A Data Governance Framework for Industry 4.0
Keywords:
Data governance, Data-Centric architecture, Industry 4.0, Big Data, Real TimeAbstract
The fourth industrial revolution, or Industry 4.0, represents a new stage of evolution in the organization, management and control of the value chain throughout the product or service life cycle. This digitization of the industrial environment is characterized by the connection of Information Technologies (IT) and Operations Technologies (OT) through cyber-physical systems and the Industrial IoT (IIoT). One of the main consequences of this integration is the increasing amount and variety of data generated in real time from different sources. In this environment of intensive generation of actionable information, data becomes a critical asset for Industry 4.0, at all stages of the value chain. However, in order to data become a competitive advantage for the company, it must be managed and governed like any other strategic asset, and therefore it is necessary to rely on a Data Governance system. Industry 4.0 requires a reformulation of governance since the data is a key element and the backbone of the processes of the organization. This paper proposes a Reference Framework for the implementation of Data Governance Systems for Industry 4.0. Previously, it contextualizes data governance for Industry 4.0 environments and identifies the requirements that this framework must address, which are conditioned by the specific features of Industry 4.0, among others, the intensive use of big data, cloud and edge computing, artificial intelligence and current regulations.
Downloads
References
Deloitte Consulting LLP. Industry 4.0. Challenges and Solutions for the Digital Transformation and Use of Exponential Technologies, 1-32, (2015).
Velásquez et al. Cloud Computing, Big Data and the Industry 4.0 Reference Architectures. Journal of Computer Science and Technology, 18.03 (2018), 258-266. <https://doi.org/10.24215/16666038.18.e29>.
Kevin Kwang. “Third Platform” Shift Triggers Enterprise Software Evolution. ZDNet, 1–4 <https://www.zdnet.com/article/third-platform-shift-triggers-enterprise-software-evolution/> [accessed 26 October 2018].
Stratio. Data-Centric Architecture A Model for Embracing the Machine Age, 1-7, (2018).
DAMA International. DAMA-DMBOK2: Data Management Body of Knowledge, ed. by Deborah Henderson and Susan Earley, 2nd edn, (2017)
Alhassan et al. Data Governance Activities: An Analysis of the Literature. Journal of Decision Systems,64–75, (2016) <https://doi.org/10.1080/12460125.2016.1187397>.
Mabkhot et al. Requirements of the Smart Factory System: A Survey and Perspective. Machines, 6.2 (2018), 1-22. <https://doi.org/10.3390/MACHINES6020023>.
Yebenes & Zorrilla. Towards a Data Governance Framework for Third Generation Platforms. Procedia Computer Science, 151 (2019), 614–21 <https://doi.org/10.1016/j.procs.2019.04.082>.
López et al. RAI4 Deployment Tool and Metamodel. <https://github.com/istr-uc/RAI4DeploymentTool> [accessed 28 September 2020].
Weber et al. One Size Does Not Fit All - A Contingency Approach to Data Governance. Journal of Data and Information Quality, 1.1 (2009), 4:1--4:27 <https://doi.org/10.1145/1515693.1515696>.
MDM Institute. Data Governance Definition. <http://www.tcdii.com/whatIsDataGovernance.html>. [accessed 23 October 2018].
Gray & IBM Corporation. An Overview of Data Governance Elevator Pitch (Final), 1-4, (2011).
Otto, 2011. Data Governance. Business and Information Systems Engineering, 3.4 (2011), 241–44 <https://doi.org/10.1007/s12599-011-0162-8>.
ISACA, 2018. COBIT 2019 Framework. Introduction and Methodology. 1–64 <https://doi.org/10.1057/9780230299283_1>.
The Open Group, 2018. TOGAF® Standard Version 9.2. 197.
MIT Technology Review Insights, 2020. Data on Demand: Dynamic Architecture for a High-Speed Age, 4.
Felici et al., 2013. Accountability for Data Governance in Cloud Ecosystems., Proceedings of the 2013 IEEE International Conference on Cloud Computing Technology and Science-Volume 2, 2013, 327–332. <https://doi.org/10.1109/CloudCom.2013.157>.
Deloitte et al., 2018. Data Governance for Next-Generation Platforms, 1-11.
IIC. 2018. Industrial Internet Consortium. The Industrial Internet of Things Volume G2: Key System Concerns, 2018, pp. 1–43.
Sohail et al., 2018. 4 Pillars to Guide Data Governance for New Platforms. <https://deloitte.wsj.com/cio/2018/10/10/4-data-governance-pillars-for-modern-data-platforms/>, [accessed 26 July 2019].
Wallis et al., 2020, Agreements Between Enterprises Digitized by Smart Contracts in the Domain of Industry 4.0. Computer Science & Information Technology (CS & IT), 23–32 <https://doi.org/10.5121/csit.2020.101003>.
ISO, 2017b. International Standard Organization - ISO/IEC 38505-1. Application of ISO/IEC 38500 to the Governance of Data, pp. 1–28.
ISO, 2018. International Standard Organization - ISO/IEC TR 38505-2. Implications of ISO/IEC 38505-1 for Data Management.
Strod, 2020. Continuous Governance with DataGovOps. Datakitchen Blog, 2020. <https://blog.datakitchen.io/blog/continuous-governance-with-datagovops> [accessed 15 September 2020]
Ballard et al., 2014. IBM Information Governance Solutions, ed. by IBM Corporation, 1-264.
Madsen & Bergh, 2020. Redefining Data Governance with DataGovOps (Webinar) <https://info.datakitchen.io/watch-on-demand-webinar-redefining-data-governance-with-datagovops>.
Williams & Tang, 2020. Data Quality Management for Industry 4.0: A Survey. Data Quality, 22.2 (2020), 26–35.
Collibra, 2018. The Need for Big Data Governance, 1-8.
IIC. 2016. Industrial Internet Consortium. The Industrial Internet of Things Volume G4: Security Framework, Industrial Internet Consortium, 2016, pp. 1–173.
IIC. 2109. Industrial Internet Consortium. Data Protection Best Practices, 1-38.
DataKitchen, 2020. What Is-Dataops. Ten Most Common Questions. Blog of DataKitchen <https://blog.datakitchen.io/blog/what-is-dataops-ten-most-common-questions> [accessed 2 March 2020].
ISO, 2017a. International Standard Organization - ISO/IEC/IEEE 24765 Systems and Software Engineering - Vocabulary, 2017, pp. 1–530.
ISO, 2008. International Standard Organization - ISO/IEC 38500:2008(E) Corporate Governance of Information Technology).
Brous et al., 2016. Coordinating Decision-Making in Data Management Activities: A Systematic Review of Data Governance Principles. Lecture Notes in Computer Science, 9820 LNCS, 115–125. <https://doi.org/10.1007/978-3-319-44421-5_9>.
ISO, 2011. International Standard Organization - ISO/IEC/IEEE 42010:2011 Systems and Software Engineering — Architecture Description, 2011, pp. 1–46 <https://doi.org/10.1080/21670811.2017.1279978>.
Carretero et al., 2017. MAMD 2.0: Environment for Data Quality Processes Implantation Based on ISO 8000-6X and ISO/IEC 33000. Computer Standards and Interfaces, 54. September 2016 (2017), 139–51 <https://doi.org/10.1016/j.csi.2016.11.008>.
Object Management Group, 2005. Reusable Asset Specification, Version 2.2, pp. 1–121.
The Open Group, 2014. TOGAF® 9.1 Translation Glossary: English – Castilian Spanish, 1-45.
C González García, ER Núñez-Valdez, V García-Díaz, Juan Manuel Cueva Lovelle A Review of Artificial Intelligence in the Internet of Things, International Journal of Interactive Multimedia and Artificial Intelligence, 5(4), pp. 9-20, 2019.
Avison, D.; F. Myers L.M.; Nielsen A. Action Research. (1999). Communications of the ACM, 42(1): 94-97.
Katherine Downing, AHIMA Information Governance & The Information Governance Adoption Model (IGAMTM), 2017< http://www.himconnect.ca/Documents/The Information Governance Adoption - AHIMA.pdf>.
Gwen Thomas, ‘The DGI Data Governance Framework’, The Data Governance Institute, Orlando, FL (USA), 2006, 20.
Majid Al-Ruithe, ‘Development and Evaluation of a Holistic Framework and Maturity Assessment Tools for Data Governance in Cloud Computing Environments’ (Staffordshire University, 2018).
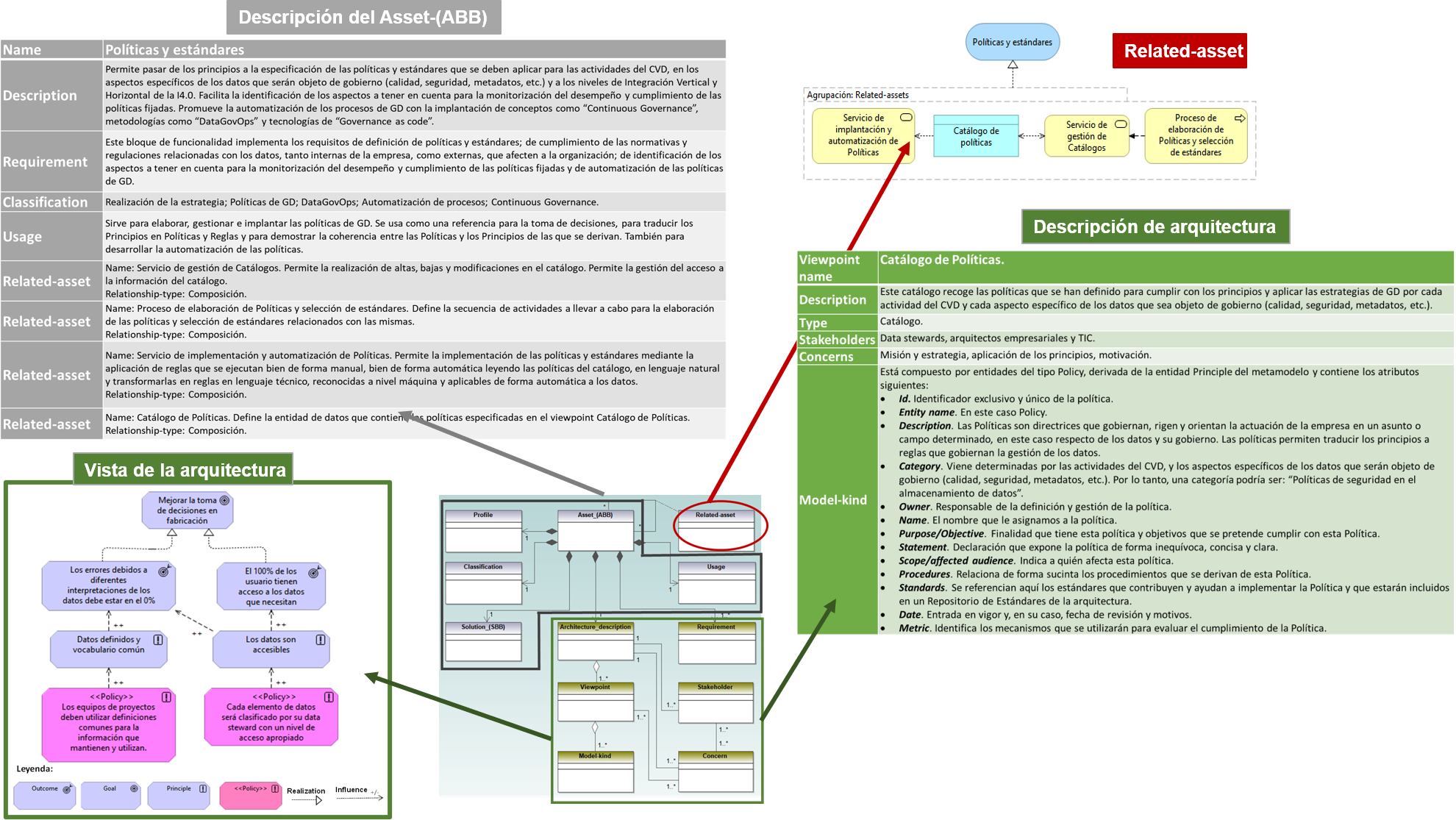