Less acoustic features means more statistical relevance: Disclosing the clustering behavior in music stimuli
Keywords:
music, audio analysis, cluster analysisAbstract
Identification of appropriate content-based features for the description of audio signals can provide a better repre-
sentation of naturalistic music stimuli which, in recent years, have been used to understand how the human brain processes such information. In this work, an extensive clustering analysis has been carried out on a large and benchmark audio dataset to assess whether features commonly extracted in the literature are in fact statistically relevant. Our results show that not all of these well-known acoustic features might be statistically necessary. We also demonstrate quantitatively that, regardless of the musical genre, the same acoustic feature is selected to represent each cluster. This finding discloses that there is a general redundancy among the set of audio descriptors used, that does not depend on a particular music track or genre, allowing an expressive reduction of the number of features necessary to identify apropriate time instants on the audio for further brain signal processing of music stimuli.
Downloads
References
D. Mitrovic, M. Zeppelzauer, and C. Breiteneder, “Features for content based audio retrieval,” in Advances in Computers Volume 78 Improving the Web, pp. 71–150, Elsevier, 2010.
V. Alluri, P. Toiviainen, I. P. Jaaskelainen, E. Glerean, M. Sams, and E. Brattico, “Large-scale brain networks emerge from dynamics
processing of musical timbre, key and rhythm,” NeuroImage, vol. 59,no. 4, pp. 3677–3689, 2012.
V. Alluri, P. Toiviainen, T. E. Lund, M. Wallentin, P. Vuust, K. Nandi, Asoke, T. Ristaniemi, and E. Brattico, “From vivaldi to beatles and back:Predicting lateralized brain responses to music,” NeuroImage, vol. 83, pp. 627–636, Dec. 2013.
H. Poikonen, V. Alluri, E. Brattico, O. Lartillot, M. Tervaniemi, and M. Huotilainen, “Event-related brain responses while listening to entire pieces of music,” Neuroscience, vol. 312, pp. 58–73, Jan. 2016.
P. Saari, I. Burunat, E. Brattico, and P. Toiviainen, “Decoding musical training from dynamic processing of musical features in the brain,”Scientific Report, vol. 708, no. 8, pp. 1–12, 2018.
E. Ribeiro and C. E. Thomaz, “A whole brain eeg analysis of musicianship,” Music Perception: An Interdisciplinary Journal, vol. 37, no. 1,
pp. 42–56, 2019.
N. T. Haumann, M. Lumaca, M. Kliuchko, J. L. Santacruz, P. Vuust, and E. Brattico, “Extracting human cortical responses to sound onsets and acoustic feature changes in real music, and their relation to event rate,” Brain Research, p. 147248, 2021.
L. A. Ferreira, E. Ribeiro, and C. E. Thomaz, “A multivariate statistical analysis of eeg signals for differentiation of musicians and nonmusicians,”in 17th Brazilian Symposium on Computer Music (SBCM) (J. T. Araújo and F. L. Schiavoni, eds.), (Sao Paulo, Brazil), pp. 80–85,Brazilian Computer Society (SBC), 10 2019.
G. Tzanetakis and P. Cook, “Musical genre classification of audio signals,” IEEE Transactions on Speech and Audio Processing, vol. 10,
pp. 293–302, 7 2002.
B. L. Sturm, “The state of the art ten years after a state of the art: Future research in music information retrieval,” Journal of New Music Research, vol. 43, p. 147–172, 4 2014.
O. Lartillot, MIRtoolbox 1.6.1 Users Manual, 2014.
A. Lerch, An Introduction to audio content analysis. Applications in signal processing and music informatics. IEEE Press, 1st ed., 2012.
P. Kness and M. Schedl, Music Similarity and Retrieval: An introduction to audio and web based strategies. Springer, 1st ed., 2016.
H. Poikonen, P. Toiviainen, and M. Tervaniemi, “Early auditory processing in musicians and dancers during a contemporary dance piece,” Scientific Reports, vol. 6, Sept. 2016.
R. A. Johnson and D. W. Wichern, Applied Multivariate Statistical Analysis. Pearson, 6th ed., 2007.
H. F. Kaiser, “The varimax criterion for analytic rotation in factor analysis,” Psychometrika, vol. 23, pp. 187–200, 1958.
P. J. Rousseeuw, “Silhouettes: A graphical aid to the interpretation and validation of cluster analysis,” Journal of Computational and Applied Mathematics, vol. 20, pp. 53 – 65, 1987.
D. L. Davies and D. W. Bouldin, “A cluster separation measure,” IEEE Transactions on Pattern Analysis and Machine Intelligence, vol. PAMI-1, no. 2, pp. 224–227, 1979.
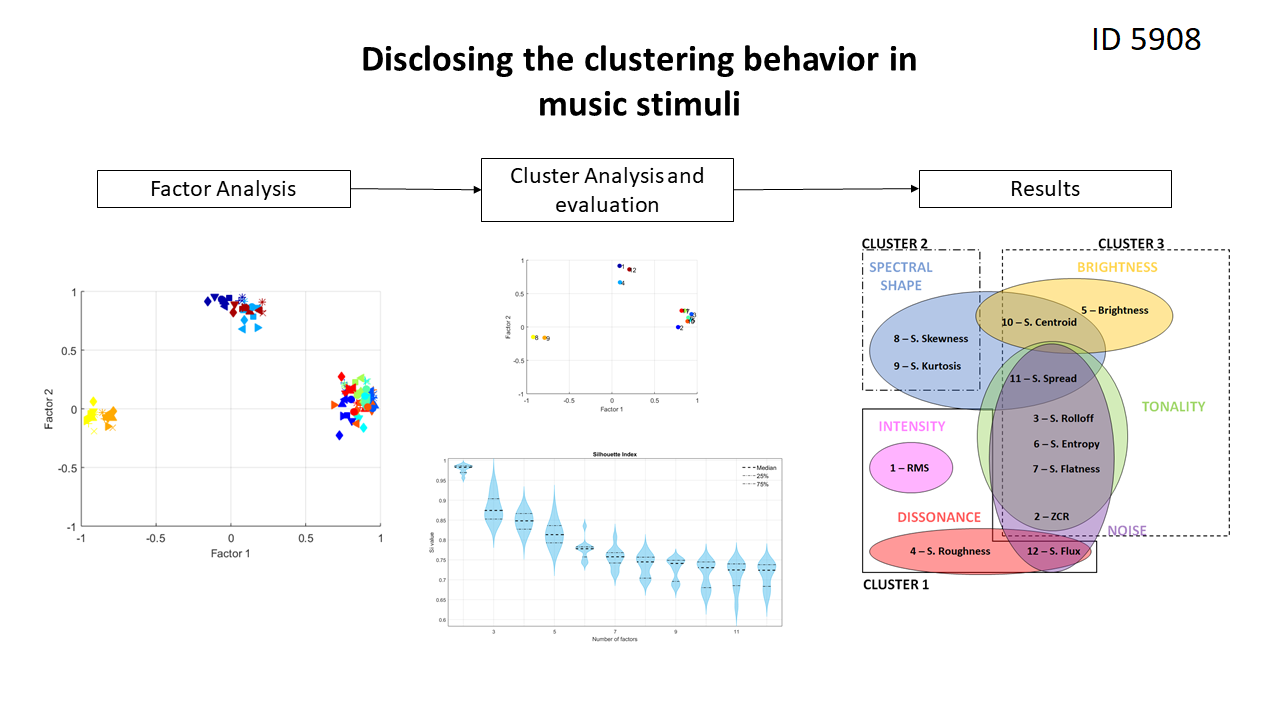