A Hybrid Evolutionary Approach Applied to the Economic Dispatch Problem with Prohibited Operating Zones and Uncertainties
Keywords:
Economic Dispatch Problem, evolutionary computation, robust optimizationAbstract
In order to be able to implement a solution to a real world problem, it must be solved taking into account the uncertainties in the associated model. In fact, there is a possibility that the action of a small uncertainty on a nominal solution obtained for a real world problem may become completely meaningless in practice. This paper presents a hybrid algorithm, HEA-GA-SA, to solve the Economic Dispatch Problem with Prohibited Operating Zones and Uncertainties. HEA-GA-SA considers the worst case of parametric uncertainties. The parameter in which the uncertainties have been considered is the power of generating units. The proposed algorithm proved to be effective to solve this problem. Despite having higher computational and dispatch costs than that of the nominal optimal solutions, the robust solutions found by HEA-GA-SA do not become unfeasible in the presence of uncertainties. Therefore, they can be implemented in the real world. The experiments showed that all nominal optimal solutions analyzed (including two in literature) became infeasible in the presence of uncertainties. They violated some of the constraints imposed on the problem, such as the Prohibited Operation Zones (POZs) or the power intervals in which each generator can operate. It was also observed that the presence of POZs makes the problem more difficult and vulnerable to uncertainty.
Downloads
References
E. S. Oliveira, “Metaheurísticas aplicadas ao problema do despacho econômico de energia elétrica,” Dissertação (Mestrado em Engenharia Elétrica) – Faculdade de Engenharia, Universidade Federal de Juiz de Fora, Juiz de Fora, Brasil, 2015. [Online]. Available: http://repositorio.ufjf.br:8080/jspui/handle/ufjf/246. Accessed on: Aug. 30, 2020.
D. S. Kirschen and G. Strbac, Fundamentals of power system economics. John Wiley & Sons, Inc. 2004.
S. O. Orero and M. R. Irving, “Economic dispatch of generators with prohibited operating zones: a genetic algorithm approach,” IEE Proceedings-Generation, Transmission and Distribution, vol. 143, no. 6, pp. 529-534, Nov. 1996.
C.-L Chiang, “Genetic-based algorithm for power economic load dispatch,” IET generation, transmission & distribution, vol. 1, no. 2, pp. 261-269, Mar. 2007.
R. N. Martins and M. H. S. Mendes, “Algoritmo genético para o problema do despacho econômico de energia elétrica com incertezas,” Anais do XLVIII SBPO (pp. 810-817), Simpósio Brasileiro de Pesquisa Operacional, Sep. 2016. [Online]. Available: http://www.din.uem.br/sbpo/sbpo2016/pdf/155992.pdf. Accessed on: May. 15, 2020.
J. N. R. Assis, T. M. Machado-Coelho, G. L. Soares and M. H. S. Mendes, “Robust evolutionary optimization algorithm for multi-objective environmental/economic dispatch problem with uncertainties,” IEEE Congress on Evolutionary Computation, IEEE World Congress on Computational Intelligence (IEEE WCCI), Jul. 2018. [Online]. Available: http://www.novoscursos.ufv.br/graduacao/caf/ccp/www/wp-content/uploads/2018/03/JoseNunes.pdf. Accessed on: May. 15, 2020.
M. R. B. D. Santos, A. R. Balbo, E. Gonçalves, E. M. Soler, R. B. N. M. Pinheiro, L. Nepomuceno and E. C. Baptista, “A proposed methodology involving progressive bounded constraints and interior-exterior methods in smoothed economic/environmental dispatch problems,” IEEE Latin America Transactions, vol. 15, no. 8, pp. 1422-1431, Jan. 2017. [Online]. Available: http://hdl.handle.net/11449/180467. Accessed on: May. 15, 2020. [8] S. Pan, J. Jian, H. Chen and L. Yang, “A full mixed-integer linear programming formulation for economic dispatch with valve-point effects, transmission loss and prohibited operating zones,” Electric Power Systems Research, vol. 180, p. 106061, Mar. 2020. [Online]. Available: https://www.researchgate.net/publication/337881980_A_full_mixed-integer_linear_programming_formulation_for_economic_dispatch_with_valve-point_effects_transmission_loss_and_prohibited_operating_zones. Accessed on: May. 15, 2020. [9] A. Derghal and N. Golea, “Genetic algorithm for solving large practical fuzzy economic load dispatch with prohibited operating zones,” IEEE International Renewable and Sustainable Energy Conference (IRSEC), pp. 469-474, Ouarzazate, Oct. 2014. [Online]. Available: http://www.univ-oeb.dz/bibliotheque/wp-content/uploads/2016/11/Genetic-Algorithm.pdf. Accessed on: May. 15, 2020. [10] C. L. Sabioni, “Desenvolvimento de Métodos para Solução de Problemas de Otimização Multiobjetivo com Incertezas,” Tese (Doutorado em Engenharia Elétrica) – Escola de Engenharia, Universidade Federal de Minas Gerais, Minas Gerais, Brasil, 2017. [Online]. Available: https://www.ppgee.ufmg.br/defesas/1077D.PDF. Accessed on: May. 15, 2020.
M. H. S. Mendes, “Algoritmos evolucionários intervalares para otimização robusta multiobjetivo,” Tese (Doutorado em Engenharia Elétrica) – Universidade Federal de Minas Gerais, Minas Gerais, Brasil, 2013. [Online]. Available: https://repositorio.ufmg.br/handle/1843/BUBD-9P8GFZ. Accessed on: May. 15, 2020.
A. Ben-tal, L. E. Ghaoui and A. Nemirovski, Robust Optimization. Princeton University Press, 2009. [Online]. Available: https://www2.isye.gatech.edu/~nemirovs/FullBookDec11.pdf. Accessed on: May. 15, 2020.
K. Deb and R. B. Agrawal, "Simulated Binary Crossover for Continuous Search Space," Complex Systems, vol. 9, no. 2, pp. 115-148, 1995. [Online]. Available: https://pdfs.semanticscholar.org/b8ee/6b68520ae0291075cb1408046a7dff9dd9ad.pdf. Accessed on: Sep. 01, 2020.
K. Deb and A. Kumar, "Real-coded Genetic Algorithms with Simulated Binary Crossover: Studies on Multimodal and Multiobjective Problems," Complex Systems, vol. 9, pp. 431-454, 1995. [Online]. Available: https://pdfs.semanticscholar.org/e1ea/72f285688e55d6cd38e8701c50f8e28f0262.pdf. Accessed on: Sep. 01, 2020.
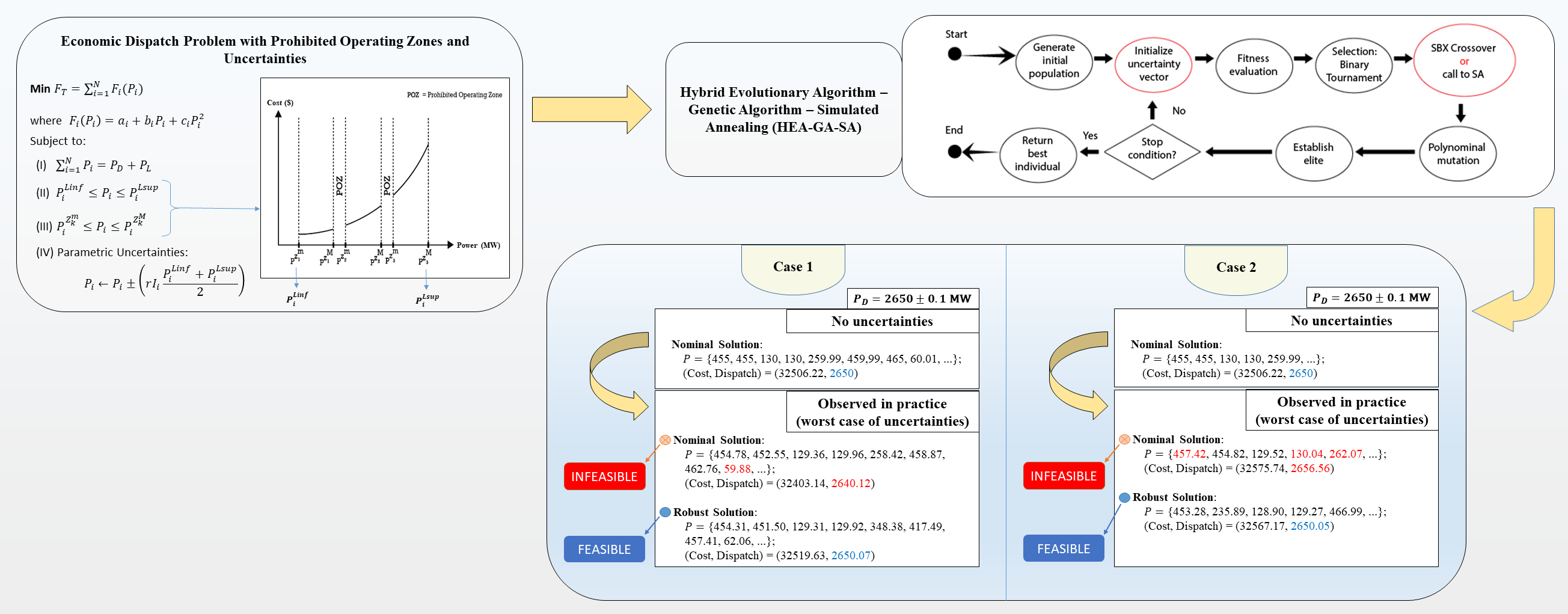