The Role of Artificial Intelligence in Latin America’s Energy Transition
Keywords:
Artificial intelligence, maturity model, energy transition, power systems, smart grid, machine learning, Latin AmericaAbstract
Latin America’s energy transition involves the massive integration of sustainable energy, different than hydro, at large and small scale, consumer empowerment, and the adoption of emerging information and communication technologies (ICT) in the entire electricity sector. These factors boost the usage of Artificial Intelligence (AI) to transform the traditional energy industry into a more complex cyber-physical ecosystem. But unlocking the full AI potential requires understanding working principles, current existing applications, and its comprehensive impact on the energy value chain. This paper discusses the role of AI in this context, emphasizing the key factors for successful implementation in the region and proposing an AI maturity model for the energy transition that allows determining the status and gaps for AI adoption.
Downloads
References
REFERENCES
B. F. Wollenberg and T. Sakaguchi, “Artificial intelligence in power system operations,” Proc. IEEE, vol. 75, no. 12, pp. 1678–1685, Dec. 1987, doi: 10.1109/PROC.1987.13935.
IRENA (2019), “Innovation landscape brief: Artificial intelligence and big data,” International Renewable Energy Agency, Abu Dhabi.
World Economic Forum, “Harnessing Artificial Intelligence to Accelerate the Energy Transition.” Sep. 01, 2021. [Online]. Available: https://www3.weforum.org/docs/WEF_Harnessing_AI_to_accelerate_the_Energy_Transition_2021.pdf
“The global AI agenda: Latin America | MIT Technology Review.” https://www.technologyreview.com/2020/06/08/1002864/the-global-ai-agenda-latin-america/ (accessed Mar. 12, 2021).
“2021 Latin American and the Caribbean Energy Outlook,” OLADE, Dec. 16, 2021.
IRENA (2016), “Renewable Energy Market Analysis: Latin America.” IRENA.
“DER and EVs Benefit with Renewables Expected to Grow 276% in Latin America.” https://guidehouseinsights.com/news-and-views/der-and-evs-benefit-with-renewables-expected-to-grow-276-in-latin-america (accessed Sep. 06, 2020).
OECD (2019), “Shaping the Digital Transformation in Latin America: Strengthening Productivity, Improving Lives.” OECD Publishing. [Online]. Available: https://doi.org/10.1787/8bb3c9f1-en
“Calidad del aire - OPS/OMS | Organización Panamericana de la Salud.”
C. Viviescas et al., “Contribution of Variable Renewable Energy to increase energy security in Latin America: Complementarity and climate change impacts on wind and solar resources,” Renew. Sustain. Energy Rev., vol. 113, p. 109232, Oct. 2019, doi: 10.1016/j.rser.2019.06.039.
P. L. Donti and J. Z. Kolter, “Machine Learning for Sustainable Energy Systems,” Annu. Rev. Environ. Resour., vol. 46, no. 1, pp. 719–747, 2021, doi: 10.1146/annurev-environ-020220-061831.
A. Mosavi, M. Salimi, S. Faizollahzadeh Ardabili, T. Rabczuk, S. Shamshirband, and A. R. Varkonyi-Koczy, “State of the Art of Machine Learning Models in Energy Systems, a Systematic Review,” Energies, vol. 12, no. 7, 2019, doi: 10.3390/en12071301.
EPRI, “An Introduction to AI, its Use Cases, and Requirements for the Electric Power Industry.” Electric Power Research Institute (EPRI), Aug. 2019.
C. Kuster, Y. Rezgui, and M. Mourshed, “Electrical load forecasting models: A critical systematic review,” Sustain. Cities Soc., vol. 35, pp. 257–270, Nov. 2017, doi: 10.1016/j.scs.2017.08.009.
S. Wu et al., “Survey on Prediction Algorithms in Smart Homes,” IEEE Internet Things J., vol. 4, no. 3, pp. 636–644, Jun. 2017.
B. Najafi, S. Moaveninejad, and F. Rinaldi, “Chapter 17 - Data Analytics for Energy Disaggregation: Methods and Applications,” in Big Data Application in Power Systems, R. Arghandeh and Y. Zhou, Eds. Elsevier, 2018, pp. 377–408. doi: 10.1016/B978-0-12-811968-6.00017-6.
Q. Wang, C. B. Martinez-Anido, H. Wu, A. R. Florita, and B. Hodge, “Quantifying the Economic and Grid Reliability Impacts of Improved Wind Power Forecasting,” IEEE Trans. Sustain. Energy, vol. 7, no. 4, pp. 1525–1537, Oct. 2016.
Y. Ren, P. N. Suganthan, and N. Srikanth, “Ensemble methods for wind and solar power forecasting—A state-of-the-art review,” Renew. Sustain. Energy Rev., vol. 50, pp. 82–91, Oct. 2015, doi: 10.1016/j.rser.2015.04.081.
C. F. M. Coimbra, J. Kleissl, and R. Marquez, “Chapter 8 - Overview of Solar-Forecasting Methods and a Metric for Accuracy Evaluation,” in Solar Energy Forecasting and Resource Assessment, J. Kleissl, Ed. Boston: Academic Press, 2013, pp. 171–194. doi: 10.1016/B978-0-12-397177-7.00008-5.
V. Bali, A. Kumar, and S. Gangwar, “Deep Learning based Wind Speed Forecasting-A Review,” in 2019 9th International Conference on Cloud Computing, Data Science & Engineering (Confluence), Jan. 2019, pp. 426–431. doi: 10.1109/CONFLUENCE.2019.8776923.
C. Kasburg and S. Frizzo Stefenon, “Deep Learning for Photovoltaic Generation Forecast in Active Solar Trackers,” IEEE Lat. Am. Trans., vol. 17, no. 12, pp. 2013–2019, Dec. 2019.
Organización Latinoamericana de Energía (OLADE), Ed., ENERLAC. Revista de energía de Latinoamérica y el Caribe. Año 2018. No.1. Quito: OLADE, 2018.
C. Suncast, “Suncast consolida servicio predictivo con más de 1.000 MW en Chile,” Mi Sitio, Apr. 22, 2022. https://www.suncast.cl/post/suncast-consolida-servicio-predictivo-con-más-de-1-000-mw-en-chile (accessed Jun. 24, 2022).
“Analysis of the forecasting system in the Dominican Republic.” https://www.energymeteo.com/customers/customer_projects/power-forecasts_dom-rep.php (accessed Jun. 24, 2022).
“UL Selected by National Energy Operator in Mexico to Provide Wind, Solar Forecasts | UL.” https://www.ul.com/news/ul-selected-national-energy-operator-mexico-provide-wind-solar-forecasts (accessed Aug. 21, 2020).
Operador Nacional do Sistema Elétrico (ONS), “Submódulo 18.2. Relação dos sistemas e modelos computacionais.” ONS, Jan. 2020. [Online]. Available: http://www.ons.org.br/%2FProcedimentosDeRede%2FM%C3%B3dulo%2018%2FSubm%C3%B3dulo%2018.2%2FSubm%C3%B3dulo%2018.2%202020.01.pdf
Elia Grid International, “Support for the short term improvement of the current renewable energy forecasting in Chile.” Deutsche Gesellschaft für Internationale Zusammenarbeit (GIZ) GmbH, Jan. 14, 2016.
Universidad del CEMA, “Integración de Renovables a la Operación y el Despacho.” [Online]. Available: https://ucema.edu.ar/conferencias/download/2018/09.05ER.pdf
“Brazil Wind Energy: Notable Output but Inaccurate Forecasts.” https://www.fitchratings.com/research/infrastructure-project-finance/brazil-wind-energy-notable-output-inaccurate-forecasts-09-08-2019 (accessed Aug. 08, 2020).
IRENA (2018), “Evaluación del Estado de Preparación de las Energías Renovables Panamá.” Agencia Internacional de Energías Renovables, Abu Dhabi.
J. Haas et al., “Sunset or sunrise? Understanding the barriers and options for the massive deployment of solar technologies in Chile,” Energy Policy, vol. 112, pp. 399–414, Jan. 2018, doi: 10.1016/j.enpol.2017.10.001.
T. A. Siddiqui, S. Bharadwaj, and S. Kalyanaraman, “A Deep Learning Approach to Solar-Irradiance Forecasting in Sky-Videos,” 2019 IEEE Winter Conf. Appl. Comput. Vis. WACV, pp. 2166–2174, 2019.
Northeast Group, LLC, “South America Smart Grid: Market Forecast (2020 – 2029).” Volume V, May 2020.
S. Ramchurn, P. Vytelingum, A. Rogers, and N. Jennings, “Putting the ‘Smarts’ into the Smart Grid: A Grand Challenge for Artificial Intelligence,” Commun. ACM - CACM, vol. 55, pp. 86–97, Apr. 2012, doi: 10.1145/2133806.2133825.
B. K. Bose, “Artificial Intelligence Techniques in Smart Grid and Renewable Energy Systems—Some Example Applications,” Proc. IEEE, vol. 105, no. 11, pp. 2262–2273, Nov. 2017, doi: 10.1109/JPROC.2017.2756596.
D. Zhang, X. Han, and C. Deng, “Review on the research and practice of deep learning and reinforcement learning in smart grids,” CSEE J. Power Energy Syst., vol. 4, no. 3, pp. 362–370, Sep. 2018, doi: 10.17775/CSEEJPES.2018.00520.
S. S. Ali and B. J. Choi, “State-of-the-Art Artificial Intelligence Techniques for Distributed Smart Grids: A Review.,” Electronics, vol. 9, no. 6, p. 1030, Jun. 22, 2020.
E. S. Rigas, S. D. Ramchurn, and N. Bassiliades, “Managing Electric Vehicles in the Smart Grid Using Artificial Intelligence: A Survey,” IEEE Trans. Intell. Transp. Syst., vol. 16, no. 4, pp. 1619–1635, Aug. 2015, doi: 10.1109/TITS.2014.2376873.
S. M. Zahraee, M. Khalaji Assadi, and R. Saidur, “Application of Artificial Intelligence Methods for Hybrid Energy System Optimization,” Renew. Sustain. Energy Rev., vol. 66, pp. 617–630, Dec. 2016, doi: 10.1016/j.rser.2016.08.028.
E. Mocanu et al., “On-Line Building Energy Optimization Using Deep Reinforcement Learning,” IEEE Trans. Smart Grid, vol. 10, no. 4, pp. 3698–3708, Jul. 2019, doi: 10.1109/TSG.2018.2834219.
I. Antonopoulos et al., “Artificial intelligence and machine learning approaches to energy demand-side response: A systematic review,” Renew. Sustain. Energy Rev., vol. 130, p. 109899, Sep. 2020, doi: 10.1016/j.rser.2020.109899.
X. Xu, Y. Jia, Y. Xu, Z. Xu, S. Chai, and C. S. Lai, “A Multi-Agent Reinforcement Learning-Based Data-Driven Method for Home Energy Management,” IEEE Trans. Smart Grid, vol. 11, no. 4, pp. 3201–3211, Jul. 2020.
L. Yu et al., “Deep Reinforcement Learning for Smart Home Energy Management,” IEEE Internet Things J., vol. 7, no. 4, pp. 2751–2762, Apr. 2020.
S. Lin, F. Li, E. Tian, Y. Fu, and D. Li, “Clustering Load Profiles for Demand Response Applications,” IEEE Trans. Smart Grid, vol. 10, no. 2, pp. 1599–1607, Mar. 2019, doi: 10.1109/TSG.2017.2773573.
Y. Chen and J. M. Chang, “EMaaS: Cloud-Based Energy Management Service for Distributed Renewable Energy Integration,” IEEE Trans. Smart Grid, vol. 6, no. 6, pp. 2816–2824, Nov. 2015, doi: 10.1109/TSG.2015.2446980.
S. Bera, S. Misra, and J. J. P. C. Rodrigues, “Cloud Computing Applications for Smart Grid: A Survey,” IEEE Trans. Parallel Distrib. Syst., vol. 26, no. 5, pp. 1477–1494, May 2015.
Y. Liu, C. Yang, L. Jiang, S. Xie, and Y. Zhang, “Intelligent Edge Computing for IoT-Based Energy Management in Smart Cities,” IEEE Netw., vol. 33, no. 2, pp. 111–117, Apr. 2019.
S. Khan, D. Paul, P. Momtahan, and M. Aloqaily, “Artificial intelligence framework for smart city microgrids: State of the art, challenges, and opportunities,” in 2018 Third International Conference on Fog and Mobile Edge Computing (FMEC), Apr. 2018, pp. 283–288.
H. Holttinen et al., “Methodologies to Determine Operating Reserves Due to Increased Wind Power,” IEEE Trans. Sustain. Energy, vol. 3, no. 4, pp. 713–723, Oct. 2012, doi: 10.1109/TSTE.2012.2208207.
H. Yuan et al., “Machine Learning-Based PV Reserve Determination Strategy for Frequency Control on the WECC System,” in 2020 IEEE Power & Energy Society Innovative Smart Grid Technologies Conference (ISGT), Feb. 2020, pp. 1–5. doi: 10.1109/ISGT45199.2020.9087744.
L. Liu and Z. Hu, “Data-Driven Regulation Reserve Capacity Determination Based on Bayes Theorem,” IEEE Trans. Power Syst., vol. 35, no. 2, pp. 1646–1649, Mar. 2020.
V. M. Meza Jiménez and J. D. Durán Hernandez, “A Data-driven Tool for Primary Frequency Regulation Evaluation,” presented at the Cigré Session 2018, Paris, France, May 2018.
V. M. Meza Jiménez and B. C. Pérez Romero, “A Machine Learning based Tool for AGC Performance Evaluation,” in 2019 FISE-IEEE/CIGRE Conference - Living the energy Transition (FISE/CIGRE), Dec. 2019, pp. 1–5.
D. Cao, W. Hu, J. Zhao, Q. Huang, Z. Chen, and F. Blaabjerg, “A Multi-agent Deep Reinforcement Learning Based Voltage Regulation using Coordinated PV Inverters,” IEEE Trans. Power Syst., pp. 1–1, 2020.
Z. Yan and Y. Xu, “Data-Driven Load Frequency Control for Stochastic Power Systems: A Deep Reinforcement Learning Method With Continuous Action Search,” IEEE Trans. Power Syst., vol. 34, no. 2, pp. 1653–1656, Mar. 2019.
S. S. Halilčevic, C. Moraga, and B. Imamović, “Neural-network-based equipment for the power system frequency control,” in Mediterranean Conference on Power Generation, Transmission, Distribution and Energy Conversion (MedPower 2016), Nov. 2016, pp. 1–8. doi: 10.1049/cp.2016.1084.
D. Xu, J. Liu, X. Yan, and W. Yan, “A Novel Adaptive Neural Network Constrained Control for a Multi-Area Interconnected Power System With Hybrid Energy Storage,” IEEE Trans. Ind. Electron., vol. 65, no. 8, pp. 6625–6634, Aug. 2018, doi: 10.1109/TIE.2017.2767544.
O. Stanojev, O. Kundavcina, U. Markovic, E. Vrettos, P. Aristidou, and G. Hug, “A Reinforcement Learning Approach for Fast Frequency Control in Low-Inertia Power Systems,” ArXiv, vol. abs/2007.05474, 2020.
J. Duan et al., “Deep-Reinforcement-Learning-Based Autonomous Voltage Control for Power Grid Operations,” IEEE Trans. Power Syst., vol. 35, no. 1, pp. 814–817, Jan. 2020.
Q. Yang, G. Wang, A. Sadeghi, G. B. Giannakis, and J. Sun, “Two-Timescale Voltage Control in Distribution Grids Using Deep Reinforcement Learning,” IEEE Trans. Smart Grid, vol. 11, no. 3, pp. 2313–2323, May 2020.
M. Glavic, “(Deep) Reinforcement learning for electric power system control and related problems: A short review and perspectives,” Annu. Rev. Control, vol. 48, pp. 22–35, Jan. 2019, doi: 10.1016/j.arcontrol.2019.09.008.
J. Zhang, Z. Chen, C. He, Z. Jiang, and L. Guan, “Data-Driven-Based Optimization for Power System Var-Voltage Sequential Control,” IEEE Trans. Ind. Inform., vol. 15, no. 4, pp. 2136–2145, Apr. 2019, doi: 10.1109/TII.2018.2856826.
X. Meng, G. Sun, J. Li, and H. Liu, “Automatic reactive power and voltage control for regional power grid based on SVM,” in 2013 IEEE International Conference of IEEE Region 10 (TENCON 2013), Oct. 2013, pp. 1–4.
R. Diao, Z. Wang, D. Shi, Q. Chang, J. Duan, and X. Zhang, “Autonomous Voltage Control for Grid Operation Using Deep Reinforcement Learning,” 2019 IEEE Power Energy Soc. Gen. Meet. PESGM, pp. 1–5, 2019.
J. V. Espinoza, A. Guzmán, F. Calero, M. V. Mynam, E. Palma, and Z. Korkmaz, “Wide-area synchrophasors protect and control Central America’s power system stability,” in 2014 Saudi Arabia Smart Grid Conference (SASG), Dec. 2014, pp. 1–9. doi: 10.1109/SASG.2014.7274294.
A. M. Khalil and R. Iravani, “Power System Coherency Identification Under High Depth of Penetration of Wind Power,” IEEE Trans. Power Syst., vol. 33, no. 5, pp. 5401–5409, Sep. 2018.
X. Liu, X. Zeng, L. Yao, G. I. Rashed, and C. Deng, “Power System State Estimation Based on Fusion of WAMS/SCADA Measurements: A Survey,” in 2018 2nd IEEE Conference on Energy Internet and Energy System Integration (EI2), Oct. 2018, pp. 1–6.
D. Kim, A. White, and Y. Shin, “PMU-Based Event Localization Technique for Wide-Area Power System,” IEEE Trans. Power Syst., vol. 33, no. 6, pp. 5875–5883, Nov. 2018.
L. Cai, N. F. Thornhill, S. Kuenzel, and B. C. Pal, “Wide-Area Monitoring of Power Systems Using Principal Component Analysis and $k$-Nearest Neighbor Analysis,” IEEE Trans. Power Syst., vol. 33, no. 5, pp. 4913–4923, Sep. 2018.
S. Liu et al., “Data-Driven Event Detection of Power Systems Based on Unequal-Interval Reduction of PMU Data and Local Outlier Factor,” IEEE Trans. Smart Grid, vol. 11, no. 2, pp. 1630–1643, Mar. 2020.
S. Bhamidipati, K. J. Kim, H. Sun, and P. V. Orlik, “Artificial-Intelligence-Based Distributed Belief Propagation and Recurrent Neural Network Algorithm for Wide-Area Monitoring Systems,” IEEE Netw., vol. 34, no. 3, pp. 64–72, Jun. 2020, doi: 10.1109/MNET.011.1900322.
W. Wang et al., “Frequency Disturbance Event Detection Based on Synchrophasors and Deep Learning,” IEEE Trans. Smart Grid, vol. 11, no. 4, pp. 3593–3605, Jul. 2020.
Y. Hashmy, Z. Yu, D. Shi, and Y. Weng, “Wide-area Measurement System-based Low Frequency Oscillation Damping Control through Reinforcement Learning,” IEEE Trans. Smart Grid, pp. 1–1, 2020.
NASPI Engineering Analysis Task Team (EATT), “Data Mining Techniques and Tools for Synchrophasor Data.” North American SynchroPhasor Initiative (NASPI), Jan. 2019. [Online]. Available: https://www.naspi.org/sites/default/files/reference_documents/naspi_data_mining_tech_pnnl_28218_final.pdf
R. Yousefian, A. Sahami, and S. Kamalasadan, “Hybrid Transient Energy Function-Based Real-Time Optimal Wide-Area Damping Controller,” IEEE Trans. Ind. Appl., vol. 53, no. 2, pp. 1506–1516, Apr. 2017, doi: 10.1109/TIA.2016.2624264.
J. Mattioli, P. Perico, and P. Robic, “Artificial Intelligence based Asset Management,” in 2020 IEEE 15th International Conference of System of Systems Engineering (SoSE), Jun. 2020, pp. 151–156. doi: 10.1109/SoSE50414.2020.9130505.
A. Stetco et al., “Machine learning methods for wind turbine condition monitoring: A review,” Renew. Energy, vol. 133, pp. 620–635, Apr. 2019, doi: 10.1016/j.renene.2018.10.047.
EPRI, “Five Artificial Intelligence Grand Challenges for the Electric Power Industry.” Electric Power Research Institute (EPRI), Sep. 29, 2021. [Online]. Available: https://www.epri.com/research/products/000000003002022804
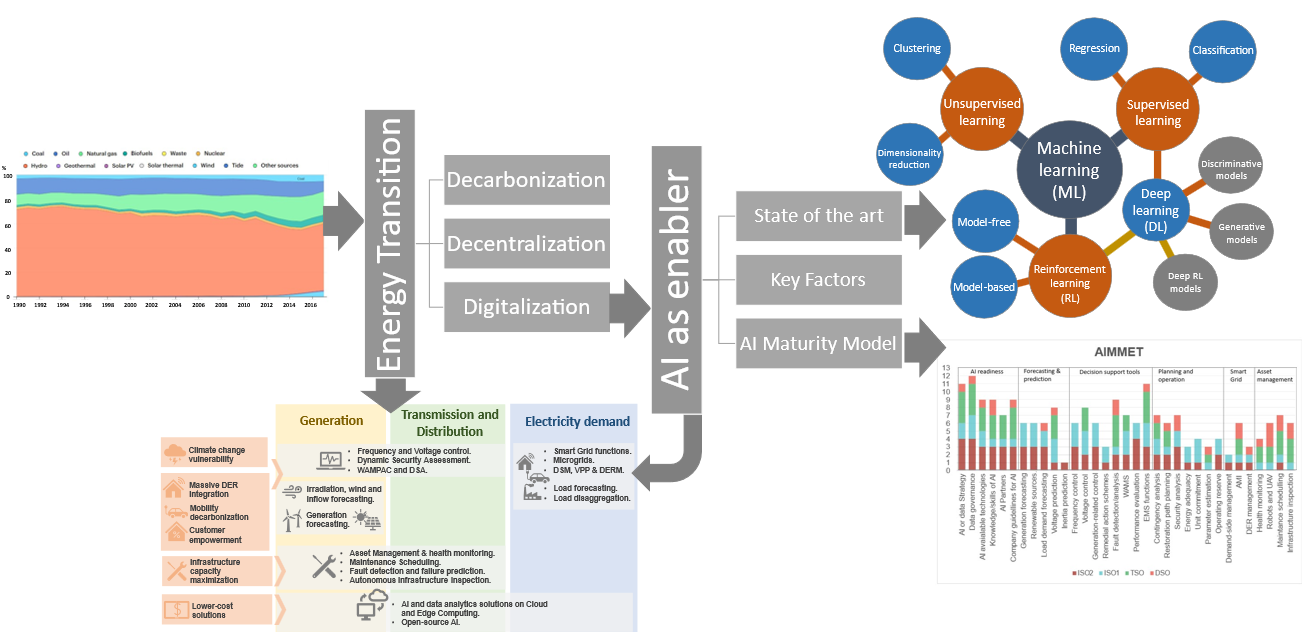