Mobiloscope: A Technological Solution for Early Mastitis Detection in Dairy Cattle
Keywords:
Mastitis detection, Articial Intelligence, Fluorescence microscopyAbstract
One of the most critical challenges in dairy farms is the Mastistis condition causing economic losses associated with milk production reduction and veterinary treatment expenses. Although it exists different methodologies for diagnosing animals with mastitis, these tests are usually indirect; others require laboratory analysis taking a lot of time to obtain the result, limiting its viability and monitoring in the field. To solve this problem, we propose a Mobiloscope, which is a portable, practical, effective, and low-cost diagnostic system for sub-clinical mastitis. Hence, this device provides an early detection in-situ and at a low cost to cover farmers' unsatisfied demand for having innovative tools that allow them to carry out better sub-clinical mastitis early detection. Our system comprises four components: (i) the holder for the electronic device and the screen to display the graphic interface; (ii) a part where the battery for the micro-computer will be housed; (iii) a dedicated part for the microscope and sample holder; and (iv) a holder for the light source. Despite the need to validate the prototype for commercial purposes, our prototype is able to estimate the number of somatic cells. Therefore, our mobiloscope could help the farmers to make an in-situ analysis of milk quality at a low-cost.
Downloads
References
O. Fernández Bolañoos, J. Trujillo Graffe, J. Peña Cabrera, J. Cerquera Gallego, and Y. Granja Salcedo, "Mastitis bovina: generalidades y métodos de diagnóstico,". Revista electrónica de Veterinaria, vol. 13, no. 11, pp. 1–20, 2012.
C. Sanford, G. P. Keefe, J. Sanchez, R. Dingwell, H. Barkema, K. Leslie, and I. R. Dohoo, "Test characteristics from latent-class models of the california mastitis test," Preventive Veterinary Medicine, vol. 77, no. 1-2, pp. 96–108, 2006.
J. Cerqueira, A. Cruz, J. Correia, I. Blanco-Penedo, J. Cantalapiedra, and J. Araujo, "Test de california para mastitis y microbiología de la glandula mamaria en explotaciones de vacas lecheras," 2017. ´
P. L. Ruegg, "Investigation of mastitis problems on farms," Veterinary Clinics: Food Animal Practice, vol. 19, no. 1, pp. 47–73, 2003.
O. E. Gomez-Quispe, C. S. Santivañez-Ballón, F. Arauco-Villar, O. H. Espezua-Flores, and J. Manrique-Meza, "Criterios de interpretación para california mastitis test en el diagnostico de mastitis subclínica en bovinos," Revista de Investigaciones Veterinarias del Peru´, vol. 26, no. 1, pp. 86–95, 2015.
R. Sinha, M. Bhakat, T. Mohanty, A. Ranjan, R. Kumar, S. A. Lone, A. Rahim, A. R. Paray, K. Khosla, and Z. Danish, "Infrared thermography as non-invasive technique for early detection of mastitis in dairy animals-a review," Asian Journal of Dairy & Food Research, vol. 37, no. 1, 2018.
F. Aguilar Galvez and C. A. Álvarez Díaz, "Mastitis bovina," 2019.
C. Bedolla, V. Castaneda, and W. Wolter, "Métodos de detección de la mastitis bovina (methods of detection of the bovine mastitis)," Redvet, 2007.
M. Perez-Ruano and L. Tarafa-Zambrana, "Evaluación del equipo mas-d-tec en el diagnóstico de campo de mastitis subclínica en el ganado bovino," Revista de Salud Animal, vol. 39, no. 3, pp. 00–00, 2017.
D. Brezovan, J. Savici, R. Moga, M. S, incai et al., "Investigations regarding the value of some direct and indirect diagnose tests for cattle subclinical mastitis," Lucrari Stiintifice-Universitatea de Stiinte Agricole a Banatului Timisoara, Medicina Veterinara, vol. 46, no. 3, pp. 20–26, 2013.
W. Law and A. Finlay, "Portascc®, new portable quantitative scc test for mastitis," in American Association of Bovine Practitioners Proceedings of the Annual Conference, 2006, pp. 302–302.
H. Langoni, D. d. S. Penachio, D. B. Nobrega, F. d. F. Guimaráes, and S. B. Lucheis, "Somaticell® as a screening method for somatic cell count from bovine milk," Ciencia Rural, vol. 42, no. 6, pp. 1095–1101, 2012.
K. Kawai, T. Hayashi, Y. Kiku, T. Chiba, H. Nagahata, H. Higuchi, T. Obayashi, S. Itoh, K. Onda, S. Arai et al., "Reliability in somatic cell count measurement of clinical mastitis milk using delaval cell counter," Animal Science Journal, vol. 84, no. 12, pp. 805–807, 2013.
E. F. Arcuri, P. D. L. d. Silva, J. R. F. Brito, M. R. Silva, and G. N. Souza, "Emprego do somacount 300, calibrado com leite de vaca, na contagem de celulas somáticas no leite de cabra," Ciencia Rural, vol. 34, no. 5, pp. 1497–1500, 2004.
S. M. R. Sumon, M. S. Parvin, M. A. Ehsan, and M. T. Islam, "Dynamics of somatic cell count and intramammary infection in lactating dairy cows," Journal of Advanced Veterinary and Animal Research, vol. 7, no. 2, p. 314, 2020.
Y. Zeng, K. Jin, J. Li, J. Liu, J. Li, T. Li, and S. Li, "A low cost and portable smartphone microscopic device for cell counting," Sensors and Actuators A: Physical, vol. 274, pp. 57–63, 2018.
F. Gao, J. Wang, Y. Ge, and S. Lu, "A vision-based instrument for measuring milk somatic cell count," Measurement Science and Technology, vol. 31, no. 12, p. 125904, 2020.
N. Otsu, "A threshold selection method from gray-level histograms," IEEE transactions on systems, man, and cybernetics, vol. 9, no. 1, pp. 62–66, 1979.
P. L. Rosin, "Unimodal thresholding," Pattern recognition, vol. 34, no. 11, pp. 2083–2096, 2001.
G. Grillo, M. Perez, J. Baro, and C. Carleos, "Video-microscopy as an alternative method for evaluation of somatic cell count," in 2005 IEEE Instrumentationand Measurement Technology Conference Proceedings, vol. 1. IEEE, 2005, pp. 236–239.
X. Gao, H. Xue, X. Pan, X. Jiang, Y. Bo, and Y. Wang, "Segmentation of somatic cells based on cloud model," Rev. Tec. Ing. Univ. Zulia, vol. 39, no. 2, pp. 93–101, 2016.
G. de Melo, V. Gomes, C. Baccili, L. de Almeida, and A. d. C. Lima, "A robust segmentation method for counting bovine milk somatic cells in microscope slide images," Computers and Electronics in Agriculture, vol. 115, pp. 142–149, 2015.
F. Wang and X. Pan, "Image segmentation for somatic cell of milk based on niching particle swarm optimization otsu," Engineering in Agriculture, Environment and Food, vol. 12, no. 2, pp. 141–149, 2019.
A. S. Ramos, C. H. Fontes, A. M. Ferreira, C. C. Baccili, K. N. da Silva, V. Gomes, and G. J. A. de Melo, "Somatic cell count in buffalo milk using fuzzy clustering and image processing techniques," Journal of Dairy Research, pp. 1–4, 2021.
A. Mavrogenis, A. Koumas, C. Kakoyiannis, and C. Taliotis, "Use of somatic cell counts for the detection of subclinical mastitis in sheep," Small Ruminant Research, vol. 17, no. 1, pp. 79–84, 1995.
Q. Dai and Y. Yu, "The advances of mathematical morphology in image processing," Control Theory and Application, vol. 18, no. 4, pp. 479–481, 2001.
J. MacQueen et al., "Some methods for classification and analysis of multivariate observations," in Proceedings of the fifth Berkeley symposium on mathematical statistics and probability, vol. 1, no. 14. Oakland, CA, USA, 1967, pp. 281–297.
J. Wu, Advances in K-means clustering: a data mining thinking," Springer Science & Business Media, 2012.
R. C. Gonzalez and R. E. Woods, "Digital image processing, 2002" Google Scholar Google Scholar Digital Library Digital Library, 2007.
J. Rogowska, "Overview and fundamentals of medical image segmentation," in Handbook of Medical Imaging, ser. Biomedical Engineering, I. N. BANKMAN, Ed. San Diego: Academic Press, 2000, pp. 69–85. [Online]. Available: https://www.sciencedirect.com/science/article/pii/B9780120777907500096
A. Kumar and A. Tiwari, "A comparative study of otsu thresholding and k-means algorithm of image segmentation," Int. J. Eng. Technol. Res, vol. 9, pp. 2454–4698, 2019.
S. van der Walt, J. L. Schonberger, J. Nunez-Iglesias, F. Boulogne, J. D. Warner, N. Yager, E. Gouillart, T. Yu, and the scikit-image contributors, "scikit-image: image processing in Python," PeerJ, vol. 2, p. e453, 6 2014. [Online]. Available: https://doi.org/10.7717/peerj.453
S. C. Prescott and R. S. Breed, "The Determination of the Number of Body Cells in Milk by a Direct Method," The Journal of Infectious Diseases, vol. 7, no. 5, pp. 632–640, 10 1910. [Online]. Available: https://doi.org/10.1093/infdis/7.5.632
X. Gao, H. Xue, X. Pan, X. Jiang, Y. Zhou, and X. Luo, "Somatic cells recognition by application of gabor feature-based (2d) 2pca," International Journal of Pattern Recognition and Artificial Intelligence, vol. 31, no. 12, p. 1757009, 2017.
J. H. B. Ribeiro and R. F. Hashimoto, "Pattern recognition based on straight line segments," in Pattern Recognition Recent Advances. InTech, 2010.
R. Medina-Rodríguez, C. B. Castañon, and R. F. Hashimoto, "Evaluation of the impact of initial positions obtained by clustering algorithms on the straight line segments classifier," in 2018 IEEE Latin American Conference on Computational Intelligence (LA-CCI). IEEE, 2018, pp. 1–6.
T. Ojala, M. Pietikainen, and D. Harwood, "Performance evaluation of texture measures with classification based on kullback discrimination of distributions," in Proceedings of 12th international conference on pattern recognition, vol. 1. IEEE, 1994, pp. 582–585.
T. Ojala, M. Pietikainen, and D. Harwood, "A comparative study of texture measures with classification based on featured distributions," Pattern recognition, vol. 29, no. 1, pp. 51–59, 1996.
M. Pietikainen and G. Zhao, "Two decades of local binary patterns: A "survey" in Advances in independent component analysis and learning machines," Elsevier, 2015, pp. 175–210
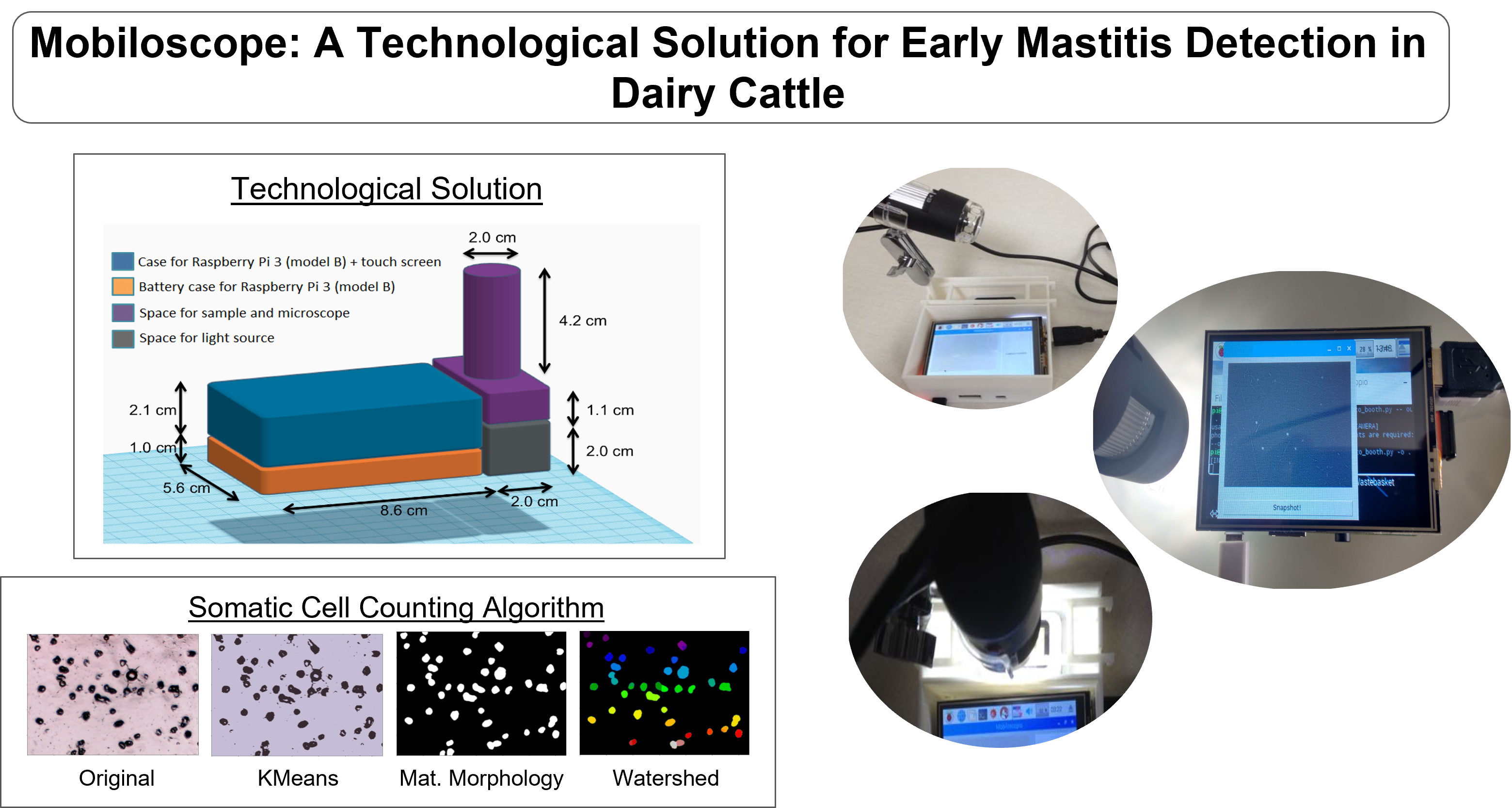