Empirical Exploration of Machine Learning Techniques for Detection of Anomalies Based on NIDS
Keywords:
Machine Learning, nidsAbstract
Computer crimes and attacks on data networks have increased significantly, so it has become necessary to implement techniques that detect these threats and safeguard the information of organizations. Network Intrusion Detection Systems (NIDS) allow detecting anomalies and attacks in real time, by analyzing the local and outgoing traffic of the network. At present, to improve its performance, it has been chosen to use Machine Learning (ML) techniques that automate these processes and improve the detection of an anomaly. This paper implements ML techniques through the use of datasets, in the context of a NIDS, for the detection and prediction of anomalies on networks. Tests were performed with non-supervised and supervised learning algorithms on NSL-KDD and UNSW-NB15 datasets. An exploratory analysis of data together with dimensionality reduction techniques allowed us to understand the nature of the data, prior to the modeling. The results show that the methodology can be extrapolated for real scenarios with different network configurations.
Downloads
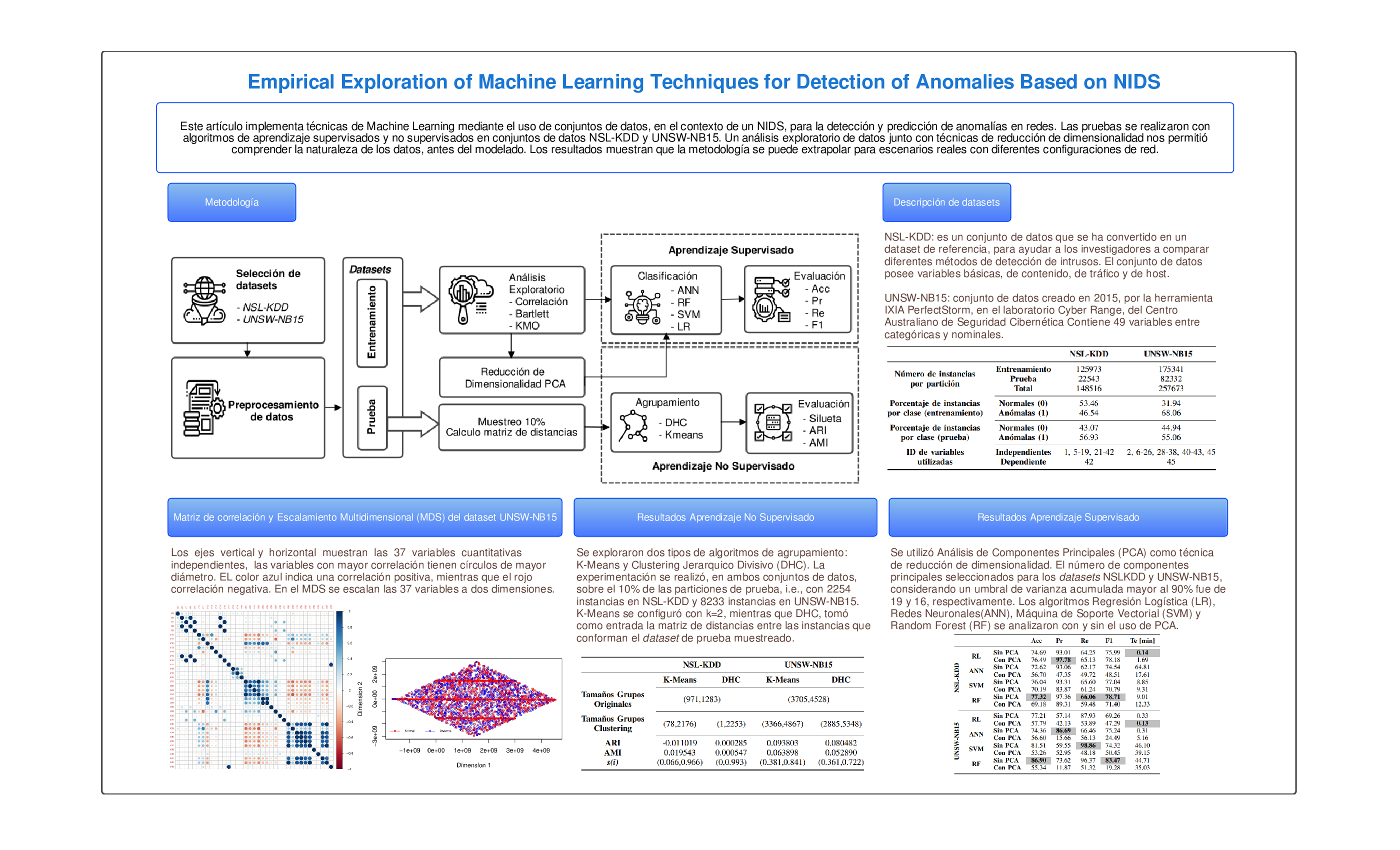