Dynamic Time Scan Forecasting: A Benchmark With M4 Competition Data
Keywords:
Univariate methods, M4 competition, benchmarking, dynamic time scan forecastingAbstract
Univariate forecasting methods are fundamental for many different application areas. M-competitions provide important benchmarks for scientists, researchers, statisticians, and engineers in the field, for evaluating and guiding the development of new forecasting techniques. In this paper, the Dynamic Time Scan Forecasting (DTSF), a new univariate forecasting method based on scan statistics, is presented. DTSF scans an entire time series, identifies past patterns whichare similar to the last available observations and forecasts based on the median of the subsequent observations of the most similar windows in past. In order to evaluate the performance of this method, a comparison with other statistical forecasting methods, applied in the M4 competition, is provided. In the hourly domain, an average sMAPE of 12.9% was achieved using hte method with the default parameters, while the baseline competition - the simple average of the forecasts of Holt, Damped and Theta methods - was 22.1%. The method proved to be competitive in longer time series, with high repeatability.
Downloads
References
T. Hill, L. Marquez, M. O’Connor, and W. Remus, “Artificial neural
network models for forecasting and decision making,” International
journal of forecasting, vol. 10, no. 1, pp. 5–15, 1994.
P.-F. Pai and C.-S. Lin, “A hybrid arima and support vector machines
model in stock price forecasting,” Omega, vol. 33, no. 6, pp. 497–505,
G. Dudek, “Pattern-based local linear regression models for short-term
load forecasting,” Electric Power Systems Research, vol. 130, pp. 139–
, 2016.
R. Shanmugam, “Book review,” Journal of Statistical Computation and
Simulation, vol. 76, no. 10, pp. 935–940, 2006.
T. T. Tchrakian, B. Basu, and M. O’Mahony, “Real-time traffic flow
forecasting using spectral analysis,” IEEE Transactions on Intelligent
Transportation Systems, vol. 13, no. 2, pp. 519–526, 2011.
C. Voyant, G. Notton, S. Kalogirou, M.-L. Nivet, C. Paoli, F. Motte, and
A. Fouilloy, “Machine learning methods for solar radiation forecasting:
A review,” Renewable Energy, vol. 105, pp. 569–582, 2017.
H. Hassani and E. S. Silva, “Forecasting uk consumer price inflation
using inflation forecasts,” Research in Economics, vol. 72, no. 3,
pp. 367–378, 2018.
W. Cai, J. Chen, J. Hong, and F. Jiang, “Forecasting chinese stock
market volatility with economic variables,” Emerging Markets Finance
and Trade, vol. 53, no. 3, pp. 521–533, 2017.
E. Bernardini and G. Cubadda, “Macroeconomic forecasting and structural
analysis through regularized reduced-rank regression,” International
Journal of Forecasting, vol. 31, no. 3, pp. 682–691, 2015.
Z. R. Khan Jaffur, N.-U.-H. Sookia, P. Nunkoo Gonpot, and B. Seetanah,
“Out-of-sample forecasting of the canadian unemployment rates
using univariate models,” Applied Economics Letters, vol. 24, no. 15,
pp. 1097–1101, 2017.
Y. Zhang, M. Zhong, N. Geng, and Y. Jiang, “Forecasting electric
vehicles sales with univariate and multivariate time series models: The
case of china,” PloS one, vol. 12, no. 5, p. e0176729, 2017.
G. A. Tularam and T. Saeed, “Oil-price forecasting based on various univariate
time-series models,” American Journal of Operations Research,
vol. 6, no. 03, p. 226, 2016.
G. Girish, A. K. Tiwari, et al., “A comparison of different univariate
forecasting models forspot electricity price in india,” Economics Bulletin,
vol. 36, no. 2, pp. 1039–1057, 2016.
M. Rana, I. Koprinska, and V. G. Agelidis, “Univariate and multivariate
methods for very short-term solar photovoltaic power forecasting,”
Energy Conversion and Management, vol. 121, pp. 380–390, 2016.
E. Raviv, K. E. Bouwman, and D. Van Dijk, “Forecasting day-ahead
electricity prices: Utilizing hourly prices,” Energy Economics, vol. 50,
pp. 227–239, 2015.
B. Billah, R. J. Hyndman, and A. B. Koehler, “Empirical information
criteria for time series forecasting model selection,” Journal of Statistical
Computation and Simulation, vol. 75, no. 10, pp. 831–840, 2005.
S. Makridakis, E. Spiliotis, and V. Assimakopoulos, “The m4 competition:
Results, findings, conclusion and way forward,” International
Journal of Forecasting, vol. 34, no. 4, pp. 802–808, 2018.
S. Makridakis and M. Hibon, “The m3-competition: results, conclusions
and implications,” International journal of forecasting, vol. 16, no. 4,
pp. 451–476, 2000.
S. Makridakis, C. Chatfield, M. Hibon, M. Lawrence, T. Mills, K. Ord,
and L. F. Simmons, “The m2-competition: A real-time judgmentally
based forecasting study,” International Journal of Forecasting, vol. 9,
no. 1, pp. 5–22, 1993.
S. Makridakis and M. Hibon, “Accuracy of forecasting: An empirical investigation,”
Journal of the Royal Statistical Society: Series A (General),
vol. 142, no. 2, pp. 97–125, 1979.
M. P. P. Flores, J. F. Solís, G. C. Valdez, J. J. G. Barbosa, J. P. Ortega, and
J. D. T. Villanueva, “Hurst exponent with arima and simple exponential
smoothing for measuring persistency of m3-competition series,” IEEE
Latin America Transactions, vol. 17, no. 05, pp. 815–822, 2019.
S. Makridakis, E. Spiliotis, and V. Assimakopoulos, “The m5 competition:
Background, organization, and implementation,” International
Journal of Forecasting, 2021.
“The m6 financial forecasting competition.”
D. Yang and S. Alessandrini, “An ultra-fast way of searching weather
analogs for renewable energy forecasting,” Solar Energy, vol. 185,
pp. 255–261, 2019.
L. E. B. Hoeltgebaum, N. L. Dias, and M. A. Costa, “An analog period
method for gap-filling of latent heat flux measurements,” Hydrological
Processes, vol. 35, no. 4, p. e14105, 2021.
M. A. Costa, R. Ruiz-Cárdenas, L. B. Mineti, and M. O. Prates,
“Dynamic time scan forecasting for multi-step wind speed prediction,”
Renewable Energy, vol. 177, pp. 584–595, 2021.
T. S. Gontijo, M. A. Costa, and R. B. de Santis, “Similarity search in
electricity prices: An ultra-fast method for finding analogs,” Journal of
Renewable and Sustainable Energy, vol. 12, no. 5, p. 056103, 2020.
T. S. Gontijo, M. A. Costa, and R. B. de Santis, “Electricity price
forecasting on electricity spot market: a case study based on the brazilian
difference settlement price,” in E3S Web of Conferences, vol. 239, EDP
Sciences, 2021.
G. Bontempi, “Comments on m4 competition,” International Journal of
Forecasting, vol. 36, no. 1, pp. 201–202, 2020.
R. Fildes and S. Makridakis, “The impact of empirical accuracy studies
on time series analysis and forecasting,” International Statistical Review/
Revue Internationale de Statistique, pp. 289–308, 1995.
J. Glaz and N. Balakrishnan, Scan statistics and applications. Springer
Science & Business Media, 2012.
D. C. Montgomery, E. A. Peck, and G. G. Vining, Introduction to linear
regression analysis. John Wiley & Sons, 2021.
C. Chatfield, Time-series forecasting. Chapman and Hall/CRC, 2000.
R. Hyndman, A. B. Koehler, J. K. Ord, and R. D. Snyder, Forecasting
with exponential smoothing: the state space approach. Springer Science
& Business Media, 2008.
E. S. Gardner and E. McKenzie, “Why the damped trend works,” Journal
of the Operational Research Society, vol. 62, no. 6, pp. 1177–1180,
V. Assimakopoulos and K. Nikolopoulos, “The theta model: a decomposition
approach to forecasting,” International journal of forecasting,
vol. 16, no. 4, pp. 521–530, 2000.
G. E. Box and D. A. Pierce, “Distribution of residual autocorrelations in
autoregressive-integrated moving average time series models,” Journal
of the American statistical Association, vol. 65, no. 332, pp. 1509–1526,
R. J. Hyndman, A. B. Koehler, R. D. Snyder, and S. Grose, “A state
space framework for automatic forecasting using exponential smoothing
methods,” International Journal of forecasting, vol. 18, no. 3, pp. 439–
, 2002.
S. M. Al-Alawi and S. M. Islam, “Principles of electricity demand
forecasting. i. methodologies,” Power Engineering Journal, vol. 10,
no. 3, pp. 139–143, 1996.
A. Azadeh, S. Ghaderi, and S. Sohrabkhani, “Annual electricity consumption
forecasting by neural network in high energy consuming
industrial sectors,” Energy Conversion and management, vol. 49, no. 8,
pp. 2272–2278, 2008.
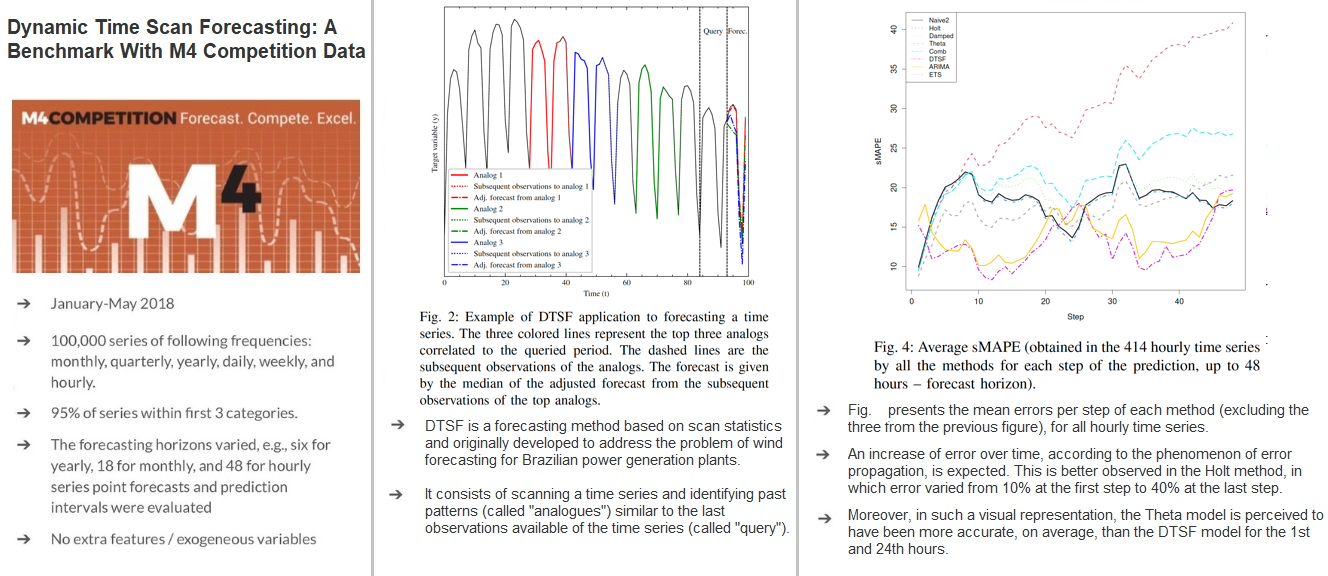