Estimation of the parameters of the mathematical model of an equivalent diode of a photovoltaic panel using a continuous genetic algorithm
Keywords:
Renewable energy, Solar, photovoltaic, parameters identification, modeling,, Photovoltaic systems, Optimization methods, Mathematical model, Genetic algorithmsAbstract
This document presents the implementation of a con- tinuous population genetic optimization algorithm (CGA) as a solution method to the parameter estimation problem of a diode model (SDM) of a photovoltaic panel (PV) from experimental data of voltage versus current (V-I). The parameters to be estimated by means of the CGA are: the photoinduced current, the diode saturation current, the ideality factor, the series resistance and the parallel resistance. The estimation of the SDM parameters is carried out in order to obtain the real values that represent the power profile of the panel and thus carry out an analysis of its physical state. For which, the mean square error of the PV current estimated by the solution method from the selected parameters is used as the objective function, with the real curve of the PV panel used as the test scenario. All of the above subject to the set of restrictions that limits the problem under analysis. To validate the effectiveness and robustness of the proposed method, in this document two comparison methods have been used: the particle swarm optimization method (PSO) and a traditional genetic algorithm (GAT). In addition, four different panel types were used to generate the test scenarios: the MSX60, the SOLAR SJ65, the KYOCERA KC200GT, and the STP245S. All simulations were obtained using MATLAB 2019b. The results obtained in this document show that the proposed method presents the best relationship between the estimation of parameters and the computation time required to solve the SDM problem.
Downloads
References
M. Azab, F. Salem, and M. Mosaad, “Pv parameters estimation using dif- ferent evolutionary algorithms,” Journal of Electrical Engineering, vol. 13, no. 4, 2013.
F. Bonanno, G. Capizzi, G. Graditi, C. Napoli, and G. M. Tina, “A radial ba- sis function neural network based approach for the electrical characteristics estimation of a photovoltaic module,” Applied Energy, vol. 97, pp. 956–961, 2012.
H. Wang, Y. Liu, M. Li, H. Huang, H. Xu, R. Hong, and H. Shen, “Multifunctional tio2 nanowires-modified nanoparticles bilayer film for 3d dye-sensitized solar cells,” Optoelectron. Adv. Mater. Rapid Commun, vol. 4, no. 8, pp. 1166–1169, 2010.
M. A. Green, E. D. Dunlop, D. H. Levi, J. Hohl-Ebinger, M. Yoshita, and A. W. Ho-Baillie, “Solar cell efficiency tables (version 54),” Progress in Photovoltaics: Research and Applications, vol. 27, no. 7, pp. 565–575, 2019.
M. Q. Taha, Q. H. Eesse, and S. M. Salih, “Mathematical modeling of different photovoltaic modules,” Journal of telecommunications, vol. 11, no. 2, pp. 59–64, 2011.
B. C. Babu and S. Gurjar, “A novel simplified two-diode model of photo- voltaic (pv) module,” IEEE journal of photovoltaics, vol. 4, no. 4, pp. 1156– 1161, 2014.
V. Tamrakar, S. Gupta, and Y. Sawle, “Single-diode and two-diode pv cell modeling using matlab for studying characteristics of solar cell under varying conditions,” Electrical & Computer Engineering: An International Journal (ECIJ), vol. 4, no. 2, pp. 67–77, 2015.
K. Ishaque, Z. Salam, et al., “A comprehensive matlab simulink pv system simulator with partial shading capability based on two-diode model,” Solar energy, vol. 85, no. 9, pp. 2217–2227, 2011.
J. S. Barroso, J. Correia, N. Barth, S. Ahzi, and M. Khaleel, “A pso algorithm for the calculation of the series and shunt resistances of the pv panel one- diode model,” in 2014 International Renewable and Sustainable Energy Conference (IRSEC), pp. 1–6, IEEE, 2014.
A. Tobón, J. Peláez-Restrepo, J. P. Villegas-Ceballos, S. I. Serna-Garcés, J. Herrera, and A. Ibeas, “Maximum power point tracking of photovoltaic panels by using improved pattern search methods,” Energies, vol. 10, no. 9, p. 1316, 2017.
J. Ma, T. Ting, K. L. Man, N. Zhang, S.-U. Guan, and P. W. Wong, “Parameter estimation of photovoltaic models via cuckoo search,” Journal of applied mathematics, vol. 2013, 2013.
N. Maouhoub, “Photovoltaic module parameter estimation using an an- alytical approach and least squares method,” Journal of Computational Electronics, vol. 17, no. 2, pp. 784–790, 2018.
G. Ciulla, V. L. Brano, V. Di Dio, and G. Cipriani, “A comparison of different one-diode models for the representation of i–v characteristic of a pv cell,” Renewable and Sustainable Energy Reviews, vol. 32, pp. 684– 696, 2014.
H. Nunes, J. Pombo, J. Fermeiro, S. Mariano, and M. do Rosário Calado, “Particle swarm optimization for photovoltaic model identification,” in 2017 International Young Engineers Forum (YEF-ECE), pp. 53–58, IEEE, 2017.
R. Benkercha, S. Moulahoum, I. Colak, and B. Taghezouit, “Pv module parameters extraction with maximum power point estimation based on flower pollination algorithm,” in 2016 IEEE International Power Electronics and Motion Control Conference (PEMC), pp. 442–449, IEEE, 2016.
G. Petrone, C. A. Ramos-Paja, and G. Spagnuolo, Photovoltaic sources modeling. John Wiley & Sons, 2017.
A. A. El Tayyan, “An approach to extract the parameters of solar cells from their illuminated iv curves using the lambert w function,” Turkish Journal of Physics, vol. 39, no. 1, pp. 1–15, 2015.
V.J.Chin,Z.Salam,andK.Ishaque,“Cellmodellingandmodelparameters estimation techniques for photovoltaic simulator application: A review,” Applied Energy, vol. 154, pp. 500–519, 2015.
M. AlRashidi, M. AlHajri, K. El-Naggar, and A. Al-Othman, “A new estimation approach for determining the i–v characteristics of solar cells,” Solar Energy, vol. 85, no. 7, pp. 1543–1550, 2011.
N. Rajasekar, N. K. Kumar, and R. Venugopalan, “Bacterial foraging algorithm based solar pv parameter estimation,” Solar Energy, vol. 97, pp. 255–265, 2013.
A. Askarzadeh and A. Rezazadeh, “Artificial bee swarm optimization algorithm for parameters identification of solar cell models,” Applied Energy, vol. 102, pp. 943–949, 2013.
N. Gokmen, E. Karatepe, B. Celik, and S. Silvestre, “Simple diagnostic approach for determining of faulted pv modules in string based pv arrays,” Solar Energy, vol. 86, no. 11, pp. 3364–3377, 2012.
S. A. Rahman, R. K. Varma, and T. Vanderheide, “Generalised model of a photovoltaic panel,” IET Renewable Power Generation, vol. 8, no. 3, pp. 217–229, 2014.
S. Lineykin, M. Averbukh, and A. Kuperman, “Five-parameter model of photovoltaic cell based on stc data and dimensionless,” in 2012 IEEE 27th Convention of Electrical and Electronics Engineers in Israel, pp. 1–5, IEEE, 2012.
J. P. Ram, T. S. Babu, and N. Rajasekar, “A comprehensive review on solar
pv maximum power point tracking techniques,” Renewable and Sustainable
Energy Reviews, vol. 67, pp. 826–847, 2017.
S. Messalti, A. Harrag, and A. Loukriz, “A new variable step size neural net-
works mppt controller: Review, simulation and hardware implementation,”
Renewable and Sustainable Energy Reviews, vol. 68, pp. 221–233, 2017. [27] M. G. Villalva, J. R. Gazoli, and E. Ruppert Filho, “Comprehensive approach to modeling and simulation of photovoltaic arrays,” IEEE Transactions on
power electronics, vol. 24, no. 5, pp. 1198–1208, 2009.
D. Bonkoungou, Z. Koalaga, and D. Njomo, “Modelling and simulation of photovoltaic module considering single-diode equivalent circuit model in matlab,” International Journal of Emerging Technology and Advanced
Engineering, vol. 3, no. 3, pp. 493–502, 2013.
O. Hachana, K. Hemsas, G. Tina, and C. Ventura, “Comparison of dif-
ferent metaheuristic algorithms for parameter identification of photovoltaic cell/module,” Journal of renewable and sustainable energy, vol. 5, no. 5, p. 053122, 2013.
Y. Yoon and Z. W. Geem, “Parameter optimization of single-diode model of photovoltaic cell using memetic algorithm,” International Journal of Photoenergy, vol. 2015, 2015.
Y. Zhang, P. Lin, Z. Chen, and S. Cheng, “A population classification evolution algorithm for the parameter extraction of solar cell models,” International Journal of Photoenergy, vol. 2016, 2016.
A. Askarzadeh and A. Rezazadeh, “Parameter identification for solar cell models using harmony search-based algorithms,” Solar Energy, vol. 86, no. 11, pp. 3241–3249, 2012.
Z. Chen, L. Wu, P. Lin, Y. Wu, and S. Cheng, “Parameters identification of photovoltaic models using hybrid adaptive nelder-mead simplex algorithm based on eagle strategy,” Applied Energy, vol. 182, pp. 47–57, 2016.
M. Galante, “Un algoritmo genético simple para la optimización de estruc- turas planas articuladas,” 1993.
P.ChuandJ.Beasley,“Ageneticalgorithmforthesetpartitioningproblem,” Imperial College, London, 1995.
O. Montoya, W. Gil-González, and L. Grisales-Noreña, “Optimal power dispatch of dgs in dc power grids: a hybrid gauss-seidel genetic-algorithm methodology for solving the opf problem,” WSEAS Transactions on Power Systems, vol. 13, no. 33, pp. 335–346, 2018.
S. S. Souza, R. Romero, J. Pereira, and J. T. Saraiva, “Specialized genetic algorithm of chu-beasley applied to the distribution system reconfiguration problem considering several demand scenarios,” in 2015 IEEE Eindhoven PowerTech, pp. 1–5, Ieee, 2015.
V. Khanna, B. Das, D. Bisht, P. Singh, et al., “Estimation of photovoltaic cells model parameters using particle swarm optimization,” in Physics of Semiconductor Devices, pp. 391–394, Springer, 2014.
E. Koroishi, F. Lara-Molina, A. Borges, and V. Steffen Jr, “Robust control in rotating machinery using linear matrix inequalities,” Journal of Vibration and Control, vol. 22, no. 17, pp. 3767–3778, 2016.
A.AhlawatandA.Ramaswamy,“Multiobjectiveoptimalstructuralvibration control using fuzzy logic control system,” Journal of Structural Engineering, vol. 127, no. 11, pp. 1330–1337, 2001.
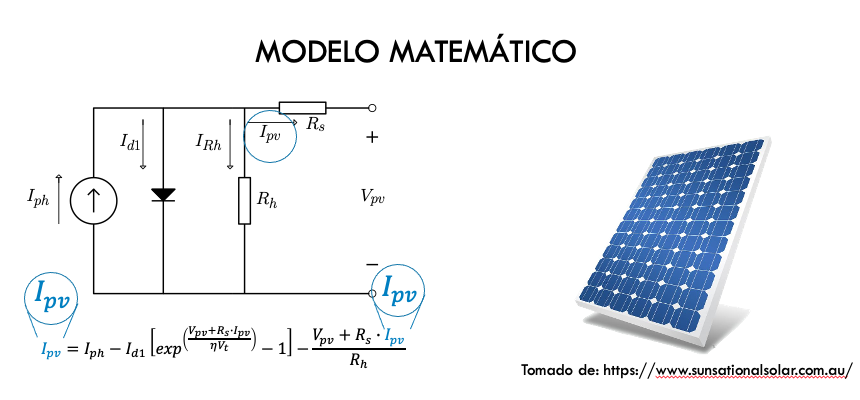