Subspace Predictive Control Tuning with Multiobjetive Optimization
Keywords:
Subspace Predictive Control, Control Tuning, Multiobjective OptimizationAbstract
In this paper, a tuning method for data-driven, subspace predictive controllers is proposed. The tuning approach is based on the solution of a multiobjective optimization, in which the optimization problem is defined as the minimization of the quadratic error between closed-loop response and some desired reference trajectories. In its turn, these trajectories are described in function of user-defined time-domain objectives. The tuning parameters are obtained as the compromise solutions of the multiobjective optimization problem. Design choices and performance are discussed and the method is validated in computational simulations of some common types of process. Additionally, the tuning methodology is implemented in a multivariable pilot-scale thermoelectrical plant.
Downloads
References
E. F. Camacho and C. B. Alba, Model predictive control. Springer, 2013.
L. Wang, Model predictive control system design and implementation using MATLAB®. Springer, 2009.
J. L. Garriga and M. Soroush, “Model predictive control tuning methods: A review,” Industrial & Engineering Chemistry Research, vol. 49, no. 8, pp. 3505–3515, 2010.
M. Alhajeri and M. Soroush, “Tuning guidelines for model-predictive control,” Industrial & Engineering Chemistry Research, vol. 59, no. 10, pp. 4177–4191, 2020.
B. Huang and R. Kadali, Dynamic modeling, predictive control and performance monitoring: a data-driven subspace approach. Springer, 2008.
K. J. Keesman, System identification: an introduction. Springer, 2011.
R. Isermann and M. Münchhof, Identification of dynamic systems: an introduction with applications. Springer, 2010.
W. Favoreel, B. De Moor, and M. Gevers, “Spc: Subspace predictive control,” IFAC Proceedings Volumes, vol. 32, no. 2, pp. 4004–4009, 1999.
V. Vajpayee, S. Mukhopadhyay, and A. P. Tiwari, “Data-driven subspace predictive control of a nuclear reactor,” IEEE Transactions on Nuclear Science, vol. 65, no. 2, pp. 666–679, 2017.
S. Sedghizadeh and S. Beheshti, “Data-driven subspace predictive control: Stability and horizon tuning,” Journal of the Franklin Institute, vol. 355, no. 15, pp. 7509–7547, 2018.
P. Van Overschee and B. De Moor, Subspace identification for linear systems: Theory, Implementation, Applications. Springer, 1996.
R. T. Marler and J. S. Arora, “Survey of multi-objective optimization methods for engineering,” Structural and multidisciplinary optimization, vol. 26, no. 6, pp. 369–395, 2004.
M. Vallerio, J. Van Impe, and F. Logist, “Tuning of nmpc controllers via multi-objective optimisation,” Computers & Chemical Engineering, vol. 61, pp. 38–50, 2014.
R. Toro, C. Ocampo-Martínez, F. Logist, J. Van Impe, and V. Puig, “Tuning of predictive controllers for drinking water networked systems,” IFAC Proceedings Volumes, vol. 44, no. 1, pp. 14507–14512, 2011.
A. Yamashita, A. Zanin, and D. Odloak, “Tuning of model predictive control with multi-objective optimization,” Brazilian Journal of Chemical Engineering, vol. 33, no. 2, pp. 333–346, 2016.
The MathWorks Inc., “Simulink - simulation and model-based design.” https://www.mathworks.com/products/simulink.html, 2021.
R. Lima, C. C. Dias, and P. R. Barros, “Desenvolvimento de uma planta térmica multivariável baseada em módulos peltier,” in XLII Congresso Brasileiro de Educaçao em Engenharia (COBENGE), 2014.
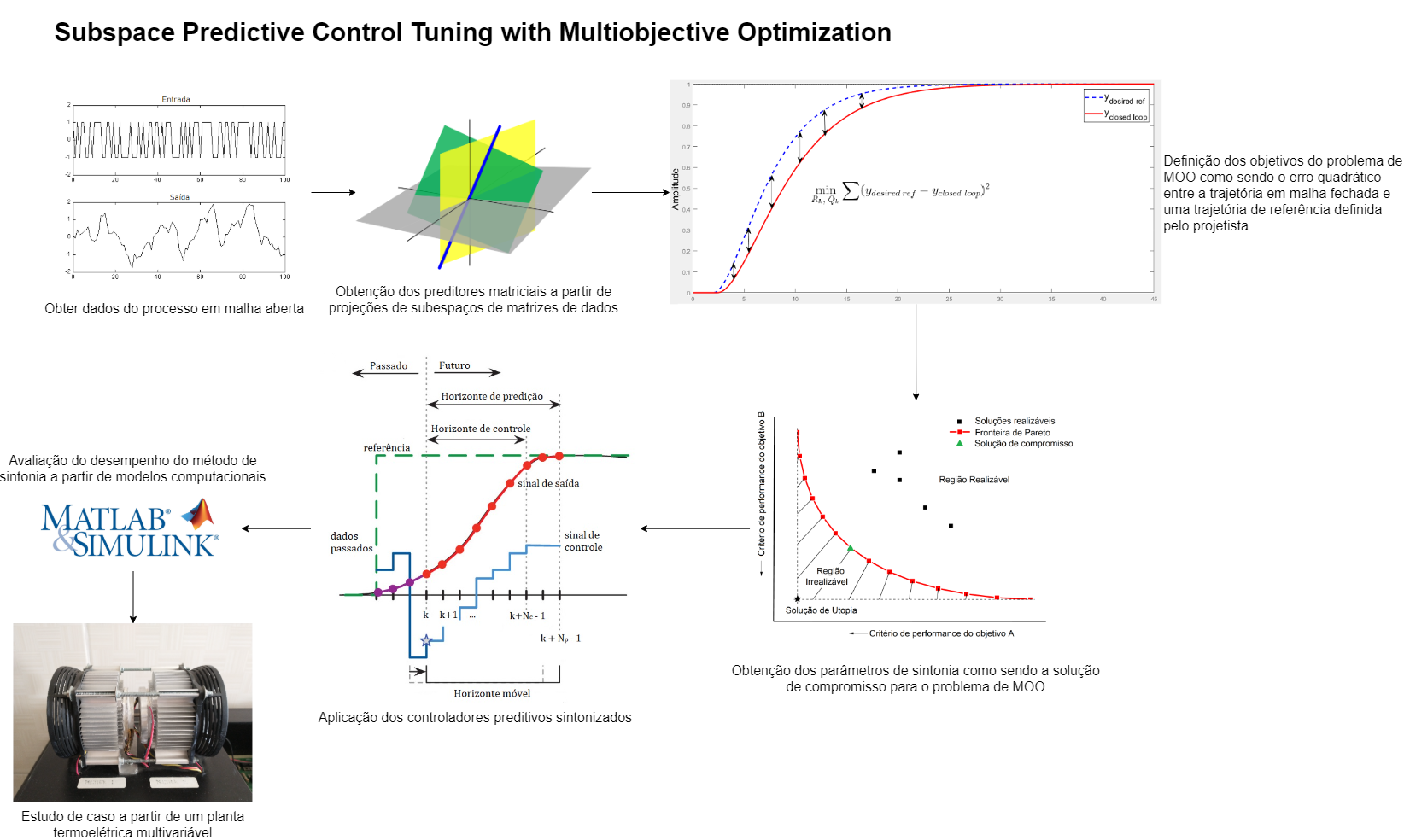