Evaluation of Technical Analysis Trading Rules in a Artificial Stock Market Environment
Keywords:
Multi-agent System, Artificial Stock Market, Heterogeneous Agents, Performance Technical Trading RulesAbstract
Artificial multi-agent systems can be applied to replicate complex processes, including the stock market. In this manuscript, an artificial stock market was simulated. Heterogeneous agents are set to trade by using different investiment strategies through a double auction market. The main innovative aspect of this work is to evaluate the performance and effectiveness of agents with different investment strategies based on the combination of Technical/Fundamental Analysis. According to the simulations performed, the results showed that the time series resulting from the interactions among these agents resemble to the real financial series, reproducing some of the stylized facts found in the Literature. Moreover, the statistical analysis reported no significant differences in the distribution mean performance between these diverse agents types
Downloads
References
Z. Eisler and J. Kertesz, “Size matters: some stylized facts of the stock market revisited,” The European Physical Journal B-Condensed Matter and Complex Systems, vol. 51, no. 1, pp. 145–154, 2006.
R. T. F. Nazário, J. L. e Silva, V. A. Sobreiro, and H. Kimura, “A literature review of technical analysis on stock markets,” The Quarterly Review of Economics and Finance, vol. 66, pp. 115–126, 2017.
J. J. Murphy, Technical Analysis of the Financial Markets: A Comprehensive Guide to Trading Methods and Applications. New York Institute of Finance, 1999.
G. Scott, M. Carr, and M. Cremonie, Technical Analysis: Modern Perspectives, ser. CFA Institute Research Foundation Literature Reviews. CFA Institute Research Foundation, 2016.
R. W. Colby, The Encyclopedia of Technical Market Indicators, 2nd ed. McGraw-Hill, 2003.
R. C. Cavalcante, R. C. Brasileiro, V. L. Souza, J. P. Nobrega, and A. L. Oliveira, “Computational intelligence and financial markets: A survey and future directions,” Expert Systems with Applications, vol. 55, pp. 194–211, 2016.
E. F. Sbruzzi, M. C. R. Leles, and C. L. Nascimento Júnior, “Introducing learning automata to financial portfolio components selection,” in 2018 Annual IEEE International Systems Conference (SysCon), 2018, pp. 1–6.
M. C. R. Leles, L. A. Mozelli, E. F. Sbruzzi, C. L. Nascimento Júnior, and H. N. Guimarães, “A multicriteria trading system based on singular spectrum analysis trading rules,” IEEE Systems Journal, 2019.
M. C. R. Leles, L. A. Mozelli, C. L. Nascimento Júnior, E. F. Sbruzzi, and H. N. Guimarães, “Study on Singular Spectrum Analysis as a new technical oscillator for trading rules design,” Fluctuations and Noise Letters, vol. 17, no. 4, p. 1850034, 2018.
M. C. R. Leles, L. A. Mozelli, and H. N. Guimarães, “New trendfollowing indicator: Using SSA to design trading rules,” Fluctuation and Noise Letters, vol. 16, no. 2, p. 1750016, 2017.
W. Brock, J. Lakonishok, and B. LeBaron, “Simple technical trading rules and the stochastic properties of stock returns,” Journal of Finance, vol. 47, no. 5, pp. 1731–1764, 1992.
N. Taylor, “The rise and fall of technical trading rule success,” Journal of Banking & Finance, vol. 40, pp. 286 – 302, 2014.
Y. M. Rekik, W. Hachicha, and Y. Boujelbene, “Agent-based Modeling and Investors’ Behavior Explanation of Asset Price Dynamics on Artificial Financial Markets,” Procedia Economics and Finance, vol. 13, pp. 30 – 46, 2014, first Annual Tunisian Society for Financial Studies (TSFS) Finance Conference 2013.
J. D. Farmer and D. Foley, “The economy needs agent-based modelling,” Nature, vol. 460, no. 7256, p. 685, 2009.
J.-P. Bouchaud, “Economics needs a scientific revolution,” Nature, vol. 455, no. 7217, p. 1181, 2008.
M. Friedman and L. J. Savage, “The utility analysis of choices involving risk,” Journal of Political Economy, vol. 56, no. 4, pp. 279–304, 1948.
E. F. Fama, “Efficient capital markets: A review of theory and empirical work,” The Journal of Finance, vol. 25, no. 2, pp. 383–417, 1970.
A. Chakraborti, I. M. Toke, M. Patriarca, and F. Abergel, “Econophysics review: Ii. agent-based models,” Quantitative Finance, vol. 11, no. 7, pp. 1013–1041, 2011.
T. Lux and M. Marchesi, “Volatility clustering in financial markets: a microsimulation of interacting agents,” International Journal of Theoretical and Applied Finance, vol. 3, no. 04, pp. 675–702, 2000.
C. Chiarella and G. Iori, “A simulation analysis of the microstructure of double auction markets,” Quantitative Finance, vol. 2, no. 5, pp. 346–353, 2002.
M. C. R. Leles, E. F. Sbruzzi, C. L. Nascimento Júnior, and J. M. P. Oliveira, “Trading Switching Setup Based on Reinforcement Learning Applied to a Multiagent System Simulation of Financial Markets,” in 2019 Annual IEEE International Systems Conference. IEEE, 2019, pp. 1–8.
M. C. R. Leles, E. F. Sbruzzi, C. L. Nascimento, and J. M. P. Oliveira, “A MatLab Computational Framework for Multiagent System Simulation of Financial Markets,” in 2019 IEEE International Systems Conference (SysCon). IEEE, 2019, pp. 1–8.
E. F. Fama, “Efficient capital markets: II,” The Journal of Finance, vol. 46, no. 5, pp. 1575–1617, 1991.
A. W. Lo, “Adaptive markets hypothesis: Market efficiency from an evolutionary perspective,” Journal of Portfolio Management, vol. 30, pp. 15–29, 2004.
K. Raya, C. G. Gaxiola, and M. Castanon, “Agent-based model for self management of network flows using negotiation,” IEEE Latin America Transactions, vol. 16, no. 1, pp. 210–215, 2018.
U. Wilensky and W. Rand, An introduction to Agent-Based Modeling: Modeling Natural, Social, and Engineered Complex Systems with NetLogo. MIT Press, 2015.
S. Abar, G. K. Theodoropoulos, P. Lemarinier, and G. M. O’Hare, “Agent based modelling and simulation tools: A review of the stateof-art software,” Computer Science Review, vol. 24, pp. 13–33, 2017.
S. Maslov, “Simple model of a limit order-driven market,” Physica A: Statistical Mechanics and its Applications, vol. 278, no. 3-4, pp. 571– 578, 2000.
A. W. Lo, H. Mamaysky, and J. Wang, “Foundations of technical analysis: Computational algorithms, statistical inference, and empirical implementation,” The Journal of Finance, vol. 55, no. 4, pp. 1705–1765, 2000.
R. Cont, “Empirical properties of asset returns: stylized facts and statistical issues,” Quantitative Finance, vol. 1, no. 2, pp. 223–236, 2001.
M. Metghalchi, L. A. Hayes, and F. Niroomand, “A technical approach to equity investing in emerging markets,” Review of Financial Economics, vol. 37, no. 3, pp. 389–403, 2019.
Y. Deng, F. Bao, Y. Kong, Z. Ren, and Q. Dai, “Deep direct reinforcement learning for financial signal representation and trading,” IEEE Transactions on Neural Networks and Learning Systems, vol. 28, no. 3, pp. 653–664, 2017.
G. Yang, Y. Chen, and J. Huang, “The highly intelligent virtual agents for modeling financial markets,” Physica A: Statistical Mechanics and its Applications, vol. 443, pp. 98 – 108, 2016.
K. Yim, G. Oh, and S. Kim, “Understanding financial market states using an artificial double auction market,” PloS One, vol. 11, no. 3, p. e0152608, 2016.
R. F. Engle, “Autoregressive conditional heteroscedasticity with estimates of the variance of united kingdom inflation,” Econometrica, vol. 50, no. 4, pp. 987–1007, 1982.
R. W. Banz, “The relationship between return and market value of common stocks,” Journal of Financial Economics, vol. 9, no. 1, pp. 3–18, 1981.
W. Easterly and R. Levine, “What have we learned from a decade of empirical research on growth? it’s not factor accumulation: Stylized facts and growth models,” The World Bank Economic Review, vol. 15, no. 2, pp. 177–219, 2001.
E. F. Fama, “Market efficiency, long-term returns, and behavioral finance,” Journal of Financial Economics, vol. 49, no. 3, pp. 283–306, 1998.
B. Yandell, Practical Data Analysis for Designed Experiments, ser. Chapman & Hall/CRC Texts in Statistical Science. Taylor & Francis, 1997.
D. Freedman, R. Pisani, and R. Purves, “Statistics (international student edition),” Pisani, R. Purves, 4th edn. WW Norton & Company, New York, 2007.
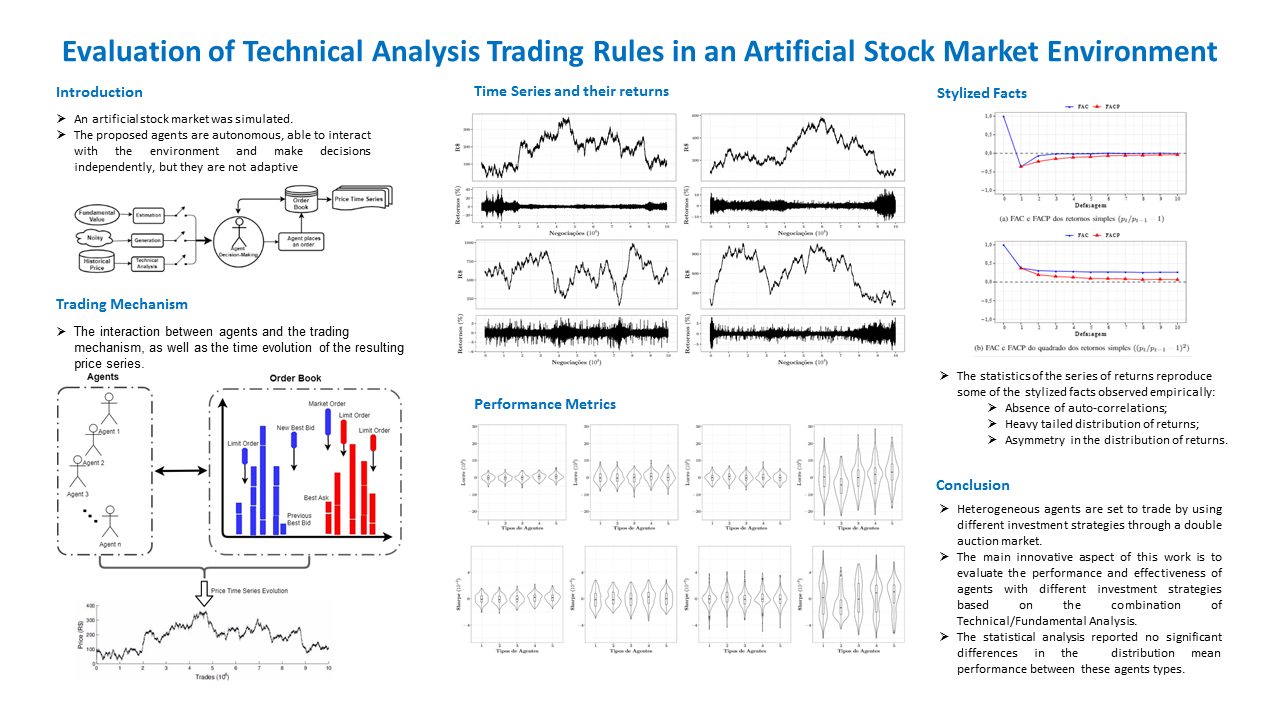