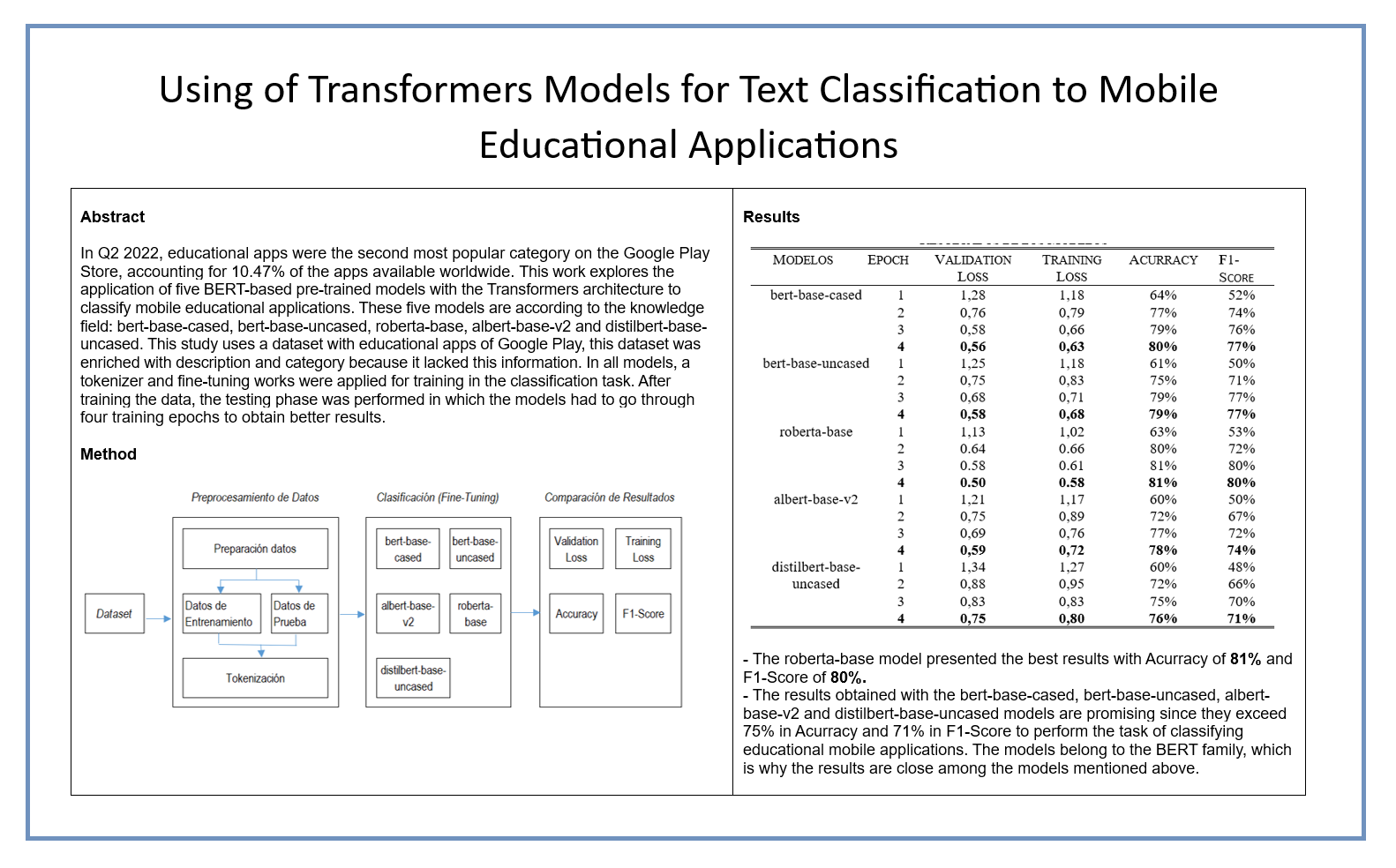